Is $(a+bX,X)$ jointly normal when $X$ is normal? The Next CEO of Stack OverflowDegenerate Bivariate NormalProve that (X,Y) is bivariate normal if X is normal and Y conditionally on X is normalProve that (X,Y) is bivariate normal if X is normal and Y conditionally on X is normalDoes it matter here that random variables are jointly normally distributed?Independence and uncorrelatedness between two normal random vectors.How do you prove 2 normal random variables X and Y are jointly normally distributed?Let $X$ and $Y$ be of the same dimension and jointly normal. Find the distribution of $X+Y$.Looking for help with dependent jointly normal/Gaussian RVs!Jointly normal and correlated normal random variablesCan two continuous random variables not be jointly continuous?Definition of Jointly NormalGetting jointly normal variables from the vector of jointly standard normal random variables
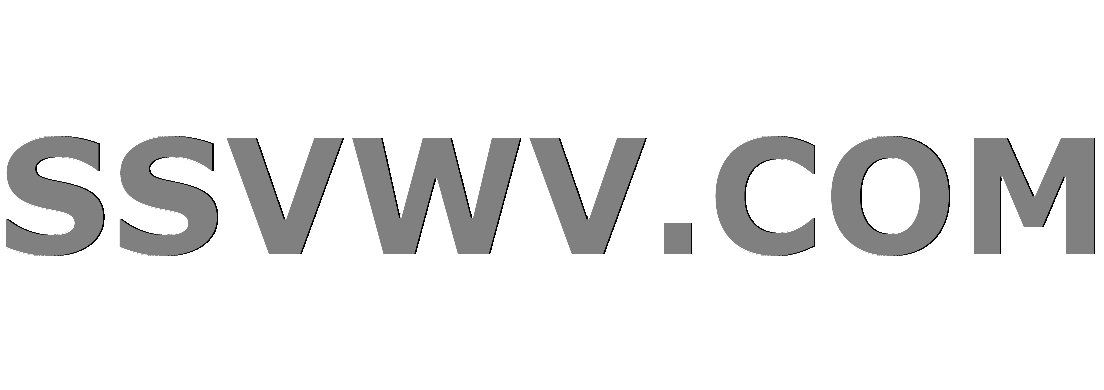
Multi tool use
Can we say or write : "No, it'sn't"?
What steps are necessary to read a Modern SSD in Medieval Europe?
Is it possible to use a NPN BJT as switch, from single power source?
Can you be charged for obstruction for refusing to answer questions?
A small doubt about the dominated convergence theorem
If Nick Fury and Coulson already knew about aliens (Kree and Skrull) why did they wait until Thor's appearance to start making weapons?
The exact meaning of 'Mom made me a sandwich'
Proper way to express "He disappeared them"
How to invert MapIndexed on a ragged structure? How to construct a tree from rules?
How do I align (1) and (2)?
Legal workarounds for testamentary trust perceived as unfair
Is a distribution that is normal, but highly skewed considered Gaussian?
Is it convenient to ask the journal's editor for two additional days to complete a review?
Why the difference in type-inference over the as-pattern in two similar function definitions?
What did we know about the Kessel run before the prequels?
How to avoid supervisors with prejudiced views?
Is it professional to write unrelated content in an almost-empty email?
Is micro rebar a better way to reinforce concrete than rebar?
What happened in Rome, when the western empire "fell"?
RigExpert AA-35 - Interpreting The Information
Calculator final project in Python
Can MTA send mail via a relay without being told so?
A Man With a Stainless Steel Endoskeleton (like The Terminator) Fighting Cloaked Aliens Only He Can See
Would this house-rule that treats advantage as a +1 to the roll instead (and disadvantage as -1) and allows them to stack be balanced?
Is $(a+bX,X)$ jointly normal when $X$ is normal?
The Next CEO of Stack OverflowDegenerate Bivariate NormalProve that (X,Y) is bivariate normal if X is normal and Y conditionally on X is normalProve that (X,Y) is bivariate normal if X is normal and Y conditionally on X is normalDoes it matter here that random variables are jointly normally distributed?Independence and uncorrelatedness between two normal random vectors.How do you prove 2 normal random variables X and Y are jointly normally distributed?Let $X$ and $Y$ be of the same dimension and jointly normal. Find the distribution of $X+Y$.Looking for help with dependent jointly normal/Gaussian RVs!Jointly normal and correlated normal random variablesCan two continuous random variables not be jointly continuous?Definition of Jointly NormalGetting jointly normal variables from the vector of jointly standard normal random variables
$begingroup$
Let $X$ be a normal random variable and $Y=a+bX$, where $a,b$ are just some constants.
Then, is it true that $(Y,X)$ are jointly normal? If yes, how can I easily see that?
Thanks!
probability probability-theory normal-distribution
$endgroup$
add a comment |
$begingroup$
Let $X$ be a normal random variable and $Y=a+bX$, where $a,b$ are just some constants.
Then, is it true that $(Y,X)$ are jointly normal? If yes, how can I easily see that?
Thanks!
probability probability-theory normal-distribution
$endgroup$
$begingroup$
Just follow the calculation which is made here by Did
$endgroup$
– callculus
Mar 15 at 17:23
$begingroup$
Too complicated. But maybe I got it now, can you confirm: As Y is linear in X, all linear combinations of X and Y are linear in X and hence normal. Per definition, if all linear combinations of two normal variables (here: X,Y) are normal, they are jointly normal.
$endgroup$
– S_Z
Mar 15 at 17:30
1
$begingroup$
@callculus In that link, $Ymid X$ is normal. Here, $Y$ is normal.
$endgroup$
– StubbornAtom
Mar 15 at 17:39
add a comment |
$begingroup$
Let $X$ be a normal random variable and $Y=a+bX$, where $a,b$ are just some constants.
Then, is it true that $(Y,X)$ are jointly normal? If yes, how can I easily see that?
Thanks!
probability probability-theory normal-distribution
$endgroup$
Let $X$ be a normal random variable and $Y=a+bX$, where $a,b$ are just some constants.
Then, is it true that $(Y,X)$ are jointly normal? If yes, how can I easily see that?
Thanks!
probability probability-theory normal-distribution
probability probability-theory normal-distribution
edited Mar 15 at 17:41


StubbornAtom
6,30831440
6,30831440
asked Mar 15 at 17:16
S_ZS_Z
121
121
$begingroup$
Just follow the calculation which is made here by Did
$endgroup$
– callculus
Mar 15 at 17:23
$begingroup$
Too complicated. But maybe I got it now, can you confirm: As Y is linear in X, all linear combinations of X and Y are linear in X and hence normal. Per definition, if all linear combinations of two normal variables (here: X,Y) are normal, they are jointly normal.
$endgroup$
– S_Z
Mar 15 at 17:30
1
$begingroup$
@callculus In that link, $Ymid X$ is normal. Here, $Y$ is normal.
$endgroup$
– StubbornAtom
Mar 15 at 17:39
add a comment |
$begingroup$
Just follow the calculation which is made here by Did
$endgroup$
– callculus
Mar 15 at 17:23
$begingroup$
Too complicated. But maybe I got it now, can you confirm: As Y is linear in X, all linear combinations of X and Y are linear in X and hence normal. Per definition, if all linear combinations of two normal variables (here: X,Y) are normal, they are jointly normal.
$endgroup$
– S_Z
Mar 15 at 17:30
1
$begingroup$
@callculus In that link, $Ymid X$ is normal. Here, $Y$ is normal.
$endgroup$
– StubbornAtom
Mar 15 at 17:39
$begingroup$
Just follow the calculation which is made here by Did
$endgroup$
– callculus
Mar 15 at 17:23
$begingroup$
Just follow the calculation which is made here by Did
$endgroup$
– callculus
Mar 15 at 17:23
$begingroup$
Too complicated. But maybe I got it now, can you confirm: As Y is linear in X, all linear combinations of X and Y are linear in X and hence normal. Per definition, if all linear combinations of two normal variables (here: X,Y) are normal, they are jointly normal.
$endgroup$
– S_Z
Mar 15 at 17:30
$begingroup$
Too complicated. But maybe I got it now, can you confirm: As Y is linear in X, all linear combinations of X and Y are linear in X and hence normal. Per definition, if all linear combinations of two normal variables (here: X,Y) are normal, they are jointly normal.
$endgroup$
– S_Z
Mar 15 at 17:30
1
1
$begingroup$
@callculus In that link, $Ymid X$ is normal. Here, $Y$ is normal.
$endgroup$
– StubbornAtom
Mar 15 at 17:39
$begingroup$
@callculus In that link, $Ymid X$ is normal. Here, $Y$ is normal.
$endgroup$
– StubbornAtom
Mar 15 at 17:39
add a comment |
3 Answers
3
active
oldest
votes
$begingroup$
Suppose $Xsim N(mu,sigma^2)$.
Then the dispersion matrix of $(Y,X)$ is
beginalign
Sigma=sigma^2beginpmatrixb^2 & b \ b & 1 endpmatrix
endalign
Since $Sigma$ does not have full rank, the joint distribution of $(Y,X)$ is a degenerate bivariate normal.
The degenerate bivariate normal is not expected to possess all the regular properties of the usual (nonsingular) bivariate normal. Notably, $(Y,X)$ does not enjoy a joint density.
You can see this from the fact that the correlation $rho$ between $Y$ and $X$ satisfies $rho^2=1$. In other words there exists a perfect linear relationship between $Y$ and $X$, with the random point $(Y,X)$ falling on a fixed line with probability one.
For details on this degenerate distribution, check out this excellent post on Cross Validated.
$endgroup$
add a comment |
$begingroup$
The answer is Yes. One of the equivalent definition of jointly normal is as follow:
Let $(Omega,mathcalF,P)$ be a probability space. We say that
a random vector $(X_1,X_2,ldots,X_m)$ is jointly normal if
there exist independent random variables $Z_1,Z_2,ldots,Z_n$
such that $Z_jsim N(0,1)$ for each $j=1,ldots,n$ and for each
$i=1,ldots,m$, $X_iinmboxspan1, Z_1,Z_2,ldots,Z_n$
(i.e., $X_i=sum_j=1^nalpha_ijZ_j+beta_i$ for some $alpha_ij,beta_iinmathbbR$).
Beware of degerated cases, for example, $(0,0,ldots,0)$ is regarded
as jointly normal.
We go back to your case. Suppose that the given $X$ is not degenerated
(i.e., $sigma_X>0$). Define $Z=fracX-mu_Xsigma_X$,
then $Zsim N(0,1)$. Now $a+bXinmboxspan(Z)$ and $Xinmboxspan(Z)$,
so $(a+bX,X)$ is jointly normal.
$endgroup$
add a comment |
$begingroup$
I have some doubt to say no or yes. but I can say:
$(a+bX,X)$ have not joint density ( singular distribution )
$(a+bX,X)$ has not joint density$
$(Y|X=x) =
left{
beginarraycc
x & p=1 \
o.w & p=0
endarray
right.
$ $hspace1cm$ (1)
$F_(X,Y)(x,y)=P(Xleq x, Yleq y)=P(A)=E(I_A)=E(E(I_A)|X)
=int E(I_A|X=t) f_X(t) dt=int E(I_(Xleq x, Yleq y)|X=t) f_X(t) dtoverset(1)=
int E(I_(Xleq x, a+bXleq y)|X=t) f_X(t) dt=
int E(I_(tleq x, a+btleq y)|X=t) f_X(t) dt=
int E(I_(tleq x, tleq fracy-ab)|X=t) f_X(t) dt=
int_-infty^min(x,fracy-ab) E(I_(tleq x, tleq fracy-ab)|X=t) f_X(t) dt+0=int_-infty^min(x,fracy-ab) E(1|X=t) f_X(t) dt=
int_-infty^min(x,fracy-ab) f_X(t) dt=F_X(min(x,fracy-ab))
$
so it easy now to say $(a+bX,X)$ have not joint density.
$F_(X,Y)(x,y)=F_X(min(x,fracy-ab))$
$f_(X,Y)(x,y)=fracd^2dx dyF_(X,Y)(x,y)=0$
this is a singular distribution. so , p.d.f of(X,Y) are not normal density.
to say yes you should check this:
$X_ktimes 1 sim N(mu_ktimes 1, Sigma) iff $ there exist
$mu in R^k $ and $A_Ktimes L in R^ktimes L$ such that $X_Ktimes 1=A_Ktimes LZ_Ltimes 1+mu_Ktimes 1 $ for $Z_noverseti.i.dsim N(0,1)$
to say yes ,so you should find $A$ such that
$left[ beginarrayc X \ a+bX endarray right]
=A_2times L Z_Ltimes 1 +mu $
go and find $A$ and check this:
( $left[ beginarrayc X \ a+bX endarray right]$
and $A_2times L Z_Ltimes 1 $ have a same family and $Z_noverseti.i.dsim N(0,1)$ "i.i.d" )
$(Y|X=x)$ is not a normal
$(Y|X=x)$ is not a normal . it is a degenerated in point $x$. if you say
$left[ beginarrayc X \ a+bX endarray right]$ is joint normal so
conditional distribution should be normal. note that conditional distribution exists and not normal!
$endgroup$
add a comment |
Your Answer
StackExchange.ifUsing("editor", function ()
return StackExchange.using("mathjaxEditing", function ()
StackExchange.MarkdownEditor.creationCallbacks.add(function (editor, postfix)
StackExchange.mathjaxEditing.prepareWmdForMathJax(editor, postfix, [["$", "$"], ["\\(","\\)"]]);
);
);
, "mathjax-editing");
StackExchange.ready(function()
var channelOptions =
tags: "".split(" "),
id: "69"
;
initTagRenderer("".split(" "), "".split(" "), channelOptions);
StackExchange.using("externalEditor", function()
// Have to fire editor after snippets, if snippets enabled
if (StackExchange.settings.snippets.snippetsEnabled)
StackExchange.using("snippets", function()
createEditor();
);
else
createEditor();
);
function createEditor()
StackExchange.prepareEditor(
heartbeatType: 'answer',
autoActivateHeartbeat: false,
convertImagesToLinks: true,
noModals: true,
showLowRepImageUploadWarning: true,
reputationToPostImages: 10,
bindNavPrevention: true,
postfix: "",
imageUploader:
brandingHtml: "Powered by u003ca class="icon-imgur-white" href="https://imgur.com/"u003eu003c/au003e",
contentPolicyHtml: "User contributions licensed under u003ca href="https://creativecommons.org/licenses/by-sa/3.0/"u003ecc by-sa 3.0 with attribution requiredu003c/au003e u003ca href="https://stackoverflow.com/legal/content-policy"u003e(content policy)u003c/au003e",
allowUrls: true
,
noCode: true, onDemand: true,
discardSelector: ".discard-answer"
,immediatelyShowMarkdownHelp:true
);
);
Sign up or log in
StackExchange.ready(function ()
StackExchange.helpers.onClickDraftSave('#login-link');
);
Sign up using Google
Sign up using Facebook
Sign up using Email and Password
Post as a guest
Required, but never shown
StackExchange.ready(
function ()
StackExchange.openid.initPostLogin('.new-post-login', 'https%3a%2f%2fmath.stackexchange.com%2fquestions%2f3149562%2fis-abx-x-jointly-normal-when-x-is-normal%23new-answer', 'question_page');
);
Post as a guest
Required, but never shown
3 Answers
3
active
oldest
votes
3 Answers
3
active
oldest
votes
active
oldest
votes
active
oldest
votes
$begingroup$
Suppose $Xsim N(mu,sigma^2)$.
Then the dispersion matrix of $(Y,X)$ is
beginalign
Sigma=sigma^2beginpmatrixb^2 & b \ b & 1 endpmatrix
endalign
Since $Sigma$ does not have full rank, the joint distribution of $(Y,X)$ is a degenerate bivariate normal.
The degenerate bivariate normal is not expected to possess all the regular properties of the usual (nonsingular) bivariate normal. Notably, $(Y,X)$ does not enjoy a joint density.
You can see this from the fact that the correlation $rho$ between $Y$ and $X$ satisfies $rho^2=1$. In other words there exists a perfect linear relationship between $Y$ and $X$, with the random point $(Y,X)$ falling on a fixed line with probability one.
For details on this degenerate distribution, check out this excellent post on Cross Validated.
$endgroup$
add a comment |
$begingroup$
Suppose $Xsim N(mu,sigma^2)$.
Then the dispersion matrix of $(Y,X)$ is
beginalign
Sigma=sigma^2beginpmatrixb^2 & b \ b & 1 endpmatrix
endalign
Since $Sigma$ does not have full rank, the joint distribution of $(Y,X)$ is a degenerate bivariate normal.
The degenerate bivariate normal is not expected to possess all the regular properties of the usual (nonsingular) bivariate normal. Notably, $(Y,X)$ does not enjoy a joint density.
You can see this from the fact that the correlation $rho$ between $Y$ and $X$ satisfies $rho^2=1$. In other words there exists a perfect linear relationship between $Y$ and $X$, with the random point $(Y,X)$ falling on a fixed line with probability one.
For details on this degenerate distribution, check out this excellent post on Cross Validated.
$endgroup$
add a comment |
$begingroup$
Suppose $Xsim N(mu,sigma^2)$.
Then the dispersion matrix of $(Y,X)$ is
beginalign
Sigma=sigma^2beginpmatrixb^2 & b \ b & 1 endpmatrix
endalign
Since $Sigma$ does not have full rank, the joint distribution of $(Y,X)$ is a degenerate bivariate normal.
The degenerate bivariate normal is not expected to possess all the regular properties of the usual (nonsingular) bivariate normal. Notably, $(Y,X)$ does not enjoy a joint density.
You can see this from the fact that the correlation $rho$ between $Y$ and $X$ satisfies $rho^2=1$. In other words there exists a perfect linear relationship between $Y$ and $X$, with the random point $(Y,X)$ falling on a fixed line with probability one.
For details on this degenerate distribution, check out this excellent post on Cross Validated.
$endgroup$
Suppose $Xsim N(mu,sigma^2)$.
Then the dispersion matrix of $(Y,X)$ is
beginalign
Sigma=sigma^2beginpmatrixb^2 & b \ b & 1 endpmatrix
endalign
Since $Sigma$ does not have full rank, the joint distribution of $(Y,X)$ is a degenerate bivariate normal.
The degenerate bivariate normal is not expected to possess all the regular properties of the usual (nonsingular) bivariate normal. Notably, $(Y,X)$ does not enjoy a joint density.
You can see this from the fact that the correlation $rho$ between $Y$ and $X$ satisfies $rho^2=1$. In other words there exists a perfect linear relationship between $Y$ and $X$, with the random point $(Y,X)$ falling on a fixed line with probability one.
For details on this degenerate distribution, check out this excellent post on Cross Validated.
edited Mar 17 at 15:06
answered Mar 16 at 14:04


StubbornAtomStubbornAtom
6,30831440
6,30831440
add a comment |
add a comment |
$begingroup$
The answer is Yes. One of the equivalent definition of jointly normal is as follow:
Let $(Omega,mathcalF,P)$ be a probability space. We say that
a random vector $(X_1,X_2,ldots,X_m)$ is jointly normal if
there exist independent random variables $Z_1,Z_2,ldots,Z_n$
such that $Z_jsim N(0,1)$ for each $j=1,ldots,n$ and for each
$i=1,ldots,m$, $X_iinmboxspan1, Z_1,Z_2,ldots,Z_n$
(i.e., $X_i=sum_j=1^nalpha_ijZ_j+beta_i$ for some $alpha_ij,beta_iinmathbbR$).
Beware of degerated cases, for example, $(0,0,ldots,0)$ is regarded
as jointly normal.
We go back to your case. Suppose that the given $X$ is not degenerated
(i.e., $sigma_X>0$). Define $Z=fracX-mu_Xsigma_X$,
then $Zsim N(0,1)$. Now $a+bXinmboxspan(Z)$ and $Xinmboxspan(Z)$,
so $(a+bX,X)$ is jointly normal.
$endgroup$
add a comment |
$begingroup$
The answer is Yes. One of the equivalent definition of jointly normal is as follow:
Let $(Omega,mathcalF,P)$ be a probability space. We say that
a random vector $(X_1,X_2,ldots,X_m)$ is jointly normal if
there exist independent random variables $Z_1,Z_2,ldots,Z_n$
such that $Z_jsim N(0,1)$ for each $j=1,ldots,n$ and for each
$i=1,ldots,m$, $X_iinmboxspan1, Z_1,Z_2,ldots,Z_n$
(i.e., $X_i=sum_j=1^nalpha_ijZ_j+beta_i$ for some $alpha_ij,beta_iinmathbbR$).
Beware of degerated cases, for example, $(0,0,ldots,0)$ is regarded
as jointly normal.
We go back to your case. Suppose that the given $X$ is not degenerated
(i.e., $sigma_X>0$). Define $Z=fracX-mu_Xsigma_X$,
then $Zsim N(0,1)$. Now $a+bXinmboxspan(Z)$ and $Xinmboxspan(Z)$,
so $(a+bX,X)$ is jointly normal.
$endgroup$
add a comment |
$begingroup$
The answer is Yes. One of the equivalent definition of jointly normal is as follow:
Let $(Omega,mathcalF,P)$ be a probability space. We say that
a random vector $(X_1,X_2,ldots,X_m)$ is jointly normal if
there exist independent random variables $Z_1,Z_2,ldots,Z_n$
such that $Z_jsim N(0,1)$ for each $j=1,ldots,n$ and for each
$i=1,ldots,m$, $X_iinmboxspan1, Z_1,Z_2,ldots,Z_n$
(i.e., $X_i=sum_j=1^nalpha_ijZ_j+beta_i$ for some $alpha_ij,beta_iinmathbbR$).
Beware of degerated cases, for example, $(0,0,ldots,0)$ is regarded
as jointly normal.
We go back to your case. Suppose that the given $X$ is not degenerated
(i.e., $sigma_X>0$). Define $Z=fracX-mu_Xsigma_X$,
then $Zsim N(0,1)$. Now $a+bXinmboxspan(Z)$ and $Xinmboxspan(Z)$,
so $(a+bX,X)$ is jointly normal.
$endgroup$
The answer is Yes. One of the equivalent definition of jointly normal is as follow:
Let $(Omega,mathcalF,P)$ be a probability space. We say that
a random vector $(X_1,X_2,ldots,X_m)$ is jointly normal if
there exist independent random variables $Z_1,Z_2,ldots,Z_n$
such that $Z_jsim N(0,1)$ for each $j=1,ldots,n$ and for each
$i=1,ldots,m$, $X_iinmboxspan1, Z_1,Z_2,ldots,Z_n$
(i.e., $X_i=sum_j=1^nalpha_ijZ_j+beta_i$ for some $alpha_ij,beta_iinmathbbR$).
Beware of degerated cases, for example, $(0,0,ldots,0)$ is regarded
as jointly normal.
We go back to your case. Suppose that the given $X$ is not degenerated
(i.e., $sigma_X>0$). Define $Z=fracX-mu_Xsigma_X$,
then $Zsim N(0,1)$. Now $a+bXinmboxspan(Z)$ and $Xinmboxspan(Z)$,
so $(a+bX,X)$ is jointly normal.
edited Mar 15 at 21:29
answered Mar 15 at 21:03
Danny Pak-Keung ChanDanny Pak-Keung Chan
2,55938
2,55938
add a comment |
add a comment |
$begingroup$
I have some doubt to say no or yes. but I can say:
$(a+bX,X)$ have not joint density ( singular distribution )
$(a+bX,X)$ has not joint density$
$(Y|X=x) =
left{
beginarraycc
x & p=1 \
o.w & p=0
endarray
right.
$ $hspace1cm$ (1)
$F_(X,Y)(x,y)=P(Xleq x, Yleq y)=P(A)=E(I_A)=E(E(I_A)|X)
=int E(I_A|X=t) f_X(t) dt=int E(I_(Xleq x, Yleq y)|X=t) f_X(t) dtoverset(1)=
int E(I_(Xleq x, a+bXleq y)|X=t) f_X(t) dt=
int E(I_(tleq x, a+btleq y)|X=t) f_X(t) dt=
int E(I_(tleq x, tleq fracy-ab)|X=t) f_X(t) dt=
int_-infty^min(x,fracy-ab) E(I_(tleq x, tleq fracy-ab)|X=t) f_X(t) dt+0=int_-infty^min(x,fracy-ab) E(1|X=t) f_X(t) dt=
int_-infty^min(x,fracy-ab) f_X(t) dt=F_X(min(x,fracy-ab))
$
so it easy now to say $(a+bX,X)$ have not joint density.
$F_(X,Y)(x,y)=F_X(min(x,fracy-ab))$
$f_(X,Y)(x,y)=fracd^2dx dyF_(X,Y)(x,y)=0$
this is a singular distribution. so , p.d.f of(X,Y) are not normal density.
to say yes you should check this:
$X_ktimes 1 sim N(mu_ktimes 1, Sigma) iff $ there exist
$mu in R^k $ and $A_Ktimes L in R^ktimes L$ such that $X_Ktimes 1=A_Ktimes LZ_Ltimes 1+mu_Ktimes 1 $ for $Z_noverseti.i.dsim N(0,1)$
to say yes ,so you should find $A$ such that
$left[ beginarrayc X \ a+bX endarray right]
=A_2times L Z_Ltimes 1 +mu $
go and find $A$ and check this:
( $left[ beginarrayc X \ a+bX endarray right]$
and $A_2times L Z_Ltimes 1 $ have a same family and $Z_noverseti.i.dsim N(0,1)$ "i.i.d" )
$(Y|X=x)$ is not a normal
$(Y|X=x)$ is not a normal . it is a degenerated in point $x$. if you say
$left[ beginarrayc X \ a+bX endarray right]$ is joint normal so
conditional distribution should be normal. note that conditional distribution exists and not normal!
$endgroup$
add a comment |
$begingroup$
I have some doubt to say no or yes. but I can say:
$(a+bX,X)$ have not joint density ( singular distribution )
$(a+bX,X)$ has not joint density$
$(Y|X=x) =
left{
beginarraycc
x & p=1 \
o.w & p=0
endarray
right.
$ $hspace1cm$ (1)
$F_(X,Y)(x,y)=P(Xleq x, Yleq y)=P(A)=E(I_A)=E(E(I_A)|X)
=int E(I_A|X=t) f_X(t) dt=int E(I_(Xleq x, Yleq y)|X=t) f_X(t) dtoverset(1)=
int E(I_(Xleq x, a+bXleq y)|X=t) f_X(t) dt=
int E(I_(tleq x, a+btleq y)|X=t) f_X(t) dt=
int E(I_(tleq x, tleq fracy-ab)|X=t) f_X(t) dt=
int_-infty^min(x,fracy-ab) E(I_(tleq x, tleq fracy-ab)|X=t) f_X(t) dt+0=int_-infty^min(x,fracy-ab) E(1|X=t) f_X(t) dt=
int_-infty^min(x,fracy-ab) f_X(t) dt=F_X(min(x,fracy-ab))
$
so it easy now to say $(a+bX,X)$ have not joint density.
$F_(X,Y)(x,y)=F_X(min(x,fracy-ab))$
$f_(X,Y)(x,y)=fracd^2dx dyF_(X,Y)(x,y)=0$
this is a singular distribution. so , p.d.f of(X,Y) are not normal density.
to say yes you should check this:
$X_ktimes 1 sim N(mu_ktimes 1, Sigma) iff $ there exist
$mu in R^k $ and $A_Ktimes L in R^ktimes L$ such that $X_Ktimes 1=A_Ktimes LZ_Ltimes 1+mu_Ktimes 1 $ for $Z_noverseti.i.dsim N(0,1)$
to say yes ,so you should find $A$ such that
$left[ beginarrayc X \ a+bX endarray right]
=A_2times L Z_Ltimes 1 +mu $
go and find $A$ and check this:
( $left[ beginarrayc X \ a+bX endarray right]$
and $A_2times L Z_Ltimes 1 $ have a same family and $Z_noverseti.i.dsim N(0,1)$ "i.i.d" )
$(Y|X=x)$ is not a normal
$(Y|X=x)$ is not a normal . it is a degenerated in point $x$. if you say
$left[ beginarrayc X \ a+bX endarray right]$ is joint normal so
conditional distribution should be normal. note that conditional distribution exists and not normal!
$endgroup$
add a comment |
$begingroup$
I have some doubt to say no or yes. but I can say:
$(a+bX,X)$ have not joint density ( singular distribution )
$(a+bX,X)$ has not joint density$
$(Y|X=x) =
left{
beginarraycc
x & p=1 \
o.w & p=0
endarray
right.
$ $hspace1cm$ (1)
$F_(X,Y)(x,y)=P(Xleq x, Yleq y)=P(A)=E(I_A)=E(E(I_A)|X)
=int E(I_A|X=t) f_X(t) dt=int E(I_(Xleq x, Yleq y)|X=t) f_X(t) dtoverset(1)=
int E(I_(Xleq x, a+bXleq y)|X=t) f_X(t) dt=
int E(I_(tleq x, a+btleq y)|X=t) f_X(t) dt=
int E(I_(tleq x, tleq fracy-ab)|X=t) f_X(t) dt=
int_-infty^min(x,fracy-ab) E(I_(tleq x, tleq fracy-ab)|X=t) f_X(t) dt+0=int_-infty^min(x,fracy-ab) E(1|X=t) f_X(t) dt=
int_-infty^min(x,fracy-ab) f_X(t) dt=F_X(min(x,fracy-ab))
$
so it easy now to say $(a+bX,X)$ have not joint density.
$F_(X,Y)(x,y)=F_X(min(x,fracy-ab))$
$f_(X,Y)(x,y)=fracd^2dx dyF_(X,Y)(x,y)=0$
this is a singular distribution. so , p.d.f of(X,Y) are not normal density.
to say yes you should check this:
$X_ktimes 1 sim N(mu_ktimes 1, Sigma) iff $ there exist
$mu in R^k $ and $A_Ktimes L in R^ktimes L$ such that $X_Ktimes 1=A_Ktimes LZ_Ltimes 1+mu_Ktimes 1 $ for $Z_noverseti.i.dsim N(0,1)$
to say yes ,so you should find $A$ such that
$left[ beginarrayc X \ a+bX endarray right]
=A_2times L Z_Ltimes 1 +mu $
go and find $A$ and check this:
( $left[ beginarrayc X \ a+bX endarray right]$
and $A_2times L Z_Ltimes 1 $ have a same family and $Z_noverseti.i.dsim N(0,1)$ "i.i.d" )
$(Y|X=x)$ is not a normal
$(Y|X=x)$ is not a normal . it is a degenerated in point $x$. if you say
$left[ beginarrayc X \ a+bX endarray right]$ is joint normal so
conditional distribution should be normal. note that conditional distribution exists and not normal!
$endgroup$
I have some doubt to say no or yes. but I can say:
$(a+bX,X)$ have not joint density ( singular distribution )
$(a+bX,X)$ has not joint density$
$(Y|X=x) =
left{
beginarraycc
x & p=1 \
o.w & p=0
endarray
right.
$ $hspace1cm$ (1)
$F_(X,Y)(x,y)=P(Xleq x, Yleq y)=P(A)=E(I_A)=E(E(I_A)|X)
=int E(I_A|X=t) f_X(t) dt=int E(I_(Xleq x, Yleq y)|X=t) f_X(t) dtoverset(1)=
int E(I_(Xleq x, a+bXleq y)|X=t) f_X(t) dt=
int E(I_(tleq x, a+btleq y)|X=t) f_X(t) dt=
int E(I_(tleq x, tleq fracy-ab)|X=t) f_X(t) dt=
int_-infty^min(x,fracy-ab) E(I_(tleq x, tleq fracy-ab)|X=t) f_X(t) dt+0=int_-infty^min(x,fracy-ab) E(1|X=t) f_X(t) dt=
int_-infty^min(x,fracy-ab) f_X(t) dt=F_X(min(x,fracy-ab))
$
so it easy now to say $(a+bX,X)$ have not joint density.
$F_(X,Y)(x,y)=F_X(min(x,fracy-ab))$
$f_(X,Y)(x,y)=fracd^2dx dyF_(X,Y)(x,y)=0$
this is a singular distribution. so , p.d.f of(X,Y) are not normal density.
to say yes you should check this:
$X_ktimes 1 sim N(mu_ktimes 1, Sigma) iff $ there exist
$mu in R^k $ and $A_Ktimes L in R^ktimes L$ such that $X_Ktimes 1=A_Ktimes LZ_Ltimes 1+mu_Ktimes 1 $ for $Z_noverseti.i.dsim N(0,1)$
to say yes ,so you should find $A$ such that
$left[ beginarrayc X \ a+bX endarray right]
=A_2times L Z_Ltimes 1 +mu $
go and find $A$ and check this:
( $left[ beginarrayc X \ a+bX endarray right]$
and $A_2times L Z_Ltimes 1 $ have a same family and $Z_noverseti.i.dsim N(0,1)$ "i.i.d" )
$(Y|X=x)$ is not a normal
$(Y|X=x)$ is not a normal . it is a degenerated in point $x$. if you say
$left[ beginarrayc X \ a+bX endarray right]$ is joint normal so
conditional distribution should be normal. note that conditional distribution exists and not normal!
edited Mar 16 at 0:11
answered Mar 15 at 19:41
masoudmasoud
1155
1155
add a comment |
add a comment |
Thanks for contributing an answer to Mathematics Stack Exchange!
- Please be sure to answer the question. Provide details and share your research!
But avoid …
- Asking for help, clarification, or responding to other answers.
- Making statements based on opinion; back them up with references or personal experience.
Use MathJax to format equations. MathJax reference.
To learn more, see our tips on writing great answers.
Sign up or log in
StackExchange.ready(function ()
StackExchange.helpers.onClickDraftSave('#login-link');
);
Sign up using Google
Sign up using Facebook
Sign up using Email and Password
Post as a guest
Required, but never shown
StackExchange.ready(
function ()
StackExchange.openid.initPostLogin('.new-post-login', 'https%3a%2f%2fmath.stackexchange.com%2fquestions%2f3149562%2fis-abx-x-jointly-normal-when-x-is-normal%23new-answer', 'question_page');
);
Post as a guest
Required, but never shown
Sign up or log in
StackExchange.ready(function ()
StackExchange.helpers.onClickDraftSave('#login-link');
);
Sign up using Google
Sign up using Facebook
Sign up using Email and Password
Post as a guest
Required, but never shown
Sign up or log in
StackExchange.ready(function ()
StackExchange.helpers.onClickDraftSave('#login-link');
);
Sign up using Google
Sign up using Facebook
Sign up using Email and Password
Post as a guest
Required, but never shown
Sign up or log in
StackExchange.ready(function ()
StackExchange.helpers.onClickDraftSave('#login-link');
);
Sign up using Google
Sign up using Facebook
Sign up using Email and Password
Sign up using Google
Sign up using Facebook
Sign up using Email and Password
Post as a guest
Required, but never shown
Required, but never shown
Required, but never shown
Required, but never shown
Required, but never shown
Required, but never shown
Required, but never shown
Required, but never shown
Required, but never shown
0GZYQT3u3q,Ul0lv4cjW9mOu H
$begingroup$
Just follow the calculation which is made here by Did
$endgroup$
– callculus
Mar 15 at 17:23
$begingroup$
Too complicated. But maybe I got it now, can you confirm: As Y is linear in X, all linear combinations of X and Y are linear in X and hence normal. Per definition, if all linear combinations of two normal variables (here: X,Y) are normal, they are jointly normal.
$endgroup$
– S_Z
Mar 15 at 17:30
1
$begingroup$
@callculus In that link, $Ymid X$ is normal. Here, $Y$ is normal.
$endgroup$
– StubbornAtom
Mar 15 at 17:39