Numerical Implementation: Solution for the Euler Lagrange Equation Of the Rudin Osher Fatemi (ROF) Total Variation Denoising Model Announcing the arrival of Valued Associate #679: Cesar Manara Planned maintenance scheduled April 17/18, 2019 at 00:00UTC (8:00pm US/Eastern)The Dual Norm of Total Variation Norm (Form of $ left langle cdot, cdot right rangle $) By SmoothingHigh Dimensional Optimization Algorithm?Euler-Lagrange, Gradient Descent, Heat Equation and Image DenoisingEuler lagrange assumptionsTime bound for gradient descentWhat's the difference between conjugate gradient and scale congjugate gradient algorithm?Unconstrained optimization algorithmFind the very first feasible solution (critical point nearest to initial point in constrained optimization)why Clipped gradient can get stuck in a flat spotImproving the convergence of gradient descent methodIntuition or interpretation of the first term of the Mumford Shah functional in image processing
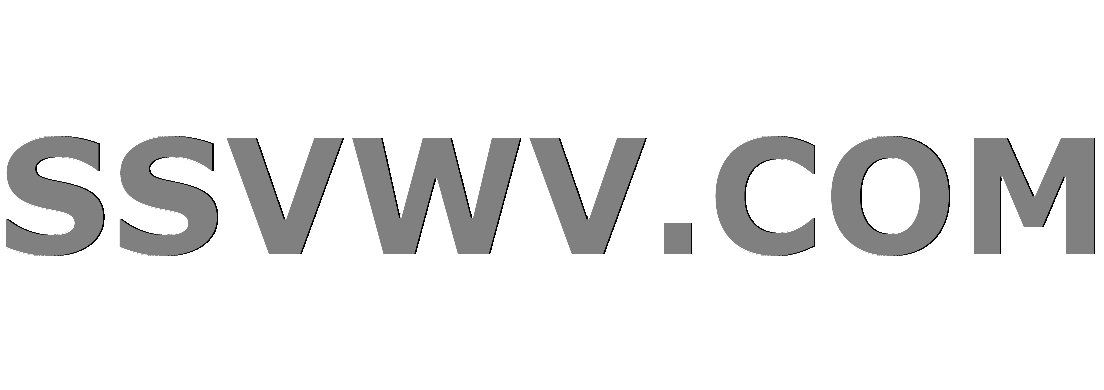
Multi tool use
Is CEO the profession with the most psychopaths?
Do wooden building fires get hotter than 600°C?
What do you call the main part of a joke?
How to Make a Beautiful Stacked 3D Plot
Generate an RGB colour grid
Can a new player join a group only when a new campaign starts?
Has negative voting ever been officially implemented in elections, or seriously proposed, or even studied?
Would "destroying" Wurmcoil Engine prevent its tokens from being created?
Does classifying an integer as a discrete log require it be part of a multiplicative group?
Do I really need to have a message in a novel to appeal to readers?
Why are there no cargo aircraft with "flying wing" design?
How to react to hostile behavior from a senior developer?
How come Sam didn't become Lord of Horn Hill?
An adverb for when you're not exaggerating
Is "Reachable Object" really an NP-complete problem?
Why are both D and D# fitting into my E minor key?
Why didn't Eitri join the fight?
How to deal with a team lead who never gives me credit?
What does this Jacques Hadamard quote mean?
Is there a kind of relay only consumes power when switching?
Is it common practice to audition new musicians one-on-one before rehearsing with the entire band?
Wu formula for manifolds with boundary
Crossing US/Canada Border for less than 24 hours
Fantasy story; one type of magic grows in power with use, but the more powerful they are, they more they are drawn to travel to their source
Numerical Implementation: Solution for the Euler Lagrange Equation Of the Rudin Osher Fatemi (ROF) Total Variation Denoising Model
Announcing the arrival of Valued Associate #679: Cesar Manara
Planned maintenance scheduled April 17/18, 2019 at 00:00UTC (8:00pm US/Eastern)The Dual Norm of Total Variation Norm (Form of $ left langle cdot, cdot right rangle $) By SmoothingHigh Dimensional Optimization Algorithm?Euler-Lagrange, Gradient Descent, Heat Equation and Image DenoisingEuler lagrange assumptionsTime bound for gradient descentWhat's the difference between conjugate gradient and scale congjugate gradient algorithm?Unconstrained optimization algorithmFind the very first feasible solution (critical point nearest to initial point in constrained optimization)why Clipped gradient can get stuck in a flat spotImproving the convergence of gradient descent methodIntuition or interpretation of the first term of the Mumford Shah functional in image processing
$begingroup$
I am watching some wonderful videos on Variational Methods in Image Processing, and the presenter talks about how variational methods are used to de-blur or denoise images, as well as other applications--including "active contours." Variational image processing has been an active research program for some time. One example model the presenter discusses is the Rudin, Osher, Fatemi model for image denoising. The energy function for this minimization problem is given below.
$$
E(u) = int_Omega(u - f)^2 + lambda |nabla u|dx
$$
In the equation, $u$ is a function which generates some data and exhibits some properties as per the first and second terms. The presenter indicated that the Variational problem is minimized using gradient descent methods for optimization.
My question was, does anyone know of a good tutorial on numerically implementing gradient descent on this type of optimization problem? I want to understand exactly how the computer will compute the solution to this problem. Gradient Descent is easy enough to compute, but I was not sure about optimizing over the discretized infinite dimensional space of functions. The functional analysis is not the problem, just understand how the computer algorithm is coded to make this work.
I found an article by Getreuer which talks about the optimization algorithm using the Split Bregman method, which also has some C code. In looking at the code though, it is a bit hard to understand the underlying algorithm versus all of the different function calls and typedefs
, and all.
I was hoping someone might know a good online tutorial or video, etc., that discusses how to implement this type of optimization routine. Thanks.
optimization numerical-methods calculus-of-variations image-processing gradient-descent
$endgroup$
add a comment |
$begingroup$
I am watching some wonderful videos on Variational Methods in Image Processing, and the presenter talks about how variational methods are used to de-blur or denoise images, as well as other applications--including "active contours." Variational image processing has been an active research program for some time. One example model the presenter discusses is the Rudin, Osher, Fatemi model for image denoising. The energy function for this minimization problem is given below.
$$
E(u) = int_Omega(u - f)^2 + lambda |nabla u|dx
$$
In the equation, $u$ is a function which generates some data and exhibits some properties as per the first and second terms. The presenter indicated that the Variational problem is minimized using gradient descent methods for optimization.
My question was, does anyone know of a good tutorial on numerically implementing gradient descent on this type of optimization problem? I want to understand exactly how the computer will compute the solution to this problem. Gradient Descent is easy enough to compute, but I was not sure about optimizing over the discretized infinite dimensional space of functions. The functional analysis is not the problem, just understand how the computer algorithm is coded to make this work.
I found an article by Getreuer which talks about the optimization algorithm using the Split Bregman method, which also has some C code. In looking at the code though, it is a bit hard to understand the underlying algorithm versus all of the different function calls and typedefs
, and all.
I was hoping someone might know a good online tutorial or video, etc., that discusses how to implement this type of optimization routine. Thanks.
optimization numerical-methods calculus-of-variations image-processing gradient-descent
$endgroup$
$begingroup$
Working on answer + code for you.
$endgroup$
– Royi
Mar 27 at 6:40
$begingroup$
@Royi Oh that is so great. Man, I imagine so many people would find your code and explanation helpful. It is so important to find some sort of readable code or explanation of how these types of algorithms work for the sake of reproducibility and validation. Anything that you can provide would be appreciated.
$endgroup$
– krishnab
Mar 27 at 7:39
$begingroup$
I added a code for the solution.
$endgroup$
– Royi
Mar 29 at 23:41
add a comment |
$begingroup$
I am watching some wonderful videos on Variational Methods in Image Processing, and the presenter talks about how variational methods are used to de-blur or denoise images, as well as other applications--including "active contours." Variational image processing has been an active research program for some time. One example model the presenter discusses is the Rudin, Osher, Fatemi model for image denoising. The energy function for this minimization problem is given below.
$$
E(u) = int_Omega(u - f)^2 + lambda |nabla u|dx
$$
In the equation, $u$ is a function which generates some data and exhibits some properties as per the first and second terms. The presenter indicated that the Variational problem is minimized using gradient descent methods for optimization.
My question was, does anyone know of a good tutorial on numerically implementing gradient descent on this type of optimization problem? I want to understand exactly how the computer will compute the solution to this problem. Gradient Descent is easy enough to compute, but I was not sure about optimizing over the discretized infinite dimensional space of functions. The functional analysis is not the problem, just understand how the computer algorithm is coded to make this work.
I found an article by Getreuer which talks about the optimization algorithm using the Split Bregman method, which also has some C code. In looking at the code though, it is a bit hard to understand the underlying algorithm versus all of the different function calls and typedefs
, and all.
I was hoping someone might know a good online tutorial or video, etc., that discusses how to implement this type of optimization routine. Thanks.
optimization numerical-methods calculus-of-variations image-processing gradient-descent
$endgroup$
I am watching some wonderful videos on Variational Methods in Image Processing, and the presenter talks about how variational methods are used to de-blur or denoise images, as well as other applications--including "active contours." Variational image processing has been an active research program for some time. One example model the presenter discusses is the Rudin, Osher, Fatemi model for image denoising. The energy function for this minimization problem is given below.
$$
E(u) = int_Omega(u - f)^2 + lambda |nabla u|dx
$$
In the equation, $u$ is a function which generates some data and exhibits some properties as per the first and second terms. The presenter indicated that the Variational problem is minimized using gradient descent methods for optimization.
My question was, does anyone know of a good tutorial on numerically implementing gradient descent on this type of optimization problem? I want to understand exactly how the computer will compute the solution to this problem. Gradient Descent is easy enough to compute, but I was not sure about optimizing over the discretized infinite dimensional space of functions. The functional analysis is not the problem, just understand how the computer algorithm is coded to make this work.
I found an article by Getreuer which talks about the optimization algorithm using the Split Bregman method, which also has some C code. In looking at the code though, it is a bit hard to understand the underlying algorithm versus all of the different function calls and typedefs
, and all.
I was hoping someone might know a good online tutorial or video, etc., that discusses how to implement this type of optimization routine. Thanks.
optimization numerical-methods calculus-of-variations image-processing gradient-descent
optimization numerical-methods calculus-of-variations image-processing gradient-descent
edited Mar 28 at 5:57
Royi
3,66512454
3,66512454
asked Mar 27 at 6:28


krishnabkrishnab
464415
464415
$begingroup$
Working on answer + code for you.
$endgroup$
– Royi
Mar 27 at 6:40
$begingroup$
@Royi Oh that is so great. Man, I imagine so many people would find your code and explanation helpful. It is so important to find some sort of readable code or explanation of how these types of algorithms work for the sake of reproducibility and validation. Anything that you can provide would be appreciated.
$endgroup$
– krishnab
Mar 27 at 7:39
$begingroup$
I added a code for the solution.
$endgroup$
– Royi
Mar 29 at 23:41
add a comment |
$begingroup$
Working on answer + code for you.
$endgroup$
– Royi
Mar 27 at 6:40
$begingroup$
@Royi Oh that is so great. Man, I imagine so many people would find your code and explanation helpful. It is so important to find some sort of readable code or explanation of how these types of algorithms work for the sake of reproducibility and validation. Anything that you can provide would be appreciated.
$endgroup$
– krishnab
Mar 27 at 7:39
$begingroup$
I added a code for the solution.
$endgroup$
– Royi
Mar 29 at 23:41
$begingroup$
Working on answer + code for you.
$endgroup$
– Royi
Mar 27 at 6:40
$begingroup$
Working on answer + code for you.
$endgroup$
– Royi
Mar 27 at 6:40
$begingroup$
@Royi Oh that is so great. Man, I imagine so many people would find your code and explanation helpful. It is so important to find some sort of readable code or explanation of how these types of algorithms work for the sake of reproducibility and validation. Anything that you can provide would be appreciated.
$endgroup$
– krishnab
Mar 27 at 7:39
$begingroup$
@Royi Oh that is so great. Man, I imagine so many people would find your code and explanation helpful. It is so important to find some sort of readable code or explanation of how these types of algorithms work for the sake of reproducibility and validation. Anything that you can provide would be appreciated.
$endgroup$
– krishnab
Mar 27 at 7:39
$begingroup$
I added a code for the solution.
$endgroup$
– Royi
Mar 29 at 23:41
$begingroup$
I added a code for the solution.
$endgroup$
– Royi
Mar 29 at 23:41
add a comment |
1 Answer
1
active
oldest
votes
$begingroup$
The basic idea is to right the problem in a discrete manner.
Let's start with a 1D example.
Imagine the discrete realization of $ f $ is given by the vector $ boldsymbolf in mathbbR^n $.
The problem becomes:
$$ hat boldsymbolu = arg min_ boldsymbolu frac12 boldsymbolu - boldsymbolf right_2^2 + lambda left| D boldsymbolu right|_1 $$
Where $ D $ is finite differences operator in Matrix form such that:
$$ left| D boldsymbolu right|_1 = sum_i = 2^n left| boldsymbolu_i - boldsymbolu_i - 1 right| $$
This is basically Linear Least Squares with Total Variation Regularization.
There are may methods to solve this, for instance, see The Dual Norm of Total Variation Norm (Form of ⟨⋅,⋅⟩) By Smoothing.
Modern way of solving this is using the Alternating Direction Method of Multipliers (ADMM) with great resource on this subject would be Professor Stephen P. Boyd page on ADMM.
Assume the solution to that is given by a Black Box - 1D TV Denoising.
I will point out more than that, let's assume our solution can solve the problem above for any matrix $ D $ (Not specifically Forward / Backward Differences operator).
We'll get to a specific solution and code for it later, but let's assume we have it.
We have in image in 2D space but we could vectorize it into a column stack form using the Vectorization Operator.
It is easy to see the Fidelity Term (The $ L_2 $ term) in our optimization works well. But we have to deal with the 2nd term - The TV Operator.
The TV operator could be defined in (At least?) 2 ways in 2D.
The 2 form of the TV Operators in 2D is $ mathbfTV left( X right) = mathbbR^m times n to mathbbR $
- 2D Anistoropic Total Variation
$$ mathbfTV_I left( X right) = sum_i = 1^m - 1 sum_j = 1^n - 1 sqrt left( X_i, j - X_i + 1, j right)^2 + left( X_i, j - X_i, j + 1 right)^2 + sum_i = 1^m - 1 left| X_i, n - X_i + 1, n right| + sum_j = 1^n - 1 left| X_m, j - X_m, j + 1 right| $$ - 2D Isotropic Total Variation
$$ mathbfTV_A left( X right) = sum_i = 1^m - 1 sum_j = 1^m left| X_i, j - X_i + 1, j right| + sum_i = 1^m sum_j = 1^n - 1 left| X_i, j - X_i, j + 1 right| $$
Remark
One could come up with many more variations. For instance, include the diagonals as well.
I will ignore the Isotropic version for this case (Though it is not hard to solve it as well).
The Anisotropic Case is very similar to the 1D case with on difference, we need to create 2 subtractions for each pixel.
So with some tweaking of the matrix we can write is in the same model of the 1D case.
We just need to update $ D $ to be $ D : mathbbR^m n to mathbbR^2 m n - m - n $.
So we need a code to build the matrix $ D $ in the 2D case and the solver to the 1D case and we're done.
2D Gradient Operator
Work in Progress
Results
So, the implemented code yields as following (The reference being CVX based solution):
As can be seen above, the numerical solver can achieve the optimal solution.
The code is available at my StackExchange Mathematics Q3164164 GitHub Repository.
Black Box Solver
Relatively simple yet effective method is An Algorithm for Total Variation Minimization and Applications.
The idea is the use of Dual Norm / Support Function which suggests that:
$$ _1 = max_boldsymbolp left boldsymbolp^T D boldsymbolx mid boldsymbolp right_infty leq 1 right $$
Hence our problem becomes:
$$ arg min_ boldsymbolu frac12 boldsymbolu - boldsymbolf right_2^2 + lambda left| D boldsymbolu right|_1 = arg min_ boldsymbolu max_ left_infty leq 1 frac12 boldsymbolu - boldsymbolf right_2^2 + lambda boldsymbolp^T D boldsymbolu $$
Using the Min Max Theorem (The objective is Convex with respect to $ boldsymbolu $ and Concave with respect to $ boldsymbolp $) one could switch the order of the Maximum and Minimum which yields:
$$ arg max_ left_infty leq 1 min_ boldsymbolu frac12 boldsymbolu - boldsymbolf right_2^2 + lambda boldsymbolp^T D boldsymbolu $$
Which can be solved pretty easily with Projected Gradient Descent.
Remarks
- The solver in its FISTA (A Fast Iterative Shrinkage Thresholding Algorithm for Linear Inverse Problems) form is much faster with a simple tweak.
- The code use matrix form for simplicity. In practice all operation should be done using Convolution in the case of the TV.
$endgroup$
$begingroup$
Wow, this is amazing. Thank you so much for your effort. I keep seeing this TV norm minimization everywhere, but did not have a sense of the implementation. This is really really helpful. Hopefully you get a lot of good karma :).
$endgroup$
– krishnab
Mar 29 at 23:49
add a comment |
Your Answer
StackExchange.ready(function()
var channelOptions =
tags: "".split(" "),
id: "69"
;
initTagRenderer("".split(" "), "".split(" "), channelOptions);
StackExchange.using("externalEditor", function()
// Have to fire editor after snippets, if snippets enabled
if (StackExchange.settings.snippets.snippetsEnabled)
StackExchange.using("snippets", function()
createEditor();
);
else
createEditor();
);
function createEditor()
StackExchange.prepareEditor(
heartbeatType: 'answer',
autoActivateHeartbeat: false,
convertImagesToLinks: true,
noModals: true,
showLowRepImageUploadWarning: true,
reputationToPostImages: 10,
bindNavPrevention: true,
postfix: "",
imageUploader:
brandingHtml: "Powered by u003ca class="icon-imgur-white" href="https://imgur.com/"u003eu003c/au003e",
contentPolicyHtml: "User contributions licensed under u003ca href="https://creativecommons.org/licenses/by-sa/3.0/"u003ecc by-sa 3.0 with attribution requiredu003c/au003e u003ca href="https://stackoverflow.com/legal/content-policy"u003e(content policy)u003c/au003e",
allowUrls: true
,
noCode: true, onDemand: true,
discardSelector: ".discard-answer"
,immediatelyShowMarkdownHelp:true
);
);
Sign up or log in
StackExchange.ready(function ()
StackExchange.helpers.onClickDraftSave('#login-link');
);
Sign up using Google
Sign up using Facebook
Sign up using Email and Password
Post as a guest
Required, but never shown
StackExchange.ready(
function ()
StackExchange.openid.initPostLogin('.new-post-login', 'https%3a%2f%2fmath.stackexchange.com%2fquestions%2f3164164%2fnumerical-implementation-solution-for-the-euler-lagrange-equation-of-the-rudin%23new-answer', 'question_page');
);
Post as a guest
Required, but never shown
1 Answer
1
active
oldest
votes
1 Answer
1
active
oldest
votes
active
oldest
votes
active
oldest
votes
$begingroup$
The basic idea is to right the problem in a discrete manner.
Let's start with a 1D example.
Imagine the discrete realization of $ f $ is given by the vector $ boldsymbolf in mathbbR^n $.
The problem becomes:
$$ hat boldsymbolu = arg min_ boldsymbolu frac12 boldsymbolu - boldsymbolf right_2^2 + lambda left| D boldsymbolu right|_1 $$
Where $ D $ is finite differences operator in Matrix form such that:
$$ left| D boldsymbolu right|_1 = sum_i = 2^n left| boldsymbolu_i - boldsymbolu_i - 1 right| $$
This is basically Linear Least Squares with Total Variation Regularization.
There are may methods to solve this, for instance, see The Dual Norm of Total Variation Norm (Form of ⟨⋅,⋅⟩) By Smoothing.
Modern way of solving this is using the Alternating Direction Method of Multipliers (ADMM) with great resource on this subject would be Professor Stephen P. Boyd page on ADMM.
Assume the solution to that is given by a Black Box - 1D TV Denoising.
I will point out more than that, let's assume our solution can solve the problem above for any matrix $ D $ (Not specifically Forward / Backward Differences operator).
We'll get to a specific solution and code for it later, but let's assume we have it.
We have in image in 2D space but we could vectorize it into a column stack form using the Vectorization Operator.
It is easy to see the Fidelity Term (The $ L_2 $ term) in our optimization works well. But we have to deal with the 2nd term - The TV Operator.
The TV operator could be defined in (At least?) 2 ways in 2D.
The 2 form of the TV Operators in 2D is $ mathbfTV left( X right) = mathbbR^m times n to mathbbR $
- 2D Anistoropic Total Variation
$$ mathbfTV_I left( X right) = sum_i = 1^m - 1 sum_j = 1^n - 1 sqrt left( X_i, j - X_i + 1, j right)^2 + left( X_i, j - X_i, j + 1 right)^2 + sum_i = 1^m - 1 left| X_i, n - X_i + 1, n right| + sum_j = 1^n - 1 left| X_m, j - X_m, j + 1 right| $$ - 2D Isotropic Total Variation
$$ mathbfTV_A left( X right) = sum_i = 1^m - 1 sum_j = 1^m left| X_i, j - X_i + 1, j right| + sum_i = 1^m sum_j = 1^n - 1 left| X_i, j - X_i, j + 1 right| $$
Remark
One could come up with many more variations. For instance, include the diagonals as well.
I will ignore the Isotropic version for this case (Though it is not hard to solve it as well).
The Anisotropic Case is very similar to the 1D case with on difference, we need to create 2 subtractions for each pixel.
So with some tweaking of the matrix we can write is in the same model of the 1D case.
We just need to update $ D $ to be $ D : mathbbR^m n to mathbbR^2 m n - m - n $.
So we need a code to build the matrix $ D $ in the 2D case and the solver to the 1D case and we're done.
2D Gradient Operator
Work in Progress
Results
So, the implemented code yields as following (The reference being CVX based solution):
As can be seen above, the numerical solver can achieve the optimal solution.
The code is available at my StackExchange Mathematics Q3164164 GitHub Repository.
Black Box Solver
Relatively simple yet effective method is An Algorithm for Total Variation Minimization and Applications.
The idea is the use of Dual Norm / Support Function which suggests that:
$$ _1 = max_boldsymbolp left boldsymbolp^T D boldsymbolx mid boldsymbolp right_infty leq 1 right $$
Hence our problem becomes:
$$ arg min_ boldsymbolu frac12 boldsymbolu - boldsymbolf right_2^2 + lambda left| D boldsymbolu right|_1 = arg min_ boldsymbolu max_ left_infty leq 1 frac12 boldsymbolu - boldsymbolf right_2^2 + lambda boldsymbolp^T D boldsymbolu $$
Using the Min Max Theorem (The objective is Convex with respect to $ boldsymbolu $ and Concave with respect to $ boldsymbolp $) one could switch the order of the Maximum and Minimum which yields:
$$ arg max_ left_infty leq 1 min_ boldsymbolu frac12 boldsymbolu - boldsymbolf right_2^2 + lambda boldsymbolp^T D boldsymbolu $$
Which can be solved pretty easily with Projected Gradient Descent.
Remarks
- The solver in its FISTA (A Fast Iterative Shrinkage Thresholding Algorithm for Linear Inverse Problems) form is much faster with a simple tweak.
- The code use matrix form for simplicity. In practice all operation should be done using Convolution in the case of the TV.
$endgroup$
$begingroup$
Wow, this is amazing. Thank you so much for your effort. I keep seeing this TV norm minimization everywhere, but did not have a sense of the implementation. This is really really helpful. Hopefully you get a lot of good karma :).
$endgroup$
– krishnab
Mar 29 at 23:49
add a comment |
$begingroup$
The basic idea is to right the problem in a discrete manner.
Let's start with a 1D example.
Imagine the discrete realization of $ f $ is given by the vector $ boldsymbolf in mathbbR^n $.
The problem becomes:
$$ hat boldsymbolu = arg min_ boldsymbolu frac12 boldsymbolu - boldsymbolf right_2^2 + lambda left| D boldsymbolu right|_1 $$
Where $ D $ is finite differences operator in Matrix form such that:
$$ left| D boldsymbolu right|_1 = sum_i = 2^n left| boldsymbolu_i - boldsymbolu_i - 1 right| $$
This is basically Linear Least Squares with Total Variation Regularization.
There are may methods to solve this, for instance, see The Dual Norm of Total Variation Norm (Form of ⟨⋅,⋅⟩) By Smoothing.
Modern way of solving this is using the Alternating Direction Method of Multipliers (ADMM) with great resource on this subject would be Professor Stephen P. Boyd page on ADMM.
Assume the solution to that is given by a Black Box - 1D TV Denoising.
I will point out more than that, let's assume our solution can solve the problem above for any matrix $ D $ (Not specifically Forward / Backward Differences operator).
We'll get to a specific solution and code for it later, but let's assume we have it.
We have in image in 2D space but we could vectorize it into a column stack form using the Vectorization Operator.
It is easy to see the Fidelity Term (The $ L_2 $ term) in our optimization works well. But we have to deal with the 2nd term - The TV Operator.
The TV operator could be defined in (At least?) 2 ways in 2D.
The 2 form of the TV Operators in 2D is $ mathbfTV left( X right) = mathbbR^m times n to mathbbR $
- 2D Anistoropic Total Variation
$$ mathbfTV_I left( X right) = sum_i = 1^m - 1 sum_j = 1^n - 1 sqrt left( X_i, j - X_i + 1, j right)^2 + left( X_i, j - X_i, j + 1 right)^2 + sum_i = 1^m - 1 left| X_i, n - X_i + 1, n right| + sum_j = 1^n - 1 left| X_m, j - X_m, j + 1 right| $$ - 2D Isotropic Total Variation
$$ mathbfTV_A left( X right) = sum_i = 1^m - 1 sum_j = 1^m left| X_i, j - X_i + 1, j right| + sum_i = 1^m sum_j = 1^n - 1 left| X_i, j - X_i, j + 1 right| $$
Remark
One could come up with many more variations. For instance, include the diagonals as well.
I will ignore the Isotropic version for this case (Though it is not hard to solve it as well).
The Anisotropic Case is very similar to the 1D case with on difference, we need to create 2 subtractions for each pixel.
So with some tweaking of the matrix we can write is in the same model of the 1D case.
We just need to update $ D $ to be $ D : mathbbR^m n to mathbbR^2 m n - m - n $.
So we need a code to build the matrix $ D $ in the 2D case and the solver to the 1D case and we're done.
2D Gradient Operator
Work in Progress
Results
So, the implemented code yields as following (The reference being CVX based solution):
As can be seen above, the numerical solver can achieve the optimal solution.
The code is available at my StackExchange Mathematics Q3164164 GitHub Repository.
Black Box Solver
Relatively simple yet effective method is An Algorithm for Total Variation Minimization and Applications.
The idea is the use of Dual Norm / Support Function which suggests that:
$$ _1 = max_boldsymbolp left boldsymbolp^T D boldsymbolx mid boldsymbolp right_infty leq 1 right $$
Hence our problem becomes:
$$ arg min_ boldsymbolu frac12 boldsymbolu - boldsymbolf right_2^2 + lambda left| D boldsymbolu right|_1 = arg min_ boldsymbolu max_ left_infty leq 1 frac12 boldsymbolu - boldsymbolf right_2^2 + lambda boldsymbolp^T D boldsymbolu $$
Using the Min Max Theorem (The objective is Convex with respect to $ boldsymbolu $ and Concave with respect to $ boldsymbolp $) one could switch the order of the Maximum and Minimum which yields:
$$ arg max_ left_infty leq 1 min_ boldsymbolu frac12 boldsymbolu - boldsymbolf right_2^2 + lambda boldsymbolp^T D boldsymbolu $$
Which can be solved pretty easily with Projected Gradient Descent.
Remarks
- The solver in its FISTA (A Fast Iterative Shrinkage Thresholding Algorithm for Linear Inverse Problems) form is much faster with a simple tweak.
- The code use matrix form for simplicity. In practice all operation should be done using Convolution in the case of the TV.
$endgroup$
$begingroup$
Wow, this is amazing. Thank you so much for your effort. I keep seeing this TV norm minimization everywhere, but did not have a sense of the implementation. This is really really helpful. Hopefully you get a lot of good karma :).
$endgroup$
– krishnab
Mar 29 at 23:49
add a comment |
$begingroup$
The basic idea is to right the problem in a discrete manner.
Let's start with a 1D example.
Imagine the discrete realization of $ f $ is given by the vector $ boldsymbolf in mathbbR^n $.
The problem becomes:
$$ hat boldsymbolu = arg min_ boldsymbolu frac12 boldsymbolu - boldsymbolf right_2^2 + lambda left| D boldsymbolu right|_1 $$
Where $ D $ is finite differences operator in Matrix form such that:
$$ left| D boldsymbolu right|_1 = sum_i = 2^n left| boldsymbolu_i - boldsymbolu_i - 1 right| $$
This is basically Linear Least Squares with Total Variation Regularization.
There are may methods to solve this, for instance, see The Dual Norm of Total Variation Norm (Form of ⟨⋅,⋅⟩) By Smoothing.
Modern way of solving this is using the Alternating Direction Method of Multipliers (ADMM) with great resource on this subject would be Professor Stephen P. Boyd page on ADMM.
Assume the solution to that is given by a Black Box - 1D TV Denoising.
I will point out more than that, let's assume our solution can solve the problem above for any matrix $ D $ (Not specifically Forward / Backward Differences operator).
We'll get to a specific solution and code for it later, but let's assume we have it.
We have in image in 2D space but we could vectorize it into a column stack form using the Vectorization Operator.
It is easy to see the Fidelity Term (The $ L_2 $ term) in our optimization works well. But we have to deal with the 2nd term - The TV Operator.
The TV operator could be defined in (At least?) 2 ways in 2D.
The 2 form of the TV Operators in 2D is $ mathbfTV left( X right) = mathbbR^m times n to mathbbR $
- 2D Anistoropic Total Variation
$$ mathbfTV_I left( X right) = sum_i = 1^m - 1 sum_j = 1^n - 1 sqrt left( X_i, j - X_i + 1, j right)^2 + left( X_i, j - X_i, j + 1 right)^2 + sum_i = 1^m - 1 left| X_i, n - X_i + 1, n right| + sum_j = 1^n - 1 left| X_m, j - X_m, j + 1 right| $$ - 2D Isotropic Total Variation
$$ mathbfTV_A left( X right) = sum_i = 1^m - 1 sum_j = 1^m left| X_i, j - X_i + 1, j right| + sum_i = 1^m sum_j = 1^n - 1 left| X_i, j - X_i, j + 1 right| $$
Remark
One could come up with many more variations. For instance, include the diagonals as well.
I will ignore the Isotropic version for this case (Though it is not hard to solve it as well).
The Anisotropic Case is very similar to the 1D case with on difference, we need to create 2 subtractions for each pixel.
So with some tweaking of the matrix we can write is in the same model of the 1D case.
We just need to update $ D $ to be $ D : mathbbR^m n to mathbbR^2 m n - m - n $.
So we need a code to build the matrix $ D $ in the 2D case and the solver to the 1D case and we're done.
2D Gradient Operator
Work in Progress
Results
So, the implemented code yields as following (The reference being CVX based solution):
As can be seen above, the numerical solver can achieve the optimal solution.
The code is available at my StackExchange Mathematics Q3164164 GitHub Repository.
Black Box Solver
Relatively simple yet effective method is An Algorithm for Total Variation Minimization and Applications.
The idea is the use of Dual Norm / Support Function which suggests that:
$$ _1 = max_boldsymbolp left boldsymbolp^T D boldsymbolx mid boldsymbolp right_infty leq 1 right $$
Hence our problem becomes:
$$ arg min_ boldsymbolu frac12 boldsymbolu - boldsymbolf right_2^2 + lambda left| D boldsymbolu right|_1 = arg min_ boldsymbolu max_ left_infty leq 1 frac12 boldsymbolu - boldsymbolf right_2^2 + lambda boldsymbolp^T D boldsymbolu $$
Using the Min Max Theorem (The objective is Convex with respect to $ boldsymbolu $ and Concave with respect to $ boldsymbolp $) one could switch the order of the Maximum and Minimum which yields:
$$ arg max_ left_infty leq 1 min_ boldsymbolu frac12 boldsymbolu - boldsymbolf right_2^2 + lambda boldsymbolp^T D boldsymbolu $$
Which can be solved pretty easily with Projected Gradient Descent.
Remarks
- The solver in its FISTA (A Fast Iterative Shrinkage Thresholding Algorithm for Linear Inverse Problems) form is much faster with a simple tweak.
- The code use matrix form for simplicity. In practice all operation should be done using Convolution in the case of the TV.
$endgroup$
The basic idea is to right the problem in a discrete manner.
Let's start with a 1D example.
Imagine the discrete realization of $ f $ is given by the vector $ boldsymbolf in mathbbR^n $.
The problem becomes:
$$ hat boldsymbolu = arg min_ boldsymbolu frac12 boldsymbolu - boldsymbolf right_2^2 + lambda left| D boldsymbolu right|_1 $$
Where $ D $ is finite differences operator in Matrix form such that:
$$ left| D boldsymbolu right|_1 = sum_i = 2^n left| boldsymbolu_i - boldsymbolu_i - 1 right| $$
This is basically Linear Least Squares with Total Variation Regularization.
There are may methods to solve this, for instance, see The Dual Norm of Total Variation Norm (Form of ⟨⋅,⋅⟩) By Smoothing.
Modern way of solving this is using the Alternating Direction Method of Multipliers (ADMM) with great resource on this subject would be Professor Stephen P. Boyd page on ADMM.
Assume the solution to that is given by a Black Box - 1D TV Denoising.
I will point out more than that, let's assume our solution can solve the problem above for any matrix $ D $ (Not specifically Forward / Backward Differences operator).
We'll get to a specific solution and code for it later, but let's assume we have it.
We have in image in 2D space but we could vectorize it into a column stack form using the Vectorization Operator.
It is easy to see the Fidelity Term (The $ L_2 $ term) in our optimization works well. But we have to deal with the 2nd term - The TV Operator.
The TV operator could be defined in (At least?) 2 ways in 2D.
The 2 form of the TV Operators in 2D is $ mathbfTV left( X right) = mathbbR^m times n to mathbbR $
- 2D Anistoropic Total Variation
$$ mathbfTV_I left( X right) = sum_i = 1^m - 1 sum_j = 1^n - 1 sqrt left( X_i, j - X_i + 1, j right)^2 + left( X_i, j - X_i, j + 1 right)^2 + sum_i = 1^m - 1 left| X_i, n - X_i + 1, n right| + sum_j = 1^n - 1 left| X_m, j - X_m, j + 1 right| $$ - 2D Isotropic Total Variation
$$ mathbfTV_A left( X right) = sum_i = 1^m - 1 sum_j = 1^m left| X_i, j - X_i + 1, j right| + sum_i = 1^m sum_j = 1^n - 1 left| X_i, j - X_i, j + 1 right| $$
Remark
One could come up with many more variations. For instance, include the diagonals as well.
I will ignore the Isotropic version for this case (Though it is not hard to solve it as well).
The Anisotropic Case is very similar to the 1D case with on difference, we need to create 2 subtractions for each pixel.
So with some tweaking of the matrix we can write is in the same model of the 1D case.
We just need to update $ D $ to be $ D : mathbbR^m n to mathbbR^2 m n - m - n $.
So we need a code to build the matrix $ D $ in the 2D case and the solver to the 1D case and we're done.
2D Gradient Operator
Work in Progress
Results
So, the implemented code yields as following (The reference being CVX based solution):
As can be seen above, the numerical solver can achieve the optimal solution.
The code is available at my StackExchange Mathematics Q3164164 GitHub Repository.
Black Box Solver
Relatively simple yet effective method is An Algorithm for Total Variation Minimization and Applications.
The idea is the use of Dual Norm / Support Function which suggests that:
$$ _1 = max_boldsymbolp left boldsymbolp^T D boldsymbolx mid boldsymbolp right_infty leq 1 right $$
Hence our problem becomes:
$$ arg min_ boldsymbolu frac12 boldsymbolu - boldsymbolf right_2^2 + lambda left| D boldsymbolu right|_1 = arg min_ boldsymbolu max_ left_infty leq 1 frac12 boldsymbolu - boldsymbolf right_2^2 + lambda boldsymbolp^T D boldsymbolu $$
Using the Min Max Theorem (The objective is Convex with respect to $ boldsymbolu $ and Concave with respect to $ boldsymbolp $) one could switch the order of the Maximum and Minimum which yields:
$$ arg max_ left_infty leq 1 min_ boldsymbolu frac12 boldsymbolu - boldsymbolf right_2^2 + lambda boldsymbolp^T D boldsymbolu $$
Which can be solved pretty easily with Projected Gradient Descent.
Remarks
- The solver in its FISTA (A Fast Iterative Shrinkage Thresholding Algorithm for Linear Inverse Problems) form is much faster with a simple tweak.
- The code use matrix form for simplicity. In practice all operation should be done using Convolution in the case of the TV.
edited Mar 29 at 23:40
answered Mar 27 at 9:04
RoyiRoyi
3,66512454
3,66512454
$begingroup$
Wow, this is amazing. Thank you so much for your effort. I keep seeing this TV norm minimization everywhere, but did not have a sense of the implementation. This is really really helpful. Hopefully you get a lot of good karma :).
$endgroup$
– krishnab
Mar 29 at 23:49
add a comment |
$begingroup$
Wow, this is amazing. Thank you so much for your effort. I keep seeing this TV norm minimization everywhere, but did not have a sense of the implementation. This is really really helpful. Hopefully you get a lot of good karma :).
$endgroup$
– krishnab
Mar 29 at 23:49
$begingroup$
Wow, this is amazing. Thank you so much for your effort. I keep seeing this TV norm minimization everywhere, but did not have a sense of the implementation. This is really really helpful. Hopefully you get a lot of good karma :).
$endgroup$
– krishnab
Mar 29 at 23:49
$begingroup$
Wow, this is amazing. Thank you so much for your effort. I keep seeing this TV norm minimization everywhere, but did not have a sense of the implementation. This is really really helpful. Hopefully you get a lot of good karma :).
$endgroup$
– krishnab
Mar 29 at 23:49
add a comment |
Thanks for contributing an answer to Mathematics Stack Exchange!
- Please be sure to answer the question. Provide details and share your research!
But avoid …
- Asking for help, clarification, or responding to other answers.
- Making statements based on opinion; back them up with references or personal experience.
Use MathJax to format equations. MathJax reference.
To learn more, see our tips on writing great answers.
Sign up or log in
StackExchange.ready(function ()
StackExchange.helpers.onClickDraftSave('#login-link');
);
Sign up using Google
Sign up using Facebook
Sign up using Email and Password
Post as a guest
Required, but never shown
StackExchange.ready(
function ()
StackExchange.openid.initPostLogin('.new-post-login', 'https%3a%2f%2fmath.stackexchange.com%2fquestions%2f3164164%2fnumerical-implementation-solution-for-the-euler-lagrange-equation-of-the-rudin%23new-answer', 'question_page');
);
Post as a guest
Required, but never shown
Sign up or log in
StackExchange.ready(function ()
StackExchange.helpers.onClickDraftSave('#login-link');
);
Sign up using Google
Sign up using Facebook
Sign up using Email and Password
Post as a guest
Required, but never shown
Sign up or log in
StackExchange.ready(function ()
StackExchange.helpers.onClickDraftSave('#login-link');
);
Sign up using Google
Sign up using Facebook
Sign up using Email and Password
Post as a guest
Required, but never shown
Sign up or log in
StackExchange.ready(function ()
StackExchange.helpers.onClickDraftSave('#login-link');
);
Sign up using Google
Sign up using Facebook
Sign up using Email and Password
Sign up using Google
Sign up using Facebook
Sign up using Email and Password
Post as a guest
Required, but never shown
Required, but never shown
Required, but never shown
Required, but never shown
Required, but never shown
Required, but never shown
Required, but never shown
Required, but never shown
Required, but never shown
1lyW6iOdA5bRTH,Q,zdGX4th8d6 oz4qsx1xnftI3iv2NhoiDHC6a LqzIBA243H82S8
$begingroup$
Working on answer + code for you.
$endgroup$
– Royi
Mar 27 at 6:40
$begingroup$
@Royi Oh that is so great. Man, I imagine so many people would find your code and explanation helpful. It is so important to find some sort of readable code or explanation of how these types of algorithms work for the sake of reproducibility and validation. Anything that you can provide would be appreciated.
$endgroup$
– krishnab
Mar 27 at 7:39
$begingroup$
I added a code for the solution.
$endgroup$
– Royi
Mar 29 at 23:41