What is degree of freedom in statistics? Announcing the arrival of Valued Associate #679: Cesar Manara Planned maintenance scheduled April 17/18, 2019 at 00:00UTC (8:00pm US/Eastern)degree of freedom, when to $-1$ or $-2$?degree of freedom, when to $-1$ or $-2$?Understanding degrees of freedomLinear regression: degrees of freedom of SST, SSR, and RSSChi Square Formula and Degrees of Freedom QuestionsDegree of freedomCalculating a statistic of specific distributions and degrees of freedomSampling a multivariate t distribution with less than 2 degrees of freedomChi-squared test : probabilityANOVA MST: why divide SST by degree of freedom?How to proper determinate the number of degrees of freedom using the Pearson Chi-Square test?
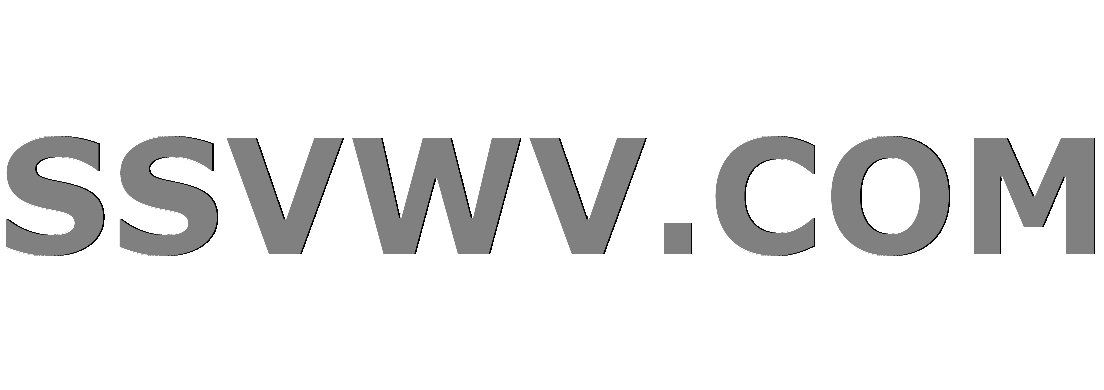
Multi tool use
An adverb for when you're not exaggerating
Can an alien society believe that their star system is the universe?
Withdrew £2800, but only £2000 shows as withdrawn on online banking; what are my obligations?
Do I really need recursive chmod to restrict access to a folder?
Do I really need to have a message in a novel to appeal to readers?
Can anything be seen from the center of the Boötes void? How dark would it be?
How to convince students of the implication truth values?
Fundamental Solution of the Pell Equation
Does classifying an integer as a discrete log require it be part of a multiplicative group?
Is there a kind of relay only consumes power when switching?
How to Make a Beautiful Stacked 3D Plot
If a VARCHAR(MAX) column is included in an index, is the entire value always stored in the index page(s)?
また usage in a dictionary
Has negative voting ever been officially implemented in elections, or seriously proposed, or even studied?
Generate an RGB colour grid
Irreducible of finite Krull dimension implies quasi-compact?
Why are both D and D# fitting into my E minor key?
Chinese Seal on silk painting - what does it mean?
What is the longest distance a player character can jump in one leap?
How to compare two different files line by line in unix?
Is grep documentation wrong?
Denied boarding although I have proper visa and documentation. To whom should I make a complaint?
Is CEO the profession with the most psychopaths?
Closed form of recurrent arithmetic series summation
What is degree of freedom in statistics?
Announcing the arrival of Valued Associate #679: Cesar Manara
Planned maintenance scheduled April 17/18, 2019 at 00:00UTC (8:00pm US/Eastern)degree of freedom, when to $-1$ or $-2$?degree of freedom, when to $-1$ or $-2$?Understanding degrees of freedomLinear regression: degrees of freedom of SST, SSR, and RSSChi Square Formula and Degrees of Freedom QuestionsDegree of freedomCalculating a statistic of specific distributions and degrees of freedomSampling a multivariate t distribution with less than 2 degrees of freedomChi-squared test : probabilityANOVA MST: why divide SST by degree of freedom?How to proper determinate the number of degrees of freedom using the Pearson Chi-Square test?
$begingroup$
In statistics, degree of freedom is widely used in regression analysis, ANOVA and so on. But, what is degree of freedom ?
Wikipedia said that
The number of degrees of freedom is the number of values in the final calculation of a statistic that are free to vary.
Mathematically, degrees of freedom is the number of dimension of the domain of a random vector, or essentially the number of 'free'
components: how many components need to be known before the vector is
fully determined.
However, it is still hard for me to understand the concept intuitively.
Question:
Could anyone provide a intuitive explain to the concept and anything can help me understand?
Thanks!
Update:
I'm NOT asking how to calculate degree of freedom.
Let me give an example:
For Chi-squared distribution, different degree of freedom produces different probability density function. Could you explain it intuitively?
statistics
$endgroup$
add a comment |
$begingroup$
In statistics, degree of freedom is widely used in regression analysis, ANOVA and so on. But, what is degree of freedom ?
Wikipedia said that
The number of degrees of freedom is the number of values in the final calculation of a statistic that are free to vary.
Mathematically, degrees of freedom is the number of dimension of the domain of a random vector, or essentially the number of 'free'
components: how many components need to be known before the vector is
fully determined.
However, it is still hard for me to understand the concept intuitively.
Question:
Could anyone provide a intuitive explain to the concept and anything can help me understand?
Thanks!
Update:
I'm NOT asking how to calculate degree of freedom.
Let me give an example:
For Chi-squared distribution, different degree of freedom produces different probability density function. Could you explain it intuitively?
statistics
$endgroup$
$begingroup$
On CV:stats.stackexchange.com/questions/16921/….
$endgroup$
– StubbornAtom
Mar 22 '18 at 21:07
add a comment |
$begingroup$
In statistics, degree of freedom is widely used in regression analysis, ANOVA and so on. But, what is degree of freedom ?
Wikipedia said that
The number of degrees of freedom is the number of values in the final calculation of a statistic that are free to vary.
Mathematically, degrees of freedom is the number of dimension of the domain of a random vector, or essentially the number of 'free'
components: how many components need to be known before the vector is
fully determined.
However, it is still hard for me to understand the concept intuitively.
Question:
Could anyone provide a intuitive explain to the concept and anything can help me understand?
Thanks!
Update:
I'm NOT asking how to calculate degree of freedom.
Let me give an example:
For Chi-squared distribution, different degree of freedom produces different probability density function. Could you explain it intuitively?
statistics
$endgroup$
In statistics, degree of freedom is widely used in regression analysis, ANOVA and so on. But, what is degree of freedom ?
Wikipedia said that
The number of degrees of freedom is the number of values in the final calculation of a statistic that are free to vary.
Mathematically, degrees of freedom is the number of dimension of the domain of a random vector, or essentially the number of 'free'
components: how many components need to be known before the vector is
fully determined.
However, it is still hard for me to understand the concept intuitively.
Question:
Could anyone provide a intuitive explain to the concept and anything can help me understand?
Thanks!
Update:
I'm NOT asking how to calculate degree of freedom.
Let me give an example:
For Chi-squared distribution, different degree of freedom produces different probability density function. Could you explain it intuitively?
statistics
statistics
edited Nov 15 '12 at 10:24
John Hass
asked Nov 15 '12 at 7:04
John HassJohn Hass
2,00371943
2,00371943
$begingroup$
On CV:stats.stackexchange.com/questions/16921/….
$endgroup$
– StubbornAtom
Mar 22 '18 at 21:07
add a comment |
$begingroup$
On CV:stats.stackexchange.com/questions/16921/….
$endgroup$
– StubbornAtom
Mar 22 '18 at 21:07
$begingroup$
On CV:stats.stackexchange.com/questions/16921/….
$endgroup$
– StubbornAtom
Mar 22 '18 at 21:07
$begingroup$
On CV:stats.stackexchange.com/questions/16921/….
$endgroup$
– StubbornAtom
Mar 22 '18 at 21:07
add a comment |
5 Answers
5
active
oldest
votes
$begingroup$
Intuitively degrees of freedom denotes how many independent things are there. As we introduce constraints, we take away the degree of freedom.
First I'll try to answer your question about Chi-square.
Chi-square distribution with $n$ degree of freedom is the sum of squares $n$ independent standard normal distributions $N(0,1)$ hence we've got $n$ things that vary independently.
I'll start with mechanical example, as degree of freedom is similar in every field.
Consider an airplane flying. It has three degrees of freedom in the usual universe of space, and can be located only if three coordinates are known. These might be latitude, longitude, and altitude; or might be altitude, horizontal distance from some origin, and an angle; or might be direct distance from some origin, and two direction angles. If we consider a given instant of time as a section through the space-time universe, the airplane moves in a four‑dimensional path and can be located by four coordinates, the three previously named and a time coordinate.Hence it now has $4$ d.f.
Note that we assumed that plane is not rotating.
Now considering statistical degrees of freedom..
Similar meaning.
Degree of freedom of a statistic is number of values in calculation of statistic that are independent to vary.As we add restriction to observations, we reduce the degree of freedom.Imposing a relationship upon the observations is equivalent to estimating a parameter from them. The number of degrees of freedom is equal to the number of independent observations, which is the number of original observations minus the number of parmeters estimated from them.
Consider the calculation of mean $frac sum_i=1^n X_n n$, we are interested in estimation of error which are estimated by residues. Sum of residuals is $0$. Knowledge of any $n-1$ residues gives the remaining residue. So, only $n-1$ can vary independently. Hence they have $n-1$ d.f.
However d.f is mainly used in regression analysis, and ANOVA. You may note that all the distributions with so called d.f correspond to particular cases in linear statistics. Hence d.f is at the best artificial as they are not constraints on the random variable, but are actually degree of freedom of some quantities in some application from where these distributions originated.
Also, For people who are interested, < http://courses.ncssm.edu/math/Stat_Inst/Worddocs/DFWalker.doc > seems to be quite good read.
$endgroup$
$begingroup$
Thanks. I have gave you an upvote. Another question here: What's the difference between degree of freedom and dimension in your mechanical example? : )
$endgroup$
– John Hass
Nov 16 '12 at 11:00
1
$begingroup$
Degree of freedom for a prticle is the number of dimensions only if the motion is not constrained. For an example, consider a particle hanging down from ceiling with the help of a rigid rod, then degree of freedom is less than $3$, right?(it is actually $2$.). Any two independent parameters give all information about the position of particle. (Try to give position using two parameters). If you still have questions, ask. I'll be glad to help if I can..
$endgroup$
– TheJoker
Nov 16 '12 at 16:42
add a comment |
$begingroup$
Two people are sitting at a bar, you and your friend. There are two sorts of juice before you, one sweet, one sour. After you have chosen your drink, say the sweet one, your friend has no more choice - so degree of freedom is "1": only one of you can choose.
Generalize it to a group of friends to understand higher degrees of freedom...
$endgroup$
add a comment |
$begingroup$
When you estimate parameters in statistics, suppose you use a parameter (which you have estimated) to estimate another parameter, then you lose $1$ degree of freedom and you end up with $n-1$ degrees of freedom where $n$ is the number of 'individuals' in the population with which you are working.
One example is when working with normal distributions
$$sigma=sqrtfracsum(x-mu)n^2$$
let $s$ be the estimate of the population standard deviation ($sigma$). We use the mean value $bar x$ instead of $mu$.
$$s=sqrtfracsum(x-bar x)n-1^2$$
Here $bar x$ is an estimate of $mu$. We estimate $sigma$ by using an estimate of $mu$. Hence we lose $1$ of degree of freedom, resulting in $n-1$ degrees of freedom.
$endgroup$
add a comment |
$begingroup$
I've always viewed "degrees of freedom" as a measure of the number of choices that are available to you in a situation. This of course is not meant to be rigorous, but works intuitively in examples.
Consider the problem of choosing a polynomial of degree $2$ (with coefficients in some fixed field $k$). Then we have three choices to make, corresponding to the selection of $a_0,a_1,a_2$ in
$$f(x):=a_2x^2+a_1x+a_0.$$
We should then expect the number of degrees of freedom in this example to be three. And indeed, the space of such polynomials is a vector space, of degree $3$. If we suppose now that we only care for polynomials admitting $x=1$ as a root, we have -- on an intuitive level -- used up one of our degrees of freedom, and we should expect to be able to make two more independent choices. This is indeed the case: we are left with a vector space of dimension $2$.
Note that these notions only really make sense for vector spaces and "choices" that correspond to the intersection of hyperplanes. This all can be generalized within the framework of algebraic geometry (and intersection theory), but matters become complicated when hypersurfaces need not intersect as expected.
$endgroup$
add a comment |
$begingroup$
To understand the concept intuitively, ask the question of why it is needed. And start from a simpler question, why is the variance computed as $V = frac 1 N-1 sum (x_i-bar x)^2$ with a $N-1$ as denominator.
The fact is that you are interested in the variance of the population, not of the one of sample. Now obviously the sample is less dispersed than the population (because it is very likely that your sample missed a few of the extreme values), so the variance computed on the sample is lower than the variance of the population.
You have to correct the bias.
Intuitively, the observed average $bar x = frac 1 N sum x_i$ is not exact but only an approximation of the population mean. The variance of this approximation should be added to the observed variance on the sample in order to to get the best approximate of the population sample.
Now this variance can be computed: $sigma^2(bar X) = frac 1 N sigma^2(X)$ (using the iid if the $X_i$). So the sample variance is $1 -frac 1 N$ the variance of the population.
Formally (in case you need to read twice the previous reasoning), compute the expected value of $sum (X_i-bar X)^2$. You will find $(N-1) sigma^2$ rather than $N sigma^2$, hence the population variance is $frac N N-1$ the sample variance (as claimed).
When you follow the computations, you start by replacing $bar X$ by its definition $bar X = frac 1 N sum X_i$, develop the squares, expand the sum, and then one of the term disappears. Namely $Nbar X=sum X_i$ appears twice with opposite sign, negative in the double product $2 bar X X$ and positive in the square $bar X^2$. So $sum (X_i-bar X)^2$ is the sum of $N-1$ terms equal in expectation.
This is because the $X_i$ are not independent but linked by one relation $sum X_i=N bar X$. In general, if you know the $X_i$ are linked by $p$ independent linear relations $f_j$, then you can cancel out $p$ terms out of the sum $sum (X_i-f_j(X_i))^2$. Hence the unbiased estimator, $sum (X_i-f_j(X_i))^2 approx frac N N-p sum (x_i-f_j(x_i))^2$.
In regression, ANOVA, etc, the independents relations are not so independent because it is often supposed that the sum of the independents variables (causes) have the same average than the dependent variable (effect). $sum a_i bar X_i = bar Y$. Hence the degree of freedom $N-1$, $p-1$ and $N-p$ and unbiasing factors $frac N N-1$, $frac p p-1$ and $frac N N-p$ for $SS_Total$, $SS_Model$ and $SS_Error$ respectively.
In two words, the degree of freedom is the number of independent relationships linking a set of variables, taking into account the variable introduced for intermediary estimators.
$endgroup$
add a comment |
Your Answer
StackExchange.ready(function()
var channelOptions =
tags: "".split(" "),
id: "69"
;
initTagRenderer("".split(" "), "".split(" "), channelOptions);
StackExchange.using("externalEditor", function()
// Have to fire editor after snippets, if snippets enabled
if (StackExchange.settings.snippets.snippetsEnabled)
StackExchange.using("snippets", function()
createEditor();
);
else
createEditor();
);
function createEditor()
StackExchange.prepareEditor(
heartbeatType: 'answer',
autoActivateHeartbeat: false,
convertImagesToLinks: true,
noModals: true,
showLowRepImageUploadWarning: true,
reputationToPostImages: 10,
bindNavPrevention: true,
postfix: "",
imageUploader:
brandingHtml: "Powered by u003ca class="icon-imgur-white" href="https://imgur.com/"u003eu003c/au003e",
contentPolicyHtml: "User contributions licensed under u003ca href="https://creativecommons.org/licenses/by-sa/3.0/"u003ecc by-sa 3.0 with attribution requiredu003c/au003e u003ca href="https://stackoverflow.com/legal/content-policy"u003e(content policy)u003c/au003e",
allowUrls: true
,
noCode: true, onDemand: true,
discardSelector: ".discard-answer"
,immediatelyShowMarkdownHelp:true
);
);
Sign up or log in
StackExchange.ready(function ()
StackExchange.helpers.onClickDraftSave('#login-link');
);
Sign up using Google
Sign up using Facebook
Sign up using Email and Password
Post as a guest
Required, but never shown
StackExchange.ready(
function ()
StackExchange.openid.initPostLogin('.new-post-login', 'https%3a%2f%2fmath.stackexchange.com%2fquestions%2f237790%2fwhat-is-degree-of-freedom-in-statistics%23new-answer', 'question_page');
);
Post as a guest
Required, but never shown
5 Answers
5
active
oldest
votes
5 Answers
5
active
oldest
votes
active
oldest
votes
active
oldest
votes
$begingroup$
Intuitively degrees of freedom denotes how many independent things are there. As we introduce constraints, we take away the degree of freedom.
First I'll try to answer your question about Chi-square.
Chi-square distribution with $n$ degree of freedom is the sum of squares $n$ independent standard normal distributions $N(0,1)$ hence we've got $n$ things that vary independently.
I'll start with mechanical example, as degree of freedom is similar in every field.
Consider an airplane flying. It has three degrees of freedom in the usual universe of space, and can be located only if three coordinates are known. These might be latitude, longitude, and altitude; or might be altitude, horizontal distance from some origin, and an angle; or might be direct distance from some origin, and two direction angles. If we consider a given instant of time as a section through the space-time universe, the airplane moves in a four‑dimensional path and can be located by four coordinates, the three previously named and a time coordinate.Hence it now has $4$ d.f.
Note that we assumed that plane is not rotating.
Now considering statistical degrees of freedom..
Similar meaning.
Degree of freedom of a statistic is number of values in calculation of statistic that are independent to vary.As we add restriction to observations, we reduce the degree of freedom.Imposing a relationship upon the observations is equivalent to estimating a parameter from them. The number of degrees of freedom is equal to the number of independent observations, which is the number of original observations minus the number of parmeters estimated from them.
Consider the calculation of mean $frac sum_i=1^n X_n n$, we are interested in estimation of error which are estimated by residues. Sum of residuals is $0$. Knowledge of any $n-1$ residues gives the remaining residue. So, only $n-1$ can vary independently. Hence they have $n-1$ d.f.
However d.f is mainly used in regression analysis, and ANOVA. You may note that all the distributions with so called d.f correspond to particular cases in linear statistics. Hence d.f is at the best artificial as they are not constraints on the random variable, but are actually degree of freedom of some quantities in some application from where these distributions originated.
Also, For people who are interested, < http://courses.ncssm.edu/math/Stat_Inst/Worddocs/DFWalker.doc > seems to be quite good read.
$endgroup$
$begingroup$
Thanks. I have gave you an upvote. Another question here: What's the difference between degree of freedom and dimension in your mechanical example? : )
$endgroup$
– John Hass
Nov 16 '12 at 11:00
1
$begingroup$
Degree of freedom for a prticle is the number of dimensions only if the motion is not constrained. For an example, consider a particle hanging down from ceiling with the help of a rigid rod, then degree of freedom is less than $3$, right?(it is actually $2$.). Any two independent parameters give all information about the position of particle. (Try to give position using two parameters). If you still have questions, ask. I'll be glad to help if I can..
$endgroup$
– TheJoker
Nov 16 '12 at 16:42
add a comment |
$begingroup$
Intuitively degrees of freedom denotes how many independent things are there. As we introduce constraints, we take away the degree of freedom.
First I'll try to answer your question about Chi-square.
Chi-square distribution with $n$ degree of freedom is the sum of squares $n$ independent standard normal distributions $N(0,1)$ hence we've got $n$ things that vary independently.
I'll start with mechanical example, as degree of freedom is similar in every field.
Consider an airplane flying. It has three degrees of freedom in the usual universe of space, and can be located only if three coordinates are known. These might be latitude, longitude, and altitude; or might be altitude, horizontal distance from some origin, and an angle; or might be direct distance from some origin, and two direction angles. If we consider a given instant of time as a section through the space-time universe, the airplane moves in a four‑dimensional path and can be located by four coordinates, the three previously named and a time coordinate.Hence it now has $4$ d.f.
Note that we assumed that plane is not rotating.
Now considering statistical degrees of freedom..
Similar meaning.
Degree of freedom of a statistic is number of values in calculation of statistic that are independent to vary.As we add restriction to observations, we reduce the degree of freedom.Imposing a relationship upon the observations is equivalent to estimating a parameter from them. The number of degrees of freedom is equal to the number of independent observations, which is the number of original observations minus the number of parmeters estimated from them.
Consider the calculation of mean $frac sum_i=1^n X_n n$, we are interested in estimation of error which are estimated by residues. Sum of residuals is $0$. Knowledge of any $n-1$ residues gives the remaining residue. So, only $n-1$ can vary independently. Hence they have $n-1$ d.f.
However d.f is mainly used in regression analysis, and ANOVA. You may note that all the distributions with so called d.f correspond to particular cases in linear statistics. Hence d.f is at the best artificial as they are not constraints on the random variable, but are actually degree of freedom of some quantities in some application from where these distributions originated.
Also, For people who are interested, < http://courses.ncssm.edu/math/Stat_Inst/Worddocs/DFWalker.doc > seems to be quite good read.
$endgroup$
$begingroup$
Thanks. I have gave you an upvote. Another question here: What's the difference between degree of freedom and dimension in your mechanical example? : )
$endgroup$
– John Hass
Nov 16 '12 at 11:00
1
$begingroup$
Degree of freedom for a prticle is the number of dimensions only if the motion is not constrained. For an example, consider a particle hanging down from ceiling with the help of a rigid rod, then degree of freedom is less than $3$, right?(it is actually $2$.). Any two independent parameters give all information about the position of particle. (Try to give position using two parameters). If you still have questions, ask. I'll be glad to help if I can..
$endgroup$
– TheJoker
Nov 16 '12 at 16:42
add a comment |
$begingroup$
Intuitively degrees of freedom denotes how many independent things are there. As we introduce constraints, we take away the degree of freedom.
First I'll try to answer your question about Chi-square.
Chi-square distribution with $n$ degree of freedom is the sum of squares $n$ independent standard normal distributions $N(0,1)$ hence we've got $n$ things that vary independently.
I'll start with mechanical example, as degree of freedom is similar in every field.
Consider an airplane flying. It has three degrees of freedom in the usual universe of space, and can be located only if three coordinates are known. These might be latitude, longitude, and altitude; or might be altitude, horizontal distance from some origin, and an angle; or might be direct distance from some origin, and two direction angles. If we consider a given instant of time as a section through the space-time universe, the airplane moves in a four‑dimensional path and can be located by four coordinates, the three previously named and a time coordinate.Hence it now has $4$ d.f.
Note that we assumed that plane is not rotating.
Now considering statistical degrees of freedom..
Similar meaning.
Degree of freedom of a statistic is number of values in calculation of statistic that are independent to vary.As we add restriction to observations, we reduce the degree of freedom.Imposing a relationship upon the observations is equivalent to estimating a parameter from them. The number of degrees of freedom is equal to the number of independent observations, which is the number of original observations minus the number of parmeters estimated from them.
Consider the calculation of mean $frac sum_i=1^n X_n n$, we are interested in estimation of error which are estimated by residues. Sum of residuals is $0$. Knowledge of any $n-1$ residues gives the remaining residue. So, only $n-1$ can vary independently. Hence they have $n-1$ d.f.
However d.f is mainly used in regression analysis, and ANOVA. You may note that all the distributions with so called d.f correspond to particular cases in linear statistics. Hence d.f is at the best artificial as they are not constraints on the random variable, but are actually degree of freedom of some quantities in some application from where these distributions originated.
Also, For people who are interested, < http://courses.ncssm.edu/math/Stat_Inst/Worddocs/DFWalker.doc > seems to be quite good read.
$endgroup$
Intuitively degrees of freedom denotes how many independent things are there. As we introduce constraints, we take away the degree of freedom.
First I'll try to answer your question about Chi-square.
Chi-square distribution with $n$ degree of freedom is the sum of squares $n$ independent standard normal distributions $N(0,1)$ hence we've got $n$ things that vary independently.
I'll start with mechanical example, as degree of freedom is similar in every field.
Consider an airplane flying. It has three degrees of freedom in the usual universe of space, and can be located only if three coordinates are known. These might be latitude, longitude, and altitude; or might be altitude, horizontal distance from some origin, and an angle; or might be direct distance from some origin, and two direction angles. If we consider a given instant of time as a section through the space-time universe, the airplane moves in a four‑dimensional path and can be located by four coordinates, the three previously named and a time coordinate.Hence it now has $4$ d.f.
Note that we assumed that plane is not rotating.
Now considering statistical degrees of freedom..
Similar meaning.
Degree of freedom of a statistic is number of values in calculation of statistic that are independent to vary.As we add restriction to observations, we reduce the degree of freedom.Imposing a relationship upon the observations is equivalent to estimating a parameter from them. The number of degrees of freedom is equal to the number of independent observations, which is the number of original observations minus the number of parmeters estimated from them.
Consider the calculation of mean $frac sum_i=1^n X_n n$, we are interested in estimation of error which are estimated by residues. Sum of residuals is $0$. Knowledge of any $n-1$ residues gives the remaining residue. So, only $n-1$ can vary independently. Hence they have $n-1$ d.f.
However d.f is mainly used in regression analysis, and ANOVA. You may note that all the distributions with so called d.f correspond to particular cases in linear statistics. Hence d.f is at the best artificial as they are not constraints on the random variable, but are actually degree of freedom of some quantities in some application from where these distributions originated.
Also, For people who are interested, < http://courses.ncssm.edu/math/Stat_Inst/Worddocs/DFWalker.doc > seems to be quite good read.
edited Nov 15 '12 at 12:00
answered Nov 15 '12 at 11:38
TheJokerTheJoker
1,694714
1,694714
$begingroup$
Thanks. I have gave you an upvote. Another question here: What's the difference between degree of freedom and dimension in your mechanical example? : )
$endgroup$
– John Hass
Nov 16 '12 at 11:00
1
$begingroup$
Degree of freedom for a prticle is the number of dimensions only if the motion is not constrained. For an example, consider a particle hanging down from ceiling with the help of a rigid rod, then degree of freedom is less than $3$, right?(it is actually $2$.). Any two independent parameters give all information about the position of particle. (Try to give position using two parameters). If you still have questions, ask. I'll be glad to help if I can..
$endgroup$
– TheJoker
Nov 16 '12 at 16:42
add a comment |
$begingroup$
Thanks. I have gave you an upvote. Another question here: What's the difference between degree of freedom and dimension in your mechanical example? : )
$endgroup$
– John Hass
Nov 16 '12 at 11:00
1
$begingroup$
Degree of freedom for a prticle is the number of dimensions only if the motion is not constrained. For an example, consider a particle hanging down from ceiling with the help of a rigid rod, then degree of freedom is less than $3$, right?(it is actually $2$.). Any two independent parameters give all information about the position of particle. (Try to give position using two parameters). If you still have questions, ask. I'll be glad to help if I can..
$endgroup$
– TheJoker
Nov 16 '12 at 16:42
$begingroup$
Thanks. I have gave you an upvote. Another question here: What's the difference between degree of freedom and dimension in your mechanical example? : )
$endgroup$
– John Hass
Nov 16 '12 at 11:00
$begingroup$
Thanks. I have gave you an upvote. Another question here: What's the difference between degree of freedom and dimension in your mechanical example? : )
$endgroup$
– John Hass
Nov 16 '12 at 11:00
1
1
$begingroup$
Degree of freedom for a prticle is the number of dimensions only if the motion is not constrained. For an example, consider a particle hanging down from ceiling with the help of a rigid rod, then degree of freedom is less than $3$, right?(it is actually $2$.). Any two independent parameters give all information about the position of particle. (Try to give position using two parameters). If you still have questions, ask. I'll be glad to help if I can..
$endgroup$
– TheJoker
Nov 16 '12 at 16:42
$begingroup$
Degree of freedom for a prticle is the number of dimensions only if the motion is not constrained. For an example, consider a particle hanging down from ceiling with the help of a rigid rod, then degree of freedom is less than $3$, right?(it is actually $2$.). Any two independent parameters give all information about the position of particle. (Try to give position using two parameters). If you still have questions, ask. I'll be glad to help if I can..
$endgroup$
– TheJoker
Nov 16 '12 at 16:42
add a comment |
$begingroup$
Two people are sitting at a bar, you and your friend. There are two sorts of juice before you, one sweet, one sour. After you have chosen your drink, say the sweet one, your friend has no more choice - so degree of freedom is "1": only one of you can choose.
Generalize it to a group of friends to understand higher degrees of freedom...
$endgroup$
add a comment |
$begingroup$
Two people are sitting at a bar, you and your friend. There are two sorts of juice before you, one sweet, one sour. After you have chosen your drink, say the sweet one, your friend has no more choice - so degree of freedom is "1": only one of you can choose.
Generalize it to a group of friends to understand higher degrees of freedom...
$endgroup$
add a comment |
$begingroup$
Two people are sitting at a bar, you and your friend. There are two sorts of juice before you, one sweet, one sour. After you have chosen your drink, say the sweet one, your friend has no more choice - so degree of freedom is "1": only one of you can choose.
Generalize it to a group of friends to understand higher degrees of freedom...
$endgroup$
Two people are sitting at a bar, you and your friend. There are two sorts of juice before you, one sweet, one sour. After you have chosen your drink, say the sweet one, your friend has no more choice - so degree of freedom is "1": only one of you can choose.
Generalize it to a group of friends to understand higher degrees of freedom...
answered Nov 15 '12 at 12:06


Gottfried HelmsGottfried Helms
23.7k245101
23.7k245101
add a comment |
add a comment |
$begingroup$
When you estimate parameters in statistics, suppose you use a parameter (which you have estimated) to estimate another parameter, then you lose $1$ degree of freedom and you end up with $n-1$ degrees of freedom where $n$ is the number of 'individuals' in the population with which you are working.
One example is when working with normal distributions
$$sigma=sqrtfracsum(x-mu)n^2$$
let $s$ be the estimate of the population standard deviation ($sigma$). We use the mean value $bar x$ instead of $mu$.
$$s=sqrtfracsum(x-bar x)n-1^2$$
Here $bar x$ is an estimate of $mu$. We estimate $sigma$ by using an estimate of $mu$. Hence we lose $1$ of degree of freedom, resulting in $n-1$ degrees of freedom.
$endgroup$
add a comment |
$begingroup$
When you estimate parameters in statistics, suppose you use a parameter (which you have estimated) to estimate another parameter, then you lose $1$ degree of freedom and you end up with $n-1$ degrees of freedom where $n$ is the number of 'individuals' in the population with which you are working.
One example is when working with normal distributions
$$sigma=sqrtfracsum(x-mu)n^2$$
let $s$ be the estimate of the population standard deviation ($sigma$). We use the mean value $bar x$ instead of $mu$.
$$s=sqrtfracsum(x-bar x)n-1^2$$
Here $bar x$ is an estimate of $mu$. We estimate $sigma$ by using an estimate of $mu$. Hence we lose $1$ of degree of freedom, resulting in $n-1$ degrees of freedom.
$endgroup$
add a comment |
$begingroup$
When you estimate parameters in statistics, suppose you use a parameter (which you have estimated) to estimate another parameter, then you lose $1$ degree of freedom and you end up with $n-1$ degrees of freedom where $n$ is the number of 'individuals' in the population with which you are working.
One example is when working with normal distributions
$$sigma=sqrtfracsum(x-mu)n^2$$
let $s$ be the estimate of the population standard deviation ($sigma$). We use the mean value $bar x$ instead of $mu$.
$$s=sqrtfracsum(x-bar x)n-1^2$$
Here $bar x$ is an estimate of $mu$. We estimate $sigma$ by using an estimate of $mu$. Hence we lose $1$ of degree of freedom, resulting in $n-1$ degrees of freedom.
$endgroup$
When you estimate parameters in statistics, suppose you use a parameter (which you have estimated) to estimate another parameter, then you lose $1$ degree of freedom and you end up with $n-1$ degrees of freedom where $n$ is the number of 'individuals' in the population with which you are working.
One example is when working with normal distributions
$$sigma=sqrtfracsum(x-mu)n^2$$
let $s$ be the estimate of the population standard deviation ($sigma$). We use the mean value $bar x$ instead of $mu$.
$$s=sqrtfracsum(x-bar x)n-1^2$$
Here $bar x$ is an estimate of $mu$. We estimate $sigma$ by using an estimate of $mu$. Hence we lose $1$ of degree of freedom, resulting in $n-1$ degrees of freedom.
answered Nov 15 '12 at 9:51
Bhavish SuarezBhavish Suarez
504415
504415
add a comment |
add a comment |
$begingroup$
I've always viewed "degrees of freedom" as a measure of the number of choices that are available to you in a situation. This of course is not meant to be rigorous, but works intuitively in examples.
Consider the problem of choosing a polynomial of degree $2$ (with coefficients in some fixed field $k$). Then we have three choices to make, corresponding to the selection of $a_0,a_1,a_2$ in
$$f(x):=a_2x^2+a_1x+a_0.$$
We should then expect the number of degrees of freedom in this example to be three. And indeed, the space of such polynomials is a vector space, of degree $3$. If we suppose now that we only care for polynomials admitting $x=1$ as a root, we have -- on an intuitive level -- used up one of our degrees of freedom, and we should expect to be able to make two more independent choices. This is indeed the case: we are left with a vector space of dimension $2$.
Note that these notions only really make sense for vector spaces and "choices" that correspond to the intersection of hyperplanes. This all can be generalized within the framework of algebraic geometry (and intersection theory), but matters become complicated when hypersurfaces need not intersect as expected.
$endgroup$
add a comment |
$begingroup$
I've always viewed "degrees of freedom" as a measure of the number of choices that are available to you in a situation. This of course is not meant to be rigorous, but works intuitively in examples.
Consider the problem of choosing a polynomial of degree $2$ (with coefficients in some fixed field $k$). Then we have three choices to make, corresponding to the selection of $a_0,a_1,a_2$ in
$$f(x):=a_2x^2+a_1x+a_0.$$
We should then expect the number of degrees of freedom in this example to be three. And indeed, the space of such polynomials is a vector space, of degree $3$. If we suppose now that we only care for polynomials admitting $x=1$ as a root, we have -- on an intuitive level -- used up one of our degrees of freedom, and we should expect to be able to make two more independent choices. This is indeed the case: we are left with a vector space of dimension $2$.
Note that these notions only really make sense for vector spaces and "choices" that correspond to the intersection of hyperplanes. This all can be generalized within the framework of algebraic geometry (and intersection theory), but matters become complicated when hypersurfaces need not intersect as expected.
$endgroup$
add a comment |
$begingroup$
I've always viewed "degrees of freedom" as a measure of the number of choices that are available to you in a situation. This of course is not meant to be rigorous, but works intuitively in examples.
Consider the problem of choosing a polynomial of degree $2$ (with coefficients in some fixed field $k$). Then we have three choices to make, corresponding to the selection of $a_0,a_1,a_2$ in
$$f(x):=a_2x^2+a_1x+a_0.$$
We should then expect the number of degrees of freedom in this example to be three. And indeed, the space of such polynomials is a vector space, of degree $3$. If we suppose now that we only care for polynomials admitting $x=1$ as a root, we have -- on an intuitive level -- used up one of our degrees of freedom, and we should expect to be able to make two more independent choices. This is indeed the case: we are left with a vector space of dimension $2$.
Note that these notions only really make sense for vector spaces and "choices" that correspond to the intersection of hyperplanes. This all can be generalized within the framework of algebraic geometry (and intersection theory), but matters become complicated when hypersurfaces need not intersect as expected.
$endgroup$
I've always viewed "degrees of freedom" as a measure of the number of choices that are available to you in a situation. This of course is not meant to be rigorous, but works intuitively in examples.
Consider the problem of choosing a polynomial of degree $2$ (with coefficients in some fixed field $k$). Then we have three choices to make, corresponding to the selection of $a_0,a_1,a_2$ in
$$f(x):=a_2x^2+a_1x+a_0.$$
We should then expect the number of degrees of freedom in this example to be three. And indeed, the space of such polynomials is a vector space, of degree $3$. If we suppose now that we only care for polynomials admitting $x=1$ as a root, we have -- on an intuitive level -- used up one of our degrees of freedom, and we should expect to be able to make two more independent choices. This is indeed the case: we are left with a vector space of dimension $2$.
Note that these notions only really make sense for vector spaces and "choices" that correspond to the intersection of hyperplanes. This all can be generalized within the framework of algebraic geometry (and intersection theory), but matters become complicated when hypersurfaces need not intersect as expected.
answered Nov 15 '12 at 7:17


awwalkerawwalker
5,40911728
5,40911728
add a comment |
add a comment |
$begingroup$
To understand the concept intuitively, ask the question of why it is needed. And start from a simpler question, why is the variance computed as $V = frac 1 N-1 sum (x_i-bar x)^2$ with a $N-1$ as denominator.
The fact is that you are interested in the variance of the population, not of the one of sample. Now obviously the sample is less dispersed than the population (because it is very likely that your sample missed a few of the extreme values), so the variance computed on the sample is lower than the variance of the population.
You have to correct the bias.
Intuitively, the observed average $bar x = frac 1 N sum x_i$ is not exact but only an approximation of the population mean. The variance of this approximation should be added to the observed variance on the sample in order to to get the best approximate of the population sample.
Now this variance can be computed: $sigma^2(bar X) = frac 1 N sigma^2(X)$ (using the iid if the $X_i$). So the sample variance is $1 -frac 1 N$ the variance of the population.
Formally (in case you need to read twice the previous reasoning), compute the expected value of $sum (X_i-bar X)^2$. You will find $(N-1) sigma^2$ rather than $N sigma^2$, hence the population variance is $frac N N-1$ the sample variance (as claimed).
When you follow the computations, you start by replacing $bar X$ by its definition $bar X = frac 1 N sum X_i$, develop the squares, expand the sum, and then one of the term disappears. Namely $Nbar X=sum X_i$ appears twice with opposite sign, negative in the double product $2 bar X X$ and positive in the square $bar X^2$. So $sum (X_i-bar X)^2$ is the sum of $N-1$ terms equal in expectation.
This is because the $X_i$ are not independent but linked by one relation $sum X_i=N bar X$. In general, if you know the $X_i$ are linked by $p$ independent linear relations $f_j$, then you can cancel out $p$ terms out of the sum $sum (X_i-f_j(X_i))^2$. Hence the unbiased estimator, $sum (X_i-f_j(X_i))^2 approx frac N N-p sum (x_i-f_j(x_i))^2$.
In regression, ANOVA, etc, the independents relations are not so independent because it is often supposed that the sum of the independents variables (causes) have the same average than the dependent variable (effect). $sum a_i bar X_i = bar Y$. Hence the degree of freedom $N-1$, $p-1$ and $N-p$ and unbiasing factors $frac N N-1$, $frac p p-1$ and $frac N N-p$ for $SS_Total$, $SS_Model$ and $SS_Error$ respectively.
In two words, the degree of freedom is the number of independent relationships linking a set of variables, taking into account the variable introduced for intermediary estimators.
$endgroup$
add a comment |
$begingroup$
To understand the concept intuitively, ask the question of why it is needed. And start from a simpler question, why is the variance computed as $V = frac 1 N-1 sum (x_i-bar x)^2$ with a $N-1$ as denominator.
The fact is that you are interested in the variance of the population, not of the one of sample. Now obviously the sample is less dispersed than the population (because it is very likely that your sample missed a few of the extreme values), so the variance computed on the sample is lower than the variance of the population.
You have to correct the bias.
Intuitively, the observed average $bar x = frac 1 N sum x_i$ is not exact but only an approximation of the population mean. The variance of this approximation should be added to the observed variance on the sample in order to to get the best approximate of the population sample.
Now this variance can be computed: $sigma^2(bar X) = frac 1 N sigma^2(X)$ (using the iid if the $X_i$). So the sample variance is $1 -frac 1 N$ the variance of the population.
Formally (in case you need to read twice the previous reasoning), compute the expected value of $sum (X_i-bar X)^2$. You will find $(N-1) sigma^2$ rather than $N sigma^2$, hence the population variance is $frac N N-1$ the sample variance (as claimed).
When you follow the computations, you start by replacing $bar X$ by its definition $bar X = frac 1 N sum X_i$, develop the squares, expand the sum, and then one of the term disappears. Namely $Nbar X=sum X_i$ appears twice with opposite sign, negative in the double product $2 bar X X$ and positive in the square $bar X^2$. So $sum (X_i-bar X)^2$ is the sum of $N-1$ terms equal in expectation.
This is because the $X_i$ are not independent but linked by one relation $sum X_i=N bar X$. In general, if you know the $X_i$ are linked by $p$ independent linear relations $f_j$, then you can cancel out $p$ terms out of the sum $sum (X_i-f_j(X_i))^2$. Hence the unbiased estimator, $sum (X_i-f_j(X_i))^2 approx frac N N-p sum (x_i-f_j(x_i))^2$.
In regression, ANOVA, etc, the independents relations are not so independent because it is often supposed that the sum of the independents variables (causes) have the same average than the dependent variable (effect). $sum a_i bar X_i = bar Y$. Hence the degree of freedom $N-1$, $p-1$ and $N-p$ and unbiasing factors $frac N N-1$, $frac p p-1$ and $frac N N-p$ for $SS_Total$, $SS_Model$ and $SS_Error$ respectively.
In two words, the degree of freedom is the number of independent relationships linking a set of variables, taking into account the variable introduced for intermediary estimators.
$endgroup$
add a comment |
$begingroup$
To understand the concept intuitively, ask the question of why it is needed. And start from a simpler question, why is the variance computed as $V = frac 1 N-1 sum (x_i-bar x)^2$ with a $N-1$ as denominator.
The fact is that you are interested in the variance of the population, not of the one of sample. Now obviously the sample is less dispersed than the population (because it is very likely that your sample missed a few of the extreme values), so the variance computed on the sample is lower than the variance of the population.
You have to correct the bias.
Intuitively, the observed average $bar x = frac 1 N sum x_i$ is not exact but only an approximation of the population mean. The variance of this approximation should be added to the observed variance on the sample in order to to get the best approximate of the population sample.
Now this variance can be computed: $sigma^2(bar X) = frac 1 N sigma^2(X)$ (using the iid if the $X_i$). So the sample variance is $1 -frac 1 N$ the variance of the population.
Formally (in case you need to read twice the previous reasoning), compute the expected value of $sum (X_i-bar X)^2$. You will find $(N-1) sigma^2$ rather than $N sigma^2$, hence the population variance is $frac N N-1$ the sample variance (as claimed).
When you follow the computations, you start by replacing $bar X$ by its definition $bar X = frac 1 N sum X_i$, develop the squares, expand the sum, and then one of the term disappears. Namely $Nbar X=sum X_i$ appears twice with opposite sign, negative in the double product $2 bar X X$ and positive in the square $bar X^2$. So $sum (X_i-bar X)^2$ is the sum of $N-1$ terms equal in expectation.
This is because the $X_i$ are not independent but linked by one relation $sum X_i=N bar X$. In general, if you know the $X_i$ are linked by $p$ independent linear relations $f_j$, then you can cancel out $p$ terms out of the sum $sum (X_i-f_j(X_i))^2$. Hence the unbiased estimator, $sum (X_i-f_j(X_i))^2 approx frac N N-p sum (x_i-f_j(x_i))^2$.
In regression, ANOVA, etc, the independents relations are not so independent because it is often supposed that the sum of the independents variables (causes) have the same average than the dependent variable (effect). $sum a_i bar X_i = bar Y$. Hence the degree of freedom $N-1$, $p-1$ and $N-p$ and unbiasing factors $frac N N-1$, $frac p p-1$ and $frac N N-p$ for $SS_Total$, $SS_Model$ and $SS_Error$ respectively.
In two words, the degree of freedom is the number of independent relationships linking a set of variables, taking into account the variable introduced for intermediary estimators.
$endgroup$
To understand the concept intuitively, ask the question of why it is needed. And start from a simpler question, why is the variance computed as $V = frac 1 N-1 sum (x_i-bar x)^2$ with a $N-1$ as denominator.
The fact is that you are interested in the variance of the population, not of the one of sample. Now obviously the sample is less dispersed than the population (because it is very likely that your sample missed a few of the extreme values), so the variance computed on the sample is lower than the variance of the population.
You have to correct the bias.
Intuitively, the observed average $bar x = frac 1 N sum x_i$ is not exact but only an approximation of the population mean. The variance of this approximation should be added to the observed variance on the sample in order to to get the best approximate of the population sample.
Now this variance can be computed: $sigma^2(bar X) = frac 1 N sigma^2(X)$ (using the iid if the $X_i$). So the sample variance is $1 -frac 1 N$ the variance of the population.
Formally (in case you need to read twice the previous reasoning), compute the expected value of $sum (X_i-bar X)^2$. You will find $(N-1) sigma^2$ rather than $N sigma^2$, hence the population variance is $frac N N-1$ the sample variance (as claimed).
When you follow the computations, you start by replacing $bar X$ by its definition $bar X = frac 1 N sum X_i$, develop the squares, expand the sum, and then one of the term disappears. Namely $Nbar X=sum X_i$ appears twice with opposite sign, negative in the double product $2 bar X X$ and positive in the square $bar X^2$. So $sum (X_i-bar X)^2$ is the sum of $N-1$ terms equal in expectation.
This is because the $X_i$ are not independent but linked by one relation $sum X_i=N bar X$. In general, if you know the $X_i$ are linked by $p$ independent linear relations $f_j$, then you can cancel out $p$ terms out of the sum $sum (X_i-f_j(X_i))^2$. Hence the unbiased estimator, $sum (X_i-f_j(X_i))^2 approx frac N N-p sum (x_i-f_j(x_i))^2$.
In regression, ANOVA, etc, the independents relations are not so independent because it is often supposed that the sum of the independents variables (causes) have the same average than the dependent variable (effect). $sum a_i bar X_i = bar Y$. Hence the degree of freedom $N-1$, $p-1$ and $N-p$ and unbiasing factors $frac N N-1$, $frac p p-1$ and $frac N N-p$ for $SS_Total$, $SS_Model$ and $SS_Error$ respectively.
In two words, the degree of freedom is the number of independent relationships linking a set of variables, taking into account the variable introduced for intermediary estimators.
answered May 24 '13 at 10:27
AlainDAlainD
65636
65636
add a comment |
add a comment |
Thanks for contributing an answer to Mathematics Stack Exchange!
- Please be sure to answer the question. Provide details and share your research!
But avoid …
- Asking for help, clarification, or responding to other answers.
- Making statements based on opinion; back them up with references or personal experience.
Use MathJax to format equations. MathJax reference.
To learn more, see our tips on writing great answers.
Sign up or log in
StackExchange.ready(function ()
StackExchange.helpers.onClickDraftSave('#login-link');
);
Sign up using Google
Sign up using Facebook
Sign up using Email and Password
Post as a guest
Required, but never shown
StackExchange.ready(
function ()
StackExchange.openid.initPostLogin('.new-post-login', 'https%3a%2f%2fmath.stackexchange.com%2fquestions%2f237790%2fwhat-is-degree-of-freedom-in-statistics%23new-answer', 'question_page');
);
Post as a guest
Required, but never shown
Sign up or log in
StackExchange.ready(function ()
StackExchange.helpers.onClickDraftSave('#login-link');
);
Sign up using Google
Sign up using Facebook
Sign up using Email and Password
Post as a guest
Required, but never shown
Sign up or log in
StackExchange.ready(function ()
StackExchange.helpers.onClickDraftSave('#login-link');
);
Sign up using Google
Sign up using Facebook
Sign up using Email and Password
Post as a guest
Required, but never shown
Sign up or log in
StackExchange.ready(function ()
StackExchange.helpers.onClickDraftSave('#login-link');
);
Sign up using Google
Sign up using Facebook
Sign up using Email and Password
Sign up using Google
Sign up using Facebook
Sign up using Email and Password
Post as a guest
Required, but never shown
Required, but never shown
Required, but never shown
Required, but never shown
Required, but never shown
Required, but never shown
Required, but never shown
Required, but never shown
Required, but never shown
n6,v,flIa,U,v1exhSyWUkNBR40AnK,d7jgwVk7tM,U,70U8QzZ0i3EmVcW
$begingroup$
On CV:stats.stackexchange.com/questions/16921/….
$endgroup$
– StubbornAtom
Mar 22 '18 at 21:07