How to find the MLE of these parameters given distribution?Minimally Sufficient Statistic for Bivariate DistributionAssuming $sigma$ is known, find a method of moments estimator of $mu$. (Location-scale family of exponential distribution)finding the maximum likelihood estimator of location scale family of exponential distributionsComputation of a likelihood for a discrete variableDistribution of minimum of 2 INID random variables when one is transformed.Find joint density function of X and X+Y (exponential distribution)Maximum a Posteriori estimation of the parameter of a exponential distribution with a gaussian priorfind joint distribution $min (X,Y)$ and $W$, where W is a discrete distributionWhy are cross expectations zero in MLE?how fitting a Gaussian approximation to the likelihood curve at maximum?
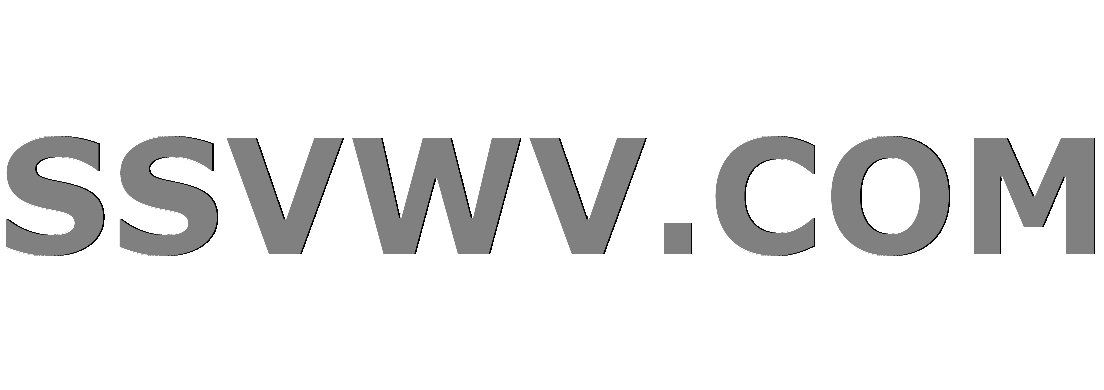
Multi tool use
ssTTsSTtRrriinInnnnNNNIiinngg
Avoiding the "not like other girls" trope?
Venezuelan girlfriend wants to travel the USA to be with me. What is the process?
How to compactly explain secondary and tertiary characters without resorting to stereotypes?
Is there a hemisphere-neutral way of specifying a season?
Why would the Red Woman birth a shadow if she worshipped the Lord of the Light?
How do I deal with an unproductive colleague in a small company?
Watching something be piped to a file live with tail
Do UK voters know if their MP will be the Speaker of the House?
What does the expression "A Mann!" means
What killed these X2 caps?
How to prevent "they're falling in love" trope
Why didn't Miles's spider sense work before?
Ambiguity in the definition of entropy
How can I determine if the org that I'm currently connected to is a scratch org?
Do scales need to be in alphabetical order?
How would I stat a creature to be immune to everything but the Magic Missile spell? (just for fun)
GFCI outlets - can they be repaired? Are they really needed at the end of a circuit?
How badly should I try to prevent a user from XSSing themselves?
Detention in 1997
Cursor Replacement for Newbies
How can I deal with my CEO asking me to hire someone with a higher salary than me, a co-founder?
How to show a landlord what we have in savings?
Should I tell management that I intend to leave due to bad software development practices?
How to find the MLE of these parameters given distribution?
Minimally Sufficient Statistic for Bivariate DistributionAssuming $sigma$ is known, find a method of moments estimator of $mu$. (Location-scale family of exponential distribution)finding the maximum likelihood estimator of location scale family of exponential distributionsComputation of a likelihood for a discrete variableDistribution of minimum of 2 INID random variables when one is transformed.Find joint density function of X and X+Y (exponential distribution)Maximum a Posteriori estimation of the parameter of a exponential distribution with a gaussian priorfind joint distribution $min (X,Y)$ and $W$, where W is a discrete distributionWhy are cross expectations zero in MLE?how fitting a Gaussian approximation to the likelihood curve at maximum?
$begingroup$
Let $X$ and $Y$ be independent exponential random variables, with
$$f(xmidlambda)=frac1lambdaexpleft(-fracxlambdaright),,x>0,, qquad f(ymidmu)=frac1muexpleft(-fracymuright),,y>0$$
We observe $Z$ and $W$ with $Z=min(X,Y)$, and $W=begincases 1 &,textif Z=X\ 0 &,textif Z=Y endcases$
I have obtained the joint distribution of $Z$ and $W$, i.e.,
$$P(Z leq z, W=0)=fraclambdamu+lambdaleft[1-expleft(-left(frac1mu+frac1lambdaright)zright)right]$$
$$P(Z leq z, W=1)=fracmumu+lambdaleft[1-expleft(-left(frac1mu+frac1lambdaright)zright)right]$$
Now assume that $(Z_i,W_i),i=1,cdots,n$, are $n$ i.i.d observations. Find the MLEs of $lambda$ and $mu$.
(This is the exercise 7.14 of the book Statistical Inference 2nd edition, but no solution given)
statistics maximum-likelihood exponential-distribution censoring
$endgroup$
add a comment |
$begingroup$
Let $X$ and $Y$ be independent exponential random variables, with
$$f(xmidlambda)=frac1lambdaexpleft(-fracxlambdaright),,x>0,, qquad f(ymidmu)=frac1muexpleft(-fracymuright),,y>0$$
We observe $Z$ and $W$ with $Z=min(X,Y)$, and $W=begincases 1 &,textif Z=X\ 0 &,textif Z=Y endcases$
I have obtained the joint distribution of $Z$ and $W$, i.e.,
$$P(Z leq z, W=0)=fraclambdamu+lambdaleft[1-expleft(-left(frac1mu+frac1lambdaright)zright)right]$$
$$P(Z leq z, W=1)=fracmumu+lambdaleft[1-expleft(-left(frac1mu+frac1lambdaright)zright)right]$$
Now assume that $(Z_i,W_i),i=1,cdots,n$, are $n$ i.i.d observations. Find the MLEs of $lambda$ and $mu$.
(This is the exercise 7.14 of the book Statistical Inference 2nd edition, but no solution given)
statistics maximum-likelihood exponential-distribution censoring
$endgroup$
add a comment |
$begingroup$
Let $X$ and $Y$ be independent exponential random variables, with
$$f(xmidlambda)=frac1lambdaexpleft(-fracxlambdaright),,x>0,, qquad f(ymidmu)=frac1muexpleft(-fracymuright),,y>0$$
We observe $Z$ and $W$ with $Z=min(X,Y)$, and $W=begincases 1 &,textif Z=X\ 0 &,textif Z=Y endcases$
I have obtained the joint distribution of $Z$ and $W$, i.e.,
$$P(Z leq z, W=0)=fraclambdamu+lambdaleft[1-expleft(-left(frac1mu+frac1lambdaright)zright)right]$$
$$P(Z leq z, W=1)=fracmumu+lambdaleft[1-expleft(-left(frac1mu+frac1lambdaright)zright)right]$$
Now assume that $(Z_i,W_i),i=1,cdots,n$, are $n$ i.i.d observations. Find the MLEs of $lambda$ and $mu$.
(This is the exercise 7.14 of the book Statistical Inference 2nd edition, but no solution given)
statistics maximum-likelihood exponential-distribution censoring
$endgroup$
Let $X$ and $Y$ be independent exponential random variables, with
$$f(xmidlambda)=frac1lambdaexpleft(-fracxlambdaright),,x>0,, qquad f(ymidmu)=frac1muexpleft(-fracymuright),,y>0$$
We observe $Z$ and $W$ with $Z=min(X,Y)$, and $W=begincases 1 &,textif Z=X\ 0 &,textif Z=Y endcases$
I have obtained the joint distribution of $Z$ and $W$, i.e.,
$$P(Z leq z, W=0)=fraclambdamu+lambdaleft[1-expleft(-left(frac1mu+frac1lambdaright)zright)right]$$
$$P(Z leq z, W=1)=fracmumu+lambdaleft[1-expleft(-left(frac1mu+frac1lambdaright)zright)right]$$
Now assume that $(Z_i,W_i),i=1,cdots,n$, are $n$ i.i.d observations. Find the MLEs of $lambda$ and $mu$.
(This is the exercise 7.14 of the book Statistical Inference 2nd edition, but no solution given)
statistics maximum-likelihood exponential-distribution censoring
statistics maximum-likelihood exponential-distribution censoring
edited Mar 21 at 10:03


StubbornAtom
6,29831440
6,29831440
asked Mar 21 at 7:16
Tim XuTim Xu
63
63
add a comment |
add a comment |
2 Answers
2
active
oldest
votes
$begingroup$
Note that $Z$ and $W$ are in fact independent.
For $z>0,,,win0,1$, you can write the joint distribution of $(Z,W)$ as
$$P(z,w)=frac1lambda^w mu^1-wexpleft[-left(frac1lambda+frac1muright)zright]$$
The above formulation is not completely rigorous as it is not a probability (I am searching for a better notation avoiding the dirac delta).
The likelihood function given the sample $(z_1,w_1),ldots,(z_n,w_n)$ is then
$$L(lambda,mu)=frac1lambda^sum_i=1^n w_imu^n-sum_i=1^n w_iexpleft[-left(frac1lambda+frac1muright)sum_i=1^n z_iright]$$
Log-likelihood is
$$ell(lambda,mu)=-sum_i=1^n w_ilnlambda-left(n-sum_i=1^n w_iright)lnmu--left(frac1lambda+frac1muright)sum_i=1^n z_i$$
For $0<bar w<1$, solving for the stationary points of $ell(lambda,mu)$ yields $$hatlambda=fracsum_i=1^n z_isum_i=1^n w_i=fracbar zbar wqquad,qquad hatmu=fracsum_i=1^n z_in-sum_i=1^n w_i=fracbar z1-bar w$$
So assuming $0<bar w<1$, the unique MLE of $(lambda,mu)$ is $(hatlambda,hatmu)$.
But when $bar win0,1$, the MLE does not exist.
$endgroup$
$begingroup$
I still have two questions. (Q1) How to solve for the stationary points of $ell(lambda,mu)$? (Q2) Why do we need to discuss the value range of $barw$? (In addition) Shouldn't the $mu$ in your Log-likelihood is $lnmu$?
$endgroup$
– Tim Xu
Mar 21 at 12:08
$begingroup$
You are right about the log-likelihood. I will edit.
$endgroup$
– StubbornAtom
Mar 21 at 12:18
$begingroup$
@TimXu I just differentiated $ell$ once wrt $lambda$ and $mu$ and set them equal to zero (for multiple parameters, one should ideally check whether the Hessian matrix of the log-likelihood is negative definite at $(hatlambda,hatmu)$, provided the derivatives exist). And you can see from the expression for $hatlambda,hatmu$ that they are not defined at $bar w=0,1$. Hence the range distinction.
$endgroup$
– StubbornAtom
Mar 21 at 12:35
add a comment |
$begingroup$
The $W=0$ and the $W=1$ case can be combined by writing $lambda^1-Wmu^W$.
First differentiating w.r.t. $z$ and forming the product the likelihood can be written as $prod_i^nfrac1lambda^w_imu^1-w_ie^-(frac1lambda+frac1mu)z_i$.
Taking logs gives the log likelihood $=-sum_i^n(w_ilnlambda+(1-w_i)lnmu+(frac1lambda+frac1mu)z_i)$.
Maximising wrt $lambda$ and $mu$ gives $lambda=fracbarzbarw$ and $mu=fracbarz(1-barw)$
Note if $lambda=mu$ then $barwapproxfrac12$ so the estimates for $lambda$ and $mu$ become equal.
$endgroup$
$begingroup$
Denote the log likelihood by $log L$. First differentiating w.r.t. $lambda$ and $mu$, gives $hatlambda=fracbarzbarw$, $hatmu=fracbarz1-barw$. To make sure they are the maximizers of the log likelihood. I calculate their second-order differentials w.r.t. $lambda$ and $mu$, and get that $fracpartial^2 log Lpartial lambda^2=-sum_i=1^n(-fracw_ilambda^2+2fracz_ilambda^3)$ and $fracpartial^2 log Lpartial mu^2=-sum_i=1^n(-frac1-w_imu^2+2fracz_imu^3)$. How do you know these two second-order differentials are negative?
$endgroup$
– Tim Xu
Mar 21 at 11:44
$begingroup$
Direct substitution. for instance $fracpartial^2 logLpartial lambda^2=-fracbarw^3barz^2$
$endgroup$
– user121049
Mar 21 at 14:09
$begingroup$
You missed an $n$. It is $fracpartial ^2 logLpartial lambda^2 |_lambda=fracbar zbar w= -sum_i=1^n (-fracw_ilambda^2+2fracz_ilambda^3) |_lambda=fracbar zbar w= (fracsum_i=1^n w_ilambda^2-2fracsum_i=1^n z_ilambda^3) |_lambda=fracbar zbar w= (fracn bar wlambda^2-2fracn bar zlambda^3) |_lambda=fracbar zbar w= fracn bar w^3bar z^2-2fracn bar z bar w^3bar z^3= fracn bar w^3bar z^2-2fracn bar w^3bar z^2=-fracn bar w^3bar z^2$
$endgroup$
– Tim Xu
Mar 22 at 6:41
add a comment |
Your Answer
StackExchange.ifUsing("editor", function ()
return StackExchange.using("mathjaxEditing", function ()
StackExchange.MarkdownEditor.creationCallbacks.add(function (editor, postfix)
StackExchange.mathjaxEditing.prepareWmdForMathJax(editor, postfix, [["$", "$"], ["\\(","\\)"]]);
);
);
, "mathjax-editing");
StackExchange.ready(function()
var channelOptions =
tags: "".split(" "),
id: "69"
;
initTagRenderer("".split(" "), "".split(" "), channelOptions);
StackExchange.using("externalEditor", function()
// Have to fire editor after snippets, if snippets enabled
if (StackExchange.settings.snippets.snippetsEnabled)
StackExchange.using("snippets", function()
createEditor();
);
else
createEditor();
);
function createEditor()
StackExchange.prepareEditor(
heartbeatType: 'answer',
autoActivateHeartbeat: false,
convertImagesToLinks: true,
noModals: true,
showLowRepImageUploadWarning: true,
reputationToPostImages: 10,
bindNavPrevention: true,
postfix: "",
imageUploader:
brandingHtml: "Powered by u003ca class="icon-imgur-white" href="https://imgur.com/"u003eu003c/au003e",
contentPolicyHtml: "User contributions licensed under u003ca href="https://creativecommons.org/licenses/by-sa/3.0/"u003ecc by-sa 3.0 with attribution requiredu003c/au003e u003ca href="https://stackoverflow.com/legal/content-policy"u003e(content policy)u003c/au003e",
allowUrls: true
,
noCode: true, onDemand: true,
discardSelector: ".discard-answer"
,immediatelyShowMarkdownHelp:true
);
);
Sign up or log in
StackExchange.ready(function ()
StackExchange.helpers.onClickDraftSave('#login-link');
);
Sign up using Google
Sign up using Facebook
Sign up using Email and Password
Post as a guest
Required, but never shown
StackExchange.ready(
function ()
StackExchange.openid.initPostLogin('.new-post-login', 'https%3a%2f%2fmath.stackexchange.com%2fquestions%2f3156467%2fhow-to-find-the-mle-of-these-parameters-given-distribution%23new-answer', 'question_page');
);
Post as a guest
Required, but never shown
2 Answers
2
active
oldest
votes
2 Answers
2
active
oldest
votes
active
oldest
votes
active
oldest
votes
$begingroup$
Note that $Z$ and $W$ are in fact independent.
For $z>0,,,win0,1$, you can write the joint distribution of $(Z,W)$ as
$$P(z,w)=frac1lambda^w mu^1-wexpleft[-left(frac1lambda+frac1muright)zright]$$
The above formulation is not completely rigorous as it is not a probability (I am searching for a better notation avoiding the dirac delta).
The likelihood function given the sample $(z_1,w_1),ldots,(z_n,w_n)$ is then
$$L(lambda,mu)=frac1lambda^sum_i=1^n w_imu^n-sum_i=1^n w_iexpleft[-left(frac1lambda+frac1muright)sum_i=1^n z_iright]$$
Log-likelihood is
$$ell(lambda,mu)=-sum_i=1^n w_ilnlambda-left(n-sum_i=1^n w_iright)lnmu--left(frac1lambda+frac1muright)sum_i=1^n z_i$$
For $0<bar w<1$, solving for the stationary points of $ell(lambda,mu)$ yields $$hatlambda=fracsum_i=1^n z_isum_i=1^n w_i=fracbar zbar wqquad,qquad hatmu=fracsum_i=1^n z_in-sum_i=1^n w_i=fracbar z1-bar w$$
So assuming $0<bar w<1$, the unique MLE of $(lambda,mu)$ is $(hatlambda,hatmu)$.
But when $bar win0,1$, the MLE does not exist.
$endgroup$
$begingroup$
I still have two questions. (Q1) How to solve for the stationary points of $ell(lambda,mu)$? (Q2) Why do we need to discuss the value range of $barw$? (In addition) Shouldn't the $mu$ in your Log-likelihood is $lnmu$?
$endgroup$
– Tim Xu
Mar 21 at 12:08
$begingroup$
You are right about the log-likelihood. I will edit.
$endgroup$
– StubbornAtom
Mar 21 at 12:18
$begingroup$
@TimXu I just differentiated $ell$ once wrt $lambda$ and $mu$ and set them equal to zero (for multiple parameters, one should ideally check whether the Hessian matrix of the log-likelihood is negative definite at $(hatlambda,hatmu)$, provided the derivatives exist). And you can see from the expression for $hatlambda,hatmu$ that they are not defined at $bar w=0,1$. Hence the range distinction.
$endgroup$
– StubbornAtom
Mar 21 at 12:35
add a comment |
$begingroup$
Note that $Z$ and $W$ are in fact independent.
For $z>0,,,win0,1$, you can write the joint distribution of $(Z,W)$ as
$$P(z,w)=frac1lambda^w mu^1-wexpleft[-left(frac1lambda+frac1muright)zright]$$
The above formulation is not completely rigorous as it is not a probability (I am searching for a better notation avoiding the dirac delta).
The likelihood function given the sample $(z_1,w_1),ldots,(z_n,w_n)$ is then
$$L(lambda,mu)=frac1lambda^sum_i=1^n w_imu^n-sum_i=1^n w_iexpleft[-left(frac1lambda+frac1muright)sum_i=1^n z_iright]$$
Log-likelihood is
$$ell(lambda,mu)=-sum_i=1^n w_ilnlambda-left(n-sum_i=1^n w_iright)lnmu--left(frac1lambda+frac1muright)sum_i=1^n z_i$$
For $0<bar w<1$, solving for the stationary points of $ell(lambda,mu)$ yields $$hatlambda=fracsum_i=1^n z_isum_i=1^n w_i=fracbar zbar wqquad,qquad hatmu=fracsum_i=1^n z_in-sum_i=1^n w_i=fracbar z1-bar w$$
So assuming $0<bar w<1$, the unique MLE of $(lambda,mu)$ is $(hatlambda,hatmu)$.
But when $bar win0,1$, the MLE does not exist.
$endgroup$
$begingroup$
I still have two questions. (Q1) How to solve for the stationary points of $ell(lambda,mu)$? (Q2) Why do we need to discuss the value range of $barw$? (In addition) Shouldn't the $mu$ in your Log-likelihood is $lnmu$?
$endgroup$
– Tim Xu
Mar 21 at 12:08
$begingroup$
You are right about the log-likelihood. I will edit.
$endgroup$
– StubbornAtom
Mar 21 at 12:18
$begingroup$
@TimXu I just differentiated $ell$ once wrt $lambda$ and $mu$ and set them equal to zero (for multiple parameters, one should ideally check whether the Hessian matrix of the log-likelihood is negative definite at $(hatlambda,hatmu)$, provided the derivatives exist). And you can see from the expression for $hatlambda,hatmu$ that they are not defined at $bar w=0,1$. Hence the range distinction.
$endgroup$
– StubbornAtom
Mar 21 at 12:35
add a comment |
$begingroup$
Note that $Z$ and $W$ are in fact independent.
For $z>0,,,win0,1$, you can write the joint distribution of $(Z,W)$ as
$$P(z,w)=frac1lambda^w mu^1-wexpleft[-left(frac1lambda+frac1muright)zright]$$
The above formulation is not completely rigorous as it is not a probability (I am searching for a better notation avoiding the dirac delta).
The likelihood function given the sample $(z_1,w_1),ldots,(z_n,w_n)$ is then
$$L(lambda,mu)=frac1lambda^sum_i=1^n w_imu^n-sum_i=1^n w_iexpleft[-left(frac1lambda+frac1muright)sum_i=1^n z_iright]$$
Log-likelihood is
$$ell(lambda,mu)=-sum_i=1^n w_ilnlambda-left(n-sum_i=1^n w_iright)lnmu--left(frac1lambda+frac1muright)sum_i=1^n z_i$$
For $0<bar w<1$, solving for the stationary points of $ell(lambda,mu)$ yields $$hatlambda=fracsum_i=1^n z_isum_i=1^n w_i=fracbar zbar wqquad,qquad hatmu=fracsum_i=1^n z_in-sum_i=1^n w_i=fracbar z1-bar w$$
So assuming $0<bar w<1$, the unique MLE of $(lambda,mu)$ is $(hatlambda,hatmu)$.
But when $bar win0,1$, the MLE does not exist.
$endgroup$
Note that $Z$ and $W$ are in fact independent.
For $z>0,,,win0,1$, you can write the joint distribution of $(Z,W)$ as
$$P(z,w)=frac1lambda^w mu^1-wexpleft[-left(frac1lambda+frac1muright)zright]$$
The above formulation is not completely rigorous as it is not a probability (I am searching for a better notation avoiding the dirac delta).
The likelihood function given the sample $(z_1,w_1),ldots,(z_n,w_n)$ is then
$$L(lambda,mu)=frac1lambda^sum_i=1^n w_imu^n-sum_i=1^n w_iexpleft[-left(frac1lambda+frac1muright)sum_i=1^n z_iright]$$
Log-likelihood is
$$ell(lambda,mu)=-sum_i=1^n w_ilnlambda-left(n-sum_i=1^n w_iright)lnmu--left(frac1lambda+frac1muright)sum_i=1^n z_i$$
For $0<bar w<1$, solving for the stationary points of $ell(lambda,mu)$ yields $$hatlambda=fracsum_i=1^n z_isum_i=1^n w_i=fracbar zbar wqquad,qquad hatmu=fracsum_i=1^n z_in-sum_i=1^n w_i=fracbar z1-bar w$$
So assuming $0<bar w<1$, the unique MLE of $(lambda,mu)$ is $(hatlambda,hatmu)$.
But when $bar win0,1$, the MLE does not exist.
edited Mar 21 at 12:29
answered Mar 21 at 11:41


StubbornAtomStubbornAtom
6,29831440
6,29831440
$begingroup$
I still have two questions. (Q1) How to solve for the stationary points of $ell(lambda,mu)$? (Q2) Why do we need to discuss the value range of $barw$? (In addition) Shouldn't the $mu$ in your Log-likelihood is $lnmu$?
$endgroup$
– Tim Xu
Mar 21 at 12:08
$begingroup$
You are right about the log-likelihood. I will edit.
$endgroup$
– StubbornAtom
Mar 21 at 12:18
$begingroup$
@TimXu I just differentiated $ell$ once wrt $lambda$ and $mu$ and set them equal to zero (for multiple parameters, one should ideally check whether the Hessian matrix of the log-likelihood is negative definite at $(hatlambda,hatmu)$, provided the derivatives exist). And you can see from the expression for $hatlambda,hatmu$ that they are not defined at $bar w=0,1$. Hence the range distinction.
$endgroup$
– StubbornAtom
Mar 21 at 12:35
add a comment |
$begingroup$
I still have two questions. (Q1) How to solve for the stationary points of $ell(lambda,mu)$? (Q2) Why do we need to discuss the value range of $barw$? (In addition) Shouldn't the $mu$ in your Log-likelihood is $lnmu$?
$endgroup$
– Tim Xu
Mar 21 at 12:08
$begingroup$
You are right about the log-likelihood. I will edit.
$endgroup$
– StubbornAtom
Mar 21 at 12:18
$begingroup$
@TimXu I just differentiated $ell$ once wrt $lambda$ and $mu$ and set them equal to zero (for multiple parameters, one should ideally check whether the Hessian matrix of the log-likelihood is negative definite at $(hatlambda,hatmu)$, provided the derivatives exist). And you can see from the expression for $hatlambda,hatmu$ that they are not defined at $bar w=0,1$. Hence the range distinction.
$endgroup$
– StubbornAtom
Mar 21 at 12:35
$begingroup$
I still have two questions. (Q1) How to solve for the stationary points of $ell(lambda,mu)$? (Q2) Why do we need to discuss the value range of $barw$? (In addition) Shouldn't the $mu$ in your Log-likelihood is $lnmu$?
$endgroup$
– Tim Xu
Mar 21 at 12:08
$begingroup$
I still have two questions. (Q1) How to solve for the stationary points of $ell(lambda,mu)$? (Q2) Why do we need to discuss the value range of $barw$? (In addition) Shouldn't the $mu$ in your Log-likelihood is $lnmu$?
$endgroup$
– Tim Xu
Mar 21 at 12:08
$begingroup$
You are right about the log-likelihood. I will edit.
$endgroup$
– StubbornAtom
Mar 21 at 12:18
$begingroup$
You are right about the log-likelihood. I will edit.
$endgroup$
– StubbornAtom
Mar 21 at 12:18
$begingroup$
@TimXu I just differentiated $ell$ once wrt $lambda$ and $mu$ and set them equal to zero (for multiple parameters, one should ideally check whether the Hessian matrix of the log-likelihood is negative definite at $(hatlambda,hatmu)$, provided the derivatives exist). And you can see from the expression for $hatlambda,hatmu$ that they are not defined at $bar w=0,1$. Hence the range distinction.
$endgroup$
– StubbornAtom
Mar 21 at 12:35
$begingroup$
@TimXu I just differentiated $ell$ once wrt $lambda$ and $mu$ and set them equal to zero (for multiple parameters, one should ideally check whether the Hessian matrix of the log-likelihood is negative definite at $(hatlambda,hatmu)$, provided the derivatives exist). And you can see from the expression for $hatlambda,hatmu$ that they are not defined at $bar w=0,1$. Hence the range distinction.
$endgroup$
– StubbornAtom
Mar 21 at 12:35
add a comment |
$begingroup$
The $W=0$ and the $W=1$ case can be combined by writing $lambda^1-Wmu^W$.
First differentiating w.r.t. $z$ and forming the product the likelihood can be written as $prod_i^nfrac1lambda^w_imu^1-w_ie^-(frac1lambda+frac1mu)z_i$.
Taking logs gives the log likelihood $=-sum_i^n(w_ilnlambda+(1-w_i)lnmu+(frac1lambda+frac1mu)z_i)$.
Maximising wrt $lambda$ and $mu$ gives $lambda=fracbarzbarw$ and $mu=fracbarz(1-barw)$
Note if $lambda=mu$ then $barwapproxfrac12$ so the estimates for $lambda$ and $mu$ become equal.
$endgroup$
$begingroup$
Denote the log likelihood by $log L$. First differentiating w.r.t. $lambda$ and $mu$, gives $hatlambda=fracbarzbarw$, $hatmu=fracbarz1-barw$. To make sure they are the maximizers of the log likelihood. I calculate their second-order differentials w.r.t. $lambda$ and $mu$, and get that $fracpartial^2 log Lpartial lambda^2=-sum_i=1^n(-fracw_ilambda^2+2fracz_ilambda^3)$ and $fracpartial^2 log Lpartial mu^2=-sum_i=1^n(-frac1-w_imu^2+2fracz_imu^3)$. How do you know these two second-order differentials are negative?
$endgroup$
– Tim Xu
Mar 21 at 11:44
$begingroup$
Direct substitution. for instance $fracpartial^2 logLpartial lambda^2=-fracbarw^3barz^2$
$endgroup$
– user121049
Mar 21 at 14:09
$begingroup$
You missed an $n$. It is $fracpartial ^2 logLpartial lambda^2 |_lambda=fracbar zbar w= -sum_i=1^n (-fracw_ilambda^2+2fracz_ilambda^3) |_lambda=fracbar zbar w= (fracsum_i=1^n w_ilambda^2-2fracsum_i=1^n z_ilambda^3) |_lambda=fracbar zbar w= (fracn bar wlambda^2-2fracn bar zlambda^3) |_lambda=fracbar zbar w= fracn bar w^3bar z^2-2fracn bar z bar w^3bar z^3= fracn bar w^3bar z^2-2fracn bar w^3bar z^2=-fracn bar w^3bar z^2$
$endgroup$
– Tim Xu
Mar 22 at 6:41
add a comment |
$begingroup$
The $W=0$ and the $W=1$ case can be combined by writing $lambda^1-Wmu^W$.
First differentiating w.r.t. $z$ and forming the product the likelihood can be written as $prod_i^nfrac1lambda^w_imu^1-w_ie^-(frac1lambda+frac1mu)z_i$.
Taking logs gives the log likelihood $=-sum_i^n(w_ilnlambda+(1-w_i)lnmu+(frac1lambda+frac1mu)z_i)$.
Maximising wrt $lambda$ and $mu$ gives $lambda=fracbarzbarw$ and $mu=fracbarz(1-barw)$
Note if $lambda=mu$ then $barwapproxfrac12$ so the estimates for $lambda$ and $mu$ become equal.
$endgroup$
$begingroup$
Denote the log likelihood by $log L$. First differentiating w.r.t. $lambda$ and $mu$, gives $hatlambda=fracbarzbarw$, $hatmu=fracbarz1-barw$. To make sure they are the maximizers of the log likelihood. I calculate their second-order differentials w.r.t. $lambda$ and $mu$, and get that $fracpartial^2 log Lpartial lambda^2=-sum_i=1^n(-fracw_ilambda^2+2fracz_ilambda^3)$ and $fracpartial^2 log Lpartial mu^2=-sum_i=1^n(-frac1-w_imu^2+2fracz_imu^3)$. How do you know these two second-order differentials are negative?
$endgroup$
– Tim Xu
Mar 21 at 11:44
$begingroup$
Direct substitution. for instance $fracpartial^2 logLpartial lambda^2=-fracbarw^3barz^2$
$endgroup$
– user121049
Mar 21 at 14:09
$begingroup$
You missed an $n$. It is $fracpartial ^2 logLpartial lambda^2 |_lambda=fracbar zbar w= -sum_i=1^n (-fracw_ilambda^2+2fracz_ilambda^3) |_lambda=fracbar zbar w= (fracsum_i=1^n w_ilambda^2-2fracsum_i=1^n z_ilambda^3) |_lambda=fracbar zbar w= (fracn bar wlambda^2-2fracn bar zlambda^3) |_lambda=fracbar zbar w= fracn bar w^3bar z^2-2fracn bar z bar w^3bar z^3= fracn bar w^3bar z^2-2fracn bar w^3bar z^2=-fracn bar w^3bar z^2$
$endgroup$
– Tim Xu
Mar 22 at 6:41
add a comment |
$begingroup$
The $W=0$ and the $W=1$ case can be combined by writing $lambda^1-Wmu^W$.
First differentiating w.r.t. $z$ and forming the product the likelihood can be written as $prod_i^nfrac1lambda^w_imu^1-w_ie^-(frac1lambda+frac1mu)z_i$.
Taking logs gives the log likelihood $=-sum_i^n(w_ilnlambda+(1-w_i)lnmu+(frac1lambda+frac1mu)z_i)$.
Maximising wrt $lambda$ and $mu$ gives $lambda=fracbarzbarw$ and $mu=fracbarz(1-barw)$
Note if $lambda=mu$ then $barwapproxfrac12$ so the estimates for $lambda$ and $mu$ become equal.
$endgroup$
The $W=0$ and the $W=1$ case can be combined by writing $lambda^1-Wmu^W$.
First differentiating w.r.t. $z$ and forming the product the likelihood can be written as $prod_i^nfrac1lambda^w_imu^1-w_ie^-(frac1lambda+frac1mu)z_i$.
Taking logs gives the log likelihood $=-sum_i^n(w_ilnlambda+(1-w_i)lnmu+(frac1lambda+frac1mu)z_i)$.
Maximising wrt $lambda$ and $mu$ gives $lambda=fracbarzbarw$ and $mu=fracbarz(1-barw)$
Note if $lambda=mu$ then $barwapproxfrac12$ so the estimates for $lambda$ and $mu$ become equal.
answered Mar 21 at 8:40
user121049user121049
1,360174
1,360174
$begingroup$
Denote the log likelihood by $log L$. First differentiating w.r.t. $lambda$ and $mu$, gives $hatlambda=fracbarzbarw$, $hatmu=fracbarz1-barw$. To make sure they are the maximizers of the log likelihood. I calculate their second-order differentials w.r.t. $lambda$ and $mu$, and get that $fracpartial^2 log Lpartial lambda^2=-sum_i=1^n(-fracw_ilambda^2+2fracz_ilambda^3)$ and $fracpartial^2 log Lpartial mu^2=-sum_i=1^n(-frac1-w_imu^2+2fracz_imu^3)$. How do you know these two second-order differentials are negative?
$endgroup$
– Tim Xu
Mar 21 at 11:44
$begingroup$
Direct substitution. for instance $fracpartial^2 logLpartial lambda^2=-fracbarw^3barz^2$
$endgroup$
– user121049
Mar 21 at 14:09
$begingroup$
You missed an $n$. It is $fracpartial ^2 logLpartial lambda^2 |_lambda=fracbar zbar w= -sum_i=1^n (-fracw_ilambda^2+2fracz_ilambda^3) |_lambda=fracbar zbar w= (fracsum_i=1^n w_ilambda^2-2fracsum_i=1^n z_ilambda^3) |_lambda=fracbar zbar w= (fracn bar wlambda^2-2fracn bar zlambda^3) |_lambda=fracbar zbar w= fracn bar w^3bar z^2-2fracn bar z bar w^3bar z^3= fracn bar w^3bar z^2-2fracn bar w^3bar z^2=-fracn bar w^3bar z^2$
$endgroup$
– Tim Xu
Mar 22 at 6:41
add a comment |
$begingroup$
Denote the log likelihood by $log L$. First differentiating w.r.t. $lambda$ and $mu$, gives $hatlambda=fracbarzbarw$, $hatmu=fracbarz1-barw$. To make sure they are the maximizers of the log likelihood. I calculate their second-order differentials w.r.t. $lambda$ and $mu$, and get that $fracpartial^2 log Lpartial lambda^2=-sum_i=1^n(-fracw_ilambda^2+2fracz_ilambda^3)$ and $fracpartial^2 log Lpartial mu^2=-sum_i=1^n(-frac1-w_imu^2+2fracz_imu^3)$. How do you know these two second-order differentials are negative?
$endgroup$
– Tim Xu
Mar 21 at 11:44
$begingroup$
Direct substitution. for instance $fracpartial^2 logLpartial lambda^2=-fracbarw^3barz^2$
$endgroup$
– user121049
Mar 21 at 14:09
$begingroup$
You missed an $n$. It is $fracpartial ^2 logLpartial lambda^2 |_lambda=fracbar zbar w= -sum_i=1^n (-fracw_ilambda^2+2fracz_ilambda^3) |_lambda=fracbar zbar w= (fracsum_i=1^n w_ilambda^2-2fracsum_i=1^n z_ilambda^3) |_lambda=fracbar zbar w= (fracn bar wlambda^2-2fracn bar zlambda^3) |_lambda=fracbar zbar w= fracn bar w^3bar z^2-2fracn bar z bar w^3bar z^3= fracn bar w^3bar z^2-2fracn bar w^3bar z^2=-fracn bar w^3bar z^2$
$endgroup$
– Tim Xu
Mar 22 at 6:41
$begingroup$
Denote the log likelihood by $log L$. First differentiating w.r.t. $lambda$ and $mu$, gives $hatlambda=fracbarzbarw$, $hatmu=fracbarz1-barw$. To make sure they are the maximizers of the log likelihood. I calculate their second-order differentials w.r.t. $lambda$ and $mu$, and get that $fracpartial^2 log Lpartial lambda^2=-sum_i=1^n(-fracw_ilambda^2+2fracz_ilambda^3)$ and $fracpartial^2 log Lpartial mu^2=-sum_i=1^n(-frac1-w_imu^2+2fracz_imu^3)$. How do you know these two second-order differentials are negative?
$endgroup$
– Tim Xu
Mar 21 at 11:44
$begingroup$
Denote the log likelihood by $log L$. First differentiating w.r.t. $lambda$ and $mu$, gives $hatlambda=fracbarzbarw$, $hatmu=fracbarz1-barw$. To make sure they are the maximizers of the log likelihood. I calculate their second-order differentials w.r.t. $lambda$ and $mu$, and get that $fracpartial^2 log Lpartial lambda^2=-sum_i=1^n(-fracw_ilambda^2+2fracz_ilambda^3)$ and $fracpartial^2 log Lpartial mu^2=-sum_i=1^n(-frac1-w_imu^2+2fracz_imu^3)$. How do you know these two second-order differentials are negative?
$endgroup$
– Tim Xu
Mar 21 at 11:44
$begingroup$
Direct substitution. for instance $fracpartial^2 logLpartial lambda^2=-fracbarw^3barz^2$
$endgroup$
– user121049
Mar 21 at 14:09
$begingroup$
Direct substitution. for instance $fracpartial^2 logLpartial lambda^2=-fracbarw^3barz^2$
$endgroup$
– user121049
Mar 21 at 14:09
$begingroup$
You missed an $n$. It is $fracpartial ^2 logLpartial lambda^2 |_lambda=fracbar zbar w= -sum_i=1^n (-fracw_ilambda^2+2fracz_ilambda^3) |_lambda=fracbar zbar w= (fracsum_i=1^n w_ilambda^2-2fracsum_i=1^n z_ilambda^3) |_lambda=fracbar zbar w= (fracn bar wlambda^2-2fracn bar zlambda^3) |_lambda=fracbar zbar w= fracn bar w^3bar z^2-2fracn bar z bar w^3bar z^3= fracn bar w^3bar z^2-2fracn bar w^3bar z^2=-fracn bar w^3bar z^2$
$endgroup$
– Tim Xu
Mar 22 at 6:41
$begingroup$
You missed an $n$. It is $fracpartial ^2 logLpartial lambda^2 |_lambda=fracbar zbar w= -sum_i=1^n (-fracw_ilambda^2+2fracz_ilambda^3) |_lambda=fracbar zbar w= (fracsum_i=1^n w_ilambda^2-2fracsum_i=1^n z_ilambda^3) |_lambda=fracbar zbar w= (fracn bar wlambda^2-2fracn bar zlambda^3) |_lambda=fracbar zbar w= fracn bar w^3bar z^2-2fracn bar z bar w^3bar z^3= fracn bar w^3bar z^2-2fracn bar w^3bar z^2=-fracn bar w^3bar z^2$
$endgroup$
– Tim Xu
Mar 22 at 6:41
add a comment |
Thanks for contributing an answer to Mathematics Stack Exchange!
- Please be sure to answer the question. Provide details and share your research!
But avoid …
- Asking for help, clarification, or responding to other answers.
- Making statements based on opinion; back them up with references or personal experience.
Use MathJax to format equations. MathJax reference.
To learn more, see our tips on writing great answers.
Sign up or log in
StackExchange.ready(function ()
StackExchange.helpers.onClickDraftSave('#login-link');
);
Sign up using Google
Sign up using Facebook
Sign up using Email and Password
Post as a guest
Required, but never shown
StackExchange.ready(
function ()
StackExchange.openid.initPostLogin('.new-post-login', 'https%3a%2f%2fmath.stackexchange.com%2fquestions%2f3156467%2fhow-to-find-the-mle-of-these-parameters-given-distribution%23new-answer', 'question_page');
);
Post as a guest
Required, but never shown
Sign up or log in
StackExchange.ready(function ()
StackExchange.helpers.onClickDraftSave('#login-link');
);
Sign up using Google
Sign up using Facebook
Sign up using Email and Password
Post as a guest
Required, but never shown
Sign up or log in
StackExchange.ready(function ()
StackExchange.helpers.onClickDraftSave('#login-link');
);
Sign up using Google
Sign up using Facebook
Sign up using Email and Password
Post as a guest
Required, but never shown
Sign up or log in
StackExchange.ready(function ()
StackExchange.helpers.onClickDraftSave('#login-link');
);
Sign up using Google
Sign up using Facebook
Sign up using Email and Password
Sign up using Google
Sign up using Facebook
Sign up using Email and Password
Post as a guest
Required, but never shown
Required, but never shown
Required, but never shown
Required, but never shown
Required, but never shown
Required, but never shown
Required, but never shown
Required, but never shown
Required, but never shown
22q7ZDmnQnIkE92LJ9Cut,1ae 0bOYjzhilU NEQ0kIxproDEbwhK3Yz,4dcIK8jczho07 SdUD l0ZRF2J,tCgrNE