Need help understanding what a natural log transformation is actually doing and why specific transformations are required for linear regression [duplicate] The 2019 Stack Overflow Developer Survey Results Are InIn linear regression, when is it appropriate to use the log of an independent variable instead of the actual values?When (and why) should you take the log of a distribution (of numbers)?Box-Cox like transformation for independent variables?Regression: Transforming VariablesBack-transformation and interpretation of $log(X+1)$ estimates in multiple linear regressionAVAS Transformation Interpretation of Multiple Factor Regression Equationlog-log vs. normal plotting for showing linear dependencyWhat is the difference between doing a linear regression and exhaustive descriptive statistics?Log transform before bootstrapping?Interpreting how much my linear model has improved after Box-Cox transformationRequired transformation and regression for non-normal variableTransformation between log and reciprocal powers for regressionusing logit transformation properly in linear model and problems with model given zero F and p values for specific variable combinationsDiscussing R-squared of log-log model with a non-technical audience
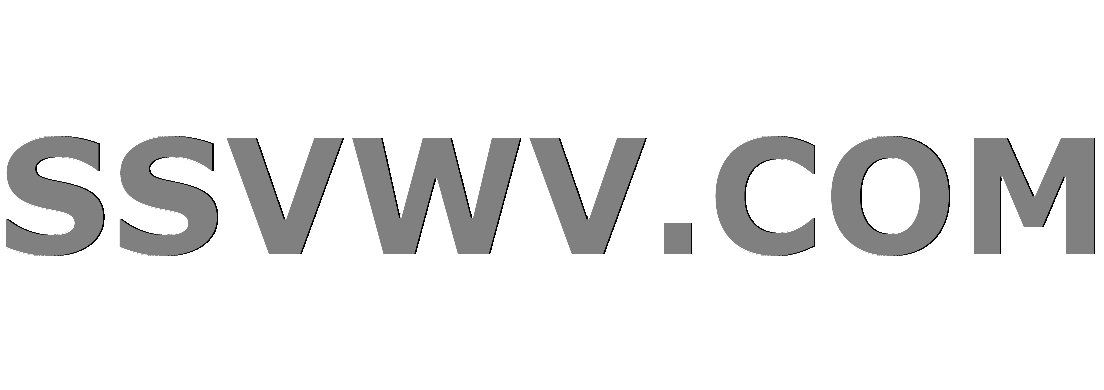
Multi tool use
Can distinct morphisms between curves induce the same morphism on singular cohomology?
Access elements in std::string where positon of string is greater than its size
Inflated grade on resume at previous job, might former employer tell new employer?
What is this 4-propeller plane?
Why is the maximum length of OpenWrt’s root password 8 characters?
It's possible to achieve negative score?
Lethal sonic weapons
Inline version of a function returns different value than non-inline version
Idiomatic way to prevent slicing?
What could be the right powersource for 15 seconds lifespan disposable giant chainsaw?
How can I fix this gap between bookcases I made?
Landlord wants to switch my lease to a "Land contract" to "get back at the city"
Inversion Puzzle
What does "rabbited" mean/imply in this sentence?
How are circuits which use complex ICs normally simulated?
What is the meaning of Triage in Cybersec world?
"To split hairs" vs "To be pedantic"
Why isn't airport relocation done gradually?
Should I use my personal or workplace e-mail when registering to external websites for work purpose?
Pristine Bit Checking
How to change the limits of integration
Patience, young "Padovan"
Realistic Alternatives to Dust: What Else Could Feed a Plankton Bloom?
Why is it "Tumoren" and not "Tumore"?
Need help understanding what a natural log transformation is actually doing and why specific transformations are required for linear regression [duplicate]
The 2019 Stack Overflow Developer Survey Results Are InIn linear regression, when is it appropriate to use the log of an independent variable instead of the actual values?When (and why) should you take the log of a distribution (of numbers)?Box-Cox like transformation for independent variables?Regression: Transforming VariablesBack-transformation and interpretation of $log(X+1)$ estimates in multiple linear regressionAVAS Transformation Interpretation of Multiple Factor Regression Equationlog-log vs. normal plotting for showing linear dependencyWhat is the difference between doing a linear regression and exhaustive descriptive statistics?Log transform before bootstrapping?Interpreting how much my linear model has improved after Box-Cox transformationRequired transformation and regression for non-normal variableTransformation between log and reciprocal powers for regressionusing logit transformation properly in linear model and problems with model given zero F and p values for specific variable combinationsDiscussing R-squared of log-log model with a non-technical audience
.everyoneloves__top-leaderboard:empty,.everyoneloves__mid-leaderboard:empty,.everyoneloves__bot-mid-leaderboard:empty margin-bottom:0;
$begingroup$
This question already has an answer here:
In linear regression, when is it appropriate to use the log of an independent variable instead of the actual values?
8 answers
Regression: Transforming Variables
1 answer
Box-Cox like transformation for independent variables?
3 answers
When (and why) should you take the log of a distribution (of numbers)?
2 answers
I’m taking an online “Intro to AI” course for which I’m doing some azure machine learning labs. This course is largely about how to apply azure ML solutions and, while there is an “essential math for ML module”, it doesn’t do any sort of deep dive into the math/statistics. But I want to understand what’s happening mathematically to a basic level. (I've taken an intro to statistics, that's it so far.) Below are some details about some specific things I’d like to understand more, and my specific questions.
At a particular point in the “training a classification model” lab, there is this text: “The distribution of the Age column in the diabetes.csv dataset is skewed because most patients are in the youngest age bracket. Creating a version of this feature that uses a natural log transformation can help create a more linear relationship between Age and other features, and improve the ability to predict the Diabetic label. This kind of feature engineering as it’s called is common in machine learning data preparation.”
Then the instructions show you how to use Azure ML Studio to apply the natural log operation to the age column in the data set:
And after doing that, the data includes the original/raw age data and the transformed age data:
Question #1: What is that transformation actually doing? I don't mean the nitty gritty math, but what is it doing conceptually?
Question #2: The next general question is around why is a transformation necessary. On that, I did some research and found this article (https://www.r-statistics.com/2013/05/log-transformations-for-skewed-and-wide-distributions-from-practical-data-science-with-r/) that describes which type of log transformations to use in a few scenarios. Here’s a snip of text from the article:
“The need for data transformation can depend on the modeling method that you plan to use. For linear and logistic regression, for example, you ideally want to make sure that the relationship between input variables and output variables is approximately linear, that the input variables are approximately normal in distribution, and that the output variable is constant variance (that is, the variance of the output variable is independent of the input variables). You may need to transform some of your input variables to better meet these assumptions.”
I don’t understand why any of that is necessary. I’ve broken my question down:
For linear and logistic regression, for example, you ideally want to make sure that:
the relationship between input variables and output variables is approximately linear – why?
the input variables are approximately normal in distribution- why?
the output variable is constant variance (that is, the variance of the output variable is independent of the input variables – why?
Could someone help with information for those whys, or point me to material that would help?
regression linear logarithm
$endgroup$
marked as duplicate by whuber♦
StackExchange.ready(function()
if (StackExchange.options.isMobile) return;
$('.dupe-hammer-message-hover:not(.hover-bound)').each(function()
var $hover = $(this).addClass('hover-bound'),
$msg = $hover.siblings('.dupe-hammer-message');
$hover.hover(
function()
$hover.showInfoMessage('',
messageElement: $msg.clone().show(),
transient: false,
position: my: 'bottom left', at: 'top center', offsetTop: -7 ,
dismissable: false,
relativeToBody: true
);
,
function()
StackExchange.helpers.removeMessages();
);
);
);
Mar 23 at 14:05
This question has been asked before and already has an answer. If those answers do not fully address your question, please ask a new question.
|
show 5 more comments
$begingroup$
This question already has an answer here:
In linear regression, when is it appropriate to use the log of an independent variable instead of the actual values?
8 answers
Regression: Transforming Variables
1 answer
Box-Cox like transformation for independent variables?
3 answers
When (and why) should you take the log of a distribution (of numbers)?
2 answers
I’m taking an online “Intro to AI” course for which I’m doing some azure machine learning labs. This course is largely about how to apply azure ML solutions and, while there is an “essential math for ML module”, it doesn’t do any sort of deep dive into the math/statistics. But I want to understand what’s happening mathematically to a basic level. (I've taken an intro to statistics, that's it so far.) Below are some details about some specific things I’d like to understand more, and my specific questions.
At a particular point in the “training a classification model” lab, there is this text: “The distribution of the Age column in the diabetes.csv dataset is skewed because most patients are in the youngest age bracket. Creating a version of this feature that uses a natural log transformation can help create a more linear relationship between Age and other features, and improve the ability to predict the Diabetic label. This kind of feature engineering as it’s called is common in machine learning data preparation.”
Then the instructions show you how to use Azure ML Studio to apply the natural log operation to the age column in the data set:
And after doing that, the data includes the original/raw age data and the transformed age data:
Question #1: What is that transformation actually doing? I don't mean the nitty gritty math, but what is it doing conceptually?
Question #2: The next general question is around why is a transformation necessary. On that, I did some research and found this article (https://www.r-statistics.com/2013/05/log-transformations-for-skewed-and-wide-distributions-from-practical-data-science-with-r/) that describes which type of log transformations to use in a few scenarios. Here’s a snip of text from the article:
“The need for data transformation can depend on the modeling method that you plan to use. For linear and logistic regression, for example, you ideally want to make sure that the relationship between input variables and output variables is approximately linear, that the input variables are approximately normal in distribution, and that the output variable is constant variance (that is, the variance of the output variable is independent of the input variables). You may need to transform some of your input variables to better meet these assumptions.”
I don’t understand why any of that is necessary. I’ve broken my question down:
For linear and logistic regression, for example, you ideally want to make sure that:
the relationship between input variables and output variables is approximately linear – why?
the input variables are approximately normal in distribution- why?
the output variable is constant variance (that is, the variance of the output variable is independent of the input variables – why?
Could someone help with information for those whys, or point me to material that would help?
regression linear logarithm
$endgroup$
marked as duplicate by whuber♦
StackExchange.ready(function()
if (StackExchange.options.isMobile) return;
$('.dupe-hammer-message-hover:not(.hover-bound)').each(function()
var $hover = $(this).addClass('hover-bound'),
$msg = $hover.siblings('.dupe-hammer-message');
$hover.hover(
function()
$hover.showInfoMessage('',
messageElement: $msg.clone().show(),
transient: false,
position: my: 'bottom left', at: 'top center', offsetTop: -7 ,
dismissable: false,
relativeToBody: true
);
,
function()
StackExchange.helpers.removeMessages();
);
);
);
Mar 23 at 14:05
This question has been asked before and already has an answer. If those answers do not fully address your question, please ask a new question.
$begingroup$
Perhaps this will help stats.stackexchange.com/questions/18844/… .
$endgroup$
– IrishStat
Mar 22 at 22:22
$begingroup$
You ask a lot of good questions. Most of them have been answered in more focused threads that duplicate parts of yours. But if any remain that are not answered there, please consider paring down your post to focus on those that still need replies. One keyword for a search is "Box-Cox."
$endgroup$
– whuber♦
Mar 23 at 14:06
$begingroup$
I hate it when questions such as yours are put on hold or marked as duplicate, as we cannot answer them properly. So we're reduced to having to just add comments instead of answers!
$endgroup$
– Isabella Ghement
Mar 23 at 18:02
$begingroup$
One intuitive way to think about what the log transformation is doing is to look at the distribution of Age - it's right skewed, so many of the ages are 'clumped together' at the lower end of the age scale, while fewer ages are spread out at the higher end of the age scale. When you log transform the distribution of Age, you 'stretch out' the clumped values to make it easier to see what is going on. In other words, the log transformed age values at the lower end of the scale are better separated from each other, instead of crowding each other.
$endgroup$
– Isabella Ghement
Mar 23 at 18:10
$begingroup$
Look at the high spike in the distribution of Age (a clear sign of clumping or crowdedness). That spike was attenuated in the distribution of the log-transformed Age.
$endgroup$
– Isabella Ghement
Mar 23 at 18:13
|
show 5 more comments
$begingroup$
This question already has an answer here:
In linear regression, when is it appropriate to use the log of an independent variable instead of the actual values?
8 answers
Regression: Transforming Variables
1 answer
Box-Cox like transformation for independent variables?
3 answers
When (and why) should you take the log of a distribution (of numbers)?
2 answers
I’m taking an online “Intro to AI” course for which I’m doing some azure machine learning labs. This course is largely about how to apply azure ML solutions and, while there is an “essential math for ML module”, it doesn’t do any sort of deep dive into the math/statistics. But I want to understand what’s happening mathematically to a basic level. (I've taken an intro to statistics, that's it so far.) Below are some details about some specific things I’d like to understand more, and my specific questions.
At a particular point in the “training a classification model” lab, there is this text: “The distribution of the Age column in the diabetes.csv dataset is skewed because most patients are in the youngest age bracket. Creating a version of this feature that uses a natural log transformation can help create a more linear relationship between Age and other features, and improve the ability to predict the Diabetic label. This kind of feature engineering as it’s called is common in machine learning data preparation.”
Then the instructions show you how to use Azure ML Studio to apply the natural log operation to the age column in the data set:
And after doing that, the data includes the original/raw age data and the transformed age data:
Question #1: What is that transformation actually doing? I don't mean the nitty gritty math, but what is it doing conceptually?
Question #2: The next general question is around why is a transformation necessary. On that, I did some research and found this article (https://www.r-statistics.com/2013/05/log-transformations-for-skewed-and-wide-distributions-from-practical-data-science-with-r/) that describes which type of log transformations to use in a few scenarios. Here’s a snip of text from the article:
“The need for data transformation can depend on the modeling method that you plan to use. For linear and logistic regression, for example, you ideally want to make sure that the relationship between input variables and output variables is approximately linear, that the input variables are approximately normal in distribution, and that the output variable is constant variance (that is, the variance of the output variable is independent of the input variables). You may need to transform some of your input variables to better meet these assumptions.”
I don’t understand why any of that is necessary. I’ve broken my question down:
For linear and logistic regression, for example, you ideally want to make sure that:
the relationship between input variables and output variables is approximately linear – why?
the input variables are approximately normal in distribution- why?
the output variable is constant variance (that is, the variance of the output variable is independent of the input variables – why?
Could someone help with information for those whys, or point me to material that would help?
regression linear logarithm
$endgroup$
This question already has an answer here:
In linear regression, when is it appropriate to use the log of an independent variable instead of the actual values?
8 answers
Regression: Transforming Variables
1 answer
Box-Cox like transformation for independent variables?
3 answers
When (and why) should you take the log of a distribution (of numbers)?
2 answers
I’m taking an online “Intro to AI” course for which I’m doing some azure machine learning labs. This course is largely about how to apply azure ML solutions and, while there is an “essential math for ML module”, it doesn’t do any sort of deep dive into the math/statistics. But I want to understand what’s happening mathematically to a basic level. (I've taken an intro to statistics, that's it so far.) Below are some details about some specific things I’d like to understand more, and my specific questions.
At a particular point in the “training a classification model” lab, there is this text: “The distribution of the Age column in the diabetes.csv dataset is skewed because most patients are in the youngest age bracket. Creating a version of this feature that uses a natural log transformation can help create a more linear relationship between Age and other features, and improve the ability to predict the Diabetic label. This kind of feature engineering as it’s called is common in machine learning data preparation.”
Then the instructions show you how to use Azure ML Studio to apply the natural log operation to the age column in the data set:
And after doing that, the data includes the original/raw age data and the transformed age data:
Question #1: What is that transformation actually doing? I don't mean the nitty gritty math, but what is it doing conceptually?
Question #2: The next general question is around why is a transformation necessary. On that, I did some research and found this article (https://www.r-statistics.com/2013/05/log-transformations-for-skewed-and-wide-distributions-from-practical-data-science-with-r/) that describes which type of log transformations to use in a few scenarios. Here’s a snip of text from the article:
“The need for data transformation can depend on the modeling method that you plan to use. For linear and logistic regression, for example, you ideally want to make sure that the relationship between input variables and output variables is approximately linear, that the input variables are approximately normal in distribution, and that the output variable is constant variance (that is, the variance of the output variable is independent of the input variables). You may need to transform some of your input variables to better meet these assumptions.”
I don’t understand why any of that is necessary. I’ve broken my question down:
For linear and logistic regression, for example, you ideally want to make sure that:
the relationship between input variables and output variables is approximately linear – why?
the input variables are approximately normal in distribution- why?
the output variable is constant variance (that is, the variance of the output variable is independent of the input variables – why?
Could someone help with information for those whys, or point me to material that would help?
This question already has an answer here:
In linear regression, when is it appropriate to use the log of an independent variable instead of the actual values?
8 answers
Regression: Transforming Variables
1 answer
Box-Cox like transformation for independent variables?
3 answers
When (and why) should you take the log of a distribution (of numbers)?
2 answers
regression linear logarithm
regression linear logarithm
edited Mar 22 at 23:22
Ferdi
3,86542355
3,86542355
asked Mar 22 at 22:07
redmondcoffeheadredmondcoffehead
262
262
marked as duplicate by whuber♦
StackExchange.ready(function()
if (StackExchange.options.isMobile) return;
$('.dupe-hammer-message-hover:not(.hover-bound)').each(function()
var $hover = $(this).addClass('hover-bound'),
$msg = $hover.siblings('.dupe-hammer-message');
$hover.hover(
function()
$hover.showInfoMessage('',
messageElement: $msg.clone().show(),
transient: false,
position: my: 'bottom left', at: 'top center', offsetTop: -7 ,
dismissable: false,
relativeToBody: true
);
,
function()
StackExchange.helpers.removeMessages();
);
);
);
Mar 23 at 14:05
This question has been asked before and already has an answer. If those answers do not fully address your question, please ask a new question.
marked as duplicate by whuber♦
StackExchange.ready(function()
if (StackExchange.options.isMobile) return;
$('.dupe-hammer-message-hover:not(.hover-bound)').each(function()
var $hover = $(this).addClass('hover-bound'),
$msg = $hover.siblings('.dupe-hammer-message');
$hover.hover(
function()
$hover.showInfoMessage('',
messageElement: $msg.clone().show(),
transient: false,
position: my: 'bottom left', at: 'top center', offsetTop: -7 ,
dismissable: false,
relativeToBody: true
);
,
function()
StackExchange.helpers.removeMessages();
);
);
);
Mar 23 at 14:05
This question has been asked before and already has an answer. If those answers do not fully address your question, please ask a new question.
$begingroup$
Perhaps this will help stats.stackexchange.com/questions/18844/… .
$endgroup$
– IrishStat
Mar 22 at 22:22
$begingroup$
You ask a lot of good questions. Most of them have been answered in more focused threads that duplicate parts of yours. But if any remain that are not answered there, please consider paring down your post to focus on those that still need replies. One keyword for a search is "Box-Cox."
$endgroup$
– whuber♦
Mar 23 at 14:06
$begingroup$
I hate it when questions such as yours are put on hold or marked as duplicate, as we cannot answer them properly. So we're reduced to having to just add comments instead of answers!
$endgroup$
– Isabella Ghement
Mar 23 at 18:02
$begingroup$
One intuitive way to think about what the log transformation is doing is to look at the distribution of Age - it's right skewed, so many of the ages are 'clumped together' at the lower end of the age scale, while fewer ages are spread out at the higher end of the age scale. When you log transform the distribution of Age, you 'stretch out' the clumped values to make it easier to see what is going on. In other words, the log transformed age values at the lower end of the scale are better separated from each other, instead of crowding each other.
$endgroup$
– Isabella Ghement
Mar 23 at 18:10
$begingroup$
Look at the high spike in the distribution of Age (a clear sign of clumping or crowdedness). That spike was attenuated in the distribution of the log-transformed Age.
$endgroup$
– Isabella Ghement
Mar 23 at 18:13
|
show 5 more comments
$begingroup$
Perhaps this will help stats.stackexchange.com/questions/18844/… .
$endgroup$
– IrishStat
Mar 22 at 22:22
$begingroup$
You ask a lot of good questions. Most of them have been answered in more focused threads that duplicate parts of yours. But if any remain that are not answered there, please consider paring down your post to focus on those that still need replies. One keyword for a search is "Box-Cox."
$endgroup$
– whuber♦
Mar 23 at 14:06
$begingroup$
I hate it when questions such as yours are put on hold or marked as duplicate, as we cannot answer them properly. So we're reduced to having to just add comments instead of answers!
$endgroup$
– Isabella Ghement
Mar 23 at 18:02
$begingroup$
One intuitive way to think about what the log transformation is doing is to look at the distribution of Age - it's right skewed, so many of the ages are 'clumped together' at the lower end of the age scale, while fewer ages are spread out at the higher end of the age scale. When you log transform the distribution of Age, you 'stretch out' the clumped values to make it easier to see what is going on. In other words, the log transformed age values at the lower end of the scale are better separated from each other, instead of crowding each other.
$endgroup$
– Isabella Ghement
Mar 23 at 18:10
$begingroup$
Look at the high spike in the distribution of Age (a clear sign of clumping or crowdedness). That spike was attenuated in the distribution of the log-transformed Age.
$endgroup$
– Isabella Ghement
Mar 23 at 18:13
$begingroup$
Perhaps this will help stats.stackexchange.com/questions/18844/… .
$endgroup$
– IrishStat
Mar 22 at 22:22
$begingroup$
Perhaps this will help stats.stackexchange.com/questions/18844/… .
$endgroup$
– IrishStat
Mar 22 at 22:22
$begingroup$
You ask a lot of good questions. Most of them have been answered in more focused threads that duplicate parts of yours. But if any remain that are not answered there, please consider paring down your post to focus on those that still need replies. One keyword for a search is "Box-Cox."
$endgroup$
– whuber♦
Mar 23 at 14:06
$begingroup$
You ask a lot of good questions. Most of them have been answered in more focused threads that duplicate parts of yours. But if any remain that are not answered there, please consider paring down your post to focus on those that still need replies. One keyword for a search is "Box-Cox."
$endgroup$
– whuber♦
Mar 23 at 14:06
$begingroup$
I hate it when questions such as yours are put on hold or marked as duplicate, as we cannot answer them properly. So we're reduced to having to just add comments instead of answers!
$endgroup$
– Isabella Ghement
Mar 23 at 18:02
$begingroup$
I hate it when questions such as yours are put on hold or marked as duplicate, as we cannot answer them properly. So we're reduced to having to just add comments instead of answers!
$endgroup$
– Isabella Ghement
Mar 23 at 18:02
$begingroup$
One intuitive way to think about what the log transformation is doing is to look at the distribution of Age - it's right skewed, so many of the ages are 'clumped together' at the lower end of the age scale, while fewer ages are spread out at the higher end of the age scale. When you log transform the distribution of Age, you 'stretch out' the clumped values to make it easier to see what is going on. In other words, the log transformed age values at the lower end of the scale are better separated from each other, instead of crowding each other.
$endgroup$
– Isabella Ghement
Mar 23 at 18:10
$begingroup$
One intuitive way to think about what the log transformation is doing is to look at the distribution of Age - it's right skewed, so many of the ages are 'clumped together' at the lower end of the age scale, while fewer ages are spread out at the higher end of the age scale. When you log transform the distribution of Age, you 'stretch out' the clumped values to make it easier to see what is going on. In other words, the log transformed age values at the lower end of the scale are better separated from each other, instead of crowding each other.
$endgroup$
– Isabella Ghement
Mar 23 at 18:10
$begingroup$
Look at the high spike in the distribution of Age (a clear sign of clumping or crowdedness). That spike was attenuated in the distribution of the log-transformed Age.
$endgroup$
– Isabella Ghement
Mar 23 at 18:13
$begingroup$
Look at the high spike in the distribution of Age (a clear sign of clumping or crowdedness). That spike was attenuated in the distribution of the log-transformed Age.
$endgroup$
– Isabella Ghement
Mar 23 at 18:13
|
show 5 more comments
1 Answer
1
active
oldest
votes
$begingroup$
There's a lot here to break down. I hate to say it, but some of the advice in your course is quite misguided and wrong.
What is that transformation actually doing? I don't mean the nitty gritty math, but what is it doing conceptually?
The math here is pretty simple. You have a bunch of measurements of people's age that you would like to use as a feature in predicting some other measurement (looks like the probability of something happening). You're simply creating a new feature which is the logarithm of the original feature. I'll explain why you would want to do this below.
For linear and logistic regression, for example, you ideally want to make sure that: the relationship between input variables and output variables is approximately linear – why?
This is a structural assumption of the linear and logistic regression models. I'll focus on linear regression, because its a bit simpler, but the same thing holds for logistic regression.
The linear regression model makes predictions by building a formula based on the data you feed into the algorithm. All prediction models work this way, but linear regression is distinguished by building the simplest possible formula. If $y$ is the thing you are trying to predict, and $x_1, x_2, ldots$ are the features you are using to predict it, then the linear regression formula is:
$$y = beta_0 + beta_1 x_1 + beta_2 x_2 + cdots + beta_k x_k$$
Here, the $beta_i$'s are just numbers, and the job of the algorithm is to determine what numbers work best.
Notice that if you vary one of the $x$'s, and look at how the output $y$ changes as a result, you'll get a line. This is a direct consequence of the way the linear regression model works. If you want it to give you sensible results, then you need to make sure this drawing lines assumption is at least approximately true.
the input variables are approximately normal in distribution- why?
This is simply wrong. Linear regression works fine even if the distribution of the input variables is highly non-normal. What is important is the relationship between the inputs and outputs, not the distribution of the inputs themselves.
This is what I meant by the advice the course being misguided. You don't transform input variables because their distribution is skew, you transform them so that the linear shape the model is trying to draw through your data is reasonable
For example, here is a scatterplot I found online of a country's GDP vs. its average life expectancy (attribution is in the image):
Clearly, drawing a line through the scatter plot is completely unreasonable, so the linear regression equation:
$$ textLife Expectancy = beta_0 + beta_1 textGDP $$
is a bad choice for the data. On the other hand, it looks like a logarithmic relationship is reasonable, so something like:
$$ textLife Expectancy = beta_0 + beta_1 log(textGDP) $$
looks like it would work a lot better. This is the type of situation where transforming the GDP measurements with a logarithm is a good idea. But it has nothing to do with the distribution of GDP. You can't tell it's a good idea by drawing a histogram of GDP, it's about the relationship between GDP and life expectancy.
the output variable is constant variance (that is, the variance of the output variable is independent of the input variables – why?
This is a deeper issue of a different nature than the others. For prediction models, it doesn't really matter, so if you're focusing on learning to build good predictive models don't worry about it for now.
As a summary, this assumption is intended to support the computation of the sampling distribution of parameter estimates. For example, if you want to say something like "the probability that I would collect data in which the relationship between log(GDP) and Life Expectancy is greater than what I actually observed, even when the there is truly no relationship, is very, very small" you need to be able to compute the sampling distribution of the parameter estimates. There are various assumptions that allow this to be done, and this constant variance assumption is one them.
That said, if you're only trying to make predictions, this isn't really relevant. And in no case is the distribution of the input data assumed to be normal, that's just a misconception.
$endgroup$
add a comment |
1 Answer
1
active
oldest
votes
1 Answer
1
active
oldest
votes
active
oldest
votes
active
oldest
votes
$begingroup$
There's a lot here to break down. I hate to say it, but some of the advice in your course is quite misguided and wrong.
What is that transformation actually doing? I don't mean the nitty gritty math, but what is it doing conceptually?
The math here is pretty simple. You have a bunch of measurements of people's age that you would like to use as a feature in predicting some other measurement (looks like the probability of something happening). You're simply creating a new feature which is the logarithm of the original feature. I'll explain why you would want to do this below.
For linear and logistic regression, for example, you ideally want to make sure that: the relationship between input variables and output variables is approximately linear – why?
This is a structural assumption of the linear and logistic regression models. I'll focus on linear regression, because its a bit simpler, but the same thing holds for logistic regression.
The linear regression model makes predictions by building a formula based on the data you feed into the algorithm. All prediction models work this way, but linear regression is distinguished by building the simplest possible formula. If $y$ is the thing you are trying to predict, and $x_1, x_2, ldots$ are the features you are using to predict it, then the linear regression formula is:
$$y = beta_0 + beta_1 x_1 + beta_2 x_2 + cdots + beta_k x_k$$
Here, the $beta_i$'s are just numbers, and the job of the algorithm is to determine what numbers work best.
Notice that if you vary one of the $x$'s, and look at how the output $y$ changes as a result, you'll get a line. This is a direct consequence of the way the linear regression model works. If you want it to give you sensible results, then you need to make sure this drawing lines assumption is at least approximately true.
the input variables are approximately normal in distribution- why?
This is simply wrong. Linear regression works fine even if the distribution of the input variables is highly non-normal. What is important is the relationship between the inputs and outputs, not the distribution of the inputs themselves.
This is what I meant by the advice the course being misguided. You don't transform input variables because their distribution is skew, you transform them so that the linear shape the model is trying to draw through your data is reasonable
For example, here is a scatterplot I found online of a country's GDP vs. its average life expectancy (attribution is in the image):
Clearly, drawing a line through the scatter plot is completely unreasonable, so the linear regression equation:
$$ textLife Expectancy = beta_0 + beta_1 textGDP $$
is a bad choice for the data. On the other hand, it looks like a logarithmic relationship is reasonable, so something like:
$$ textLife Expectancy = beta_0 + beta_1 log(textGDP) $$
looks like it would work a lot better. This is the type of situation where transforming the GDP measurements with a logarithm is a good idea. But it has nothing to do with the distribution of GDP. You can't tell it's a good idea by drawing a histogram of GDP, it's about the relationship between GDP and life expectancy.
the output variable is constant variance (that is, the variance of the output variable is independent of the input variables – why?
This is a deeper issue of a different nature than the others. For prediction models, it doesn't really matter, so if you're focusing on learning to build good predictive models don't worry about it for now.
As a summary, this assumption is intended to support the computation of the sampling distribution of parameter estimates. For example, if you want to say something like "the probability that I would collect data in which the relationship between log(GDP) and Life Expectancy is greater than what I actually observed, even when the there is truly no relationship, is very, very small" you need to be able to compute the sampling distribution of the parameter estimates. There are various assumptions that allow this to be done, and this constant variance assumption is one them.
That said, if you're only trying to make predictions, this isn't really relevant. And in no case is the distribution of the input data assumed to be normal, that's just a misconception.
$endgroup$
add a comment |
$begingroup$
There's a lot here to break down. I hate to say it, but some of the advice in your course is quite misguided and wrong.
What is that transformation actually doing? I don't mean the nitty gritty math, but what is it doing conceptually?
The math here is pretty simple. You have a bunch of measurements of people's age that you would like to use as a feature in predicting some other measurement (looks like the probability of something happening). You're simply creating a new feature which is the logarithm of the original feature. I'll explain why you would want to do this below.
For linear and logistic regression, for example, you ideally want to make sure that: the relationship between input variables and output variables is approximately linear – why?
This is a structural assumption of the linear and logistic regression models. I'll focus on linear regression, because its a bit simpler, but the same thing holds for logistic regression.
The linear regression model makes predictions by building a formula based on the data you feed into the algorithm. All prediction models work this way, but linear regression is distinguished by building the simplest possible formula. If $y$ is the thing you are trying to predict, and $x_1, x_2, ldots$ are the features you are using to predict it, then the linear regression formula is:
$$y = beta_0 + beta_1 x_1 + beta_2 x_2 + cdots + beta_k x_k$$
Here, the $beta_i$'s are just numbers, and the job of the algorithm is to determine what numbers work best.
Notice that if you vary one of the $x$'s, and look at how the output $y$ changes as a result, you'll get a line. This is a direct consequence of the way the linear regression model works. If you want it to give you sensible results, then you need to make sure this drawing lines assumption is at least approximately true.
the input variables are approximately normal in distribution- why?
This is simply wrong. Linear regression works fine even if the distribution of the input variables is highly non-normal. What is important is the relationship between the inputs and outputs, not the distribution of the inputs themselves.
This is what I meant by the advice the course being misguided. You don't transform input variables because their distribution is skew, you transform them so that the linear shape the model is trying to draw through your data is reasonable
For example, here is a scatterplot I found online of a country's GDP vs. its average life expectancy (attribution is in the image):
Clearly, drawing a line through the scatter plot is completely unreasonable, so the linear regression equation:
$$ textLife Expectancy = beta_0 + beta_1 textGDP $$
is a bad choice for the data. On the other hand, it looks like a logarithmic relationship is reasonable, so something like:
$$ textLife Expectancy = beta_0 + beta_1 log(textGDP) $$
looks like it would work a lot better. This is the type of situation where transforming the GDP measurements with a logarithm is a good idea. But it has nothing to do with the distribution of GDP. You can't tell it's a good idea by drawing a histogram of GDP, it's about the relationship between GDP and life expectancy.
the output variable is constant variance (that is, the variance of the output variable is independent of the input variables – why?
This is a deeper issue of a different nature than the others. For prediction models, it doesn't really matter, so if you're focusing on learning to build good predictive models don't worry about it for now.
As a summary, this assumption is intended to support the computation of the sampling distribution of parameter estimates. For example, if you want to say something like "the probability that I would collect data in which the relationship between log(GDP) and Life Expectancy is greater than what I actually observed, even when the there is truly no relationship, is very, very small" you need to be able to compute the sampling distribution of the parameter estimates. There are various assumptions that allow this to be done, and this constant variance assumption is one them.
That said, if you're only trying to make predictions, this isn't really relevant. And in no case is the distribution of the input data assumed to be normal, that's just a misconception.
$endgroup$
add a comment |
$begingroup$
There's a lot here to break down. I hate to say it, but some of the advice in your course is quite misguided and wrong.
What is that transformation actually doing? I don't mean the nitty gritty math, but what is it doing conceptually?
The math here is pretty simple. You have a bunch of measurements of people's age that you would like to use as a feature in predicting some other measurement (looks like the probability of something happening). You're simply creating a new feature which is the logarithm of the original feature. I'll explain why you would want to do this below.
For linear and logistic regression, for example, you ideally want to make sure that: the relationship between input variables and output variables is approximately linear – why?
This is a structural assumption of the linear and logistic regression models. I'll focus on linear regression, because its a bit simpler, but the same thing holds for logistic regression.
The linear regression model makes predictions by building a formula based on the data you feed into the algorithm. All prediction models work this way, but linear regression is distinguished by building the simplest possible formula. If $y$ is the thing you are trying to predict, and $x_1, x_2, ldots$ are the features you are using to predict it, then the linear regression formula is:
$$y = beta_0 + beta_1 x_1 + beta_2 x_2 + cdots + beta_k x_k$$
Here, the $beta_i$'s are just numbers, and the job of the algorithm is to determine what numbers work best.
Notice that if you vary one of the $x$'s, and look at how the output $y$ changes as a result, you'll get a line. This is a direct consequence of the way the linear regression model works. If you want it to give you sensible results, then you need to make sure this drawing lines assumption is at least approximately true.
the input variables are approximately normal in distribution- why?
This is simply wrong. Linear regression works fine even if the distribution of the input variables is highly non-normal. What is important is the relationship between the inputs and outputs, not the distribution of the inputs themselves.
This is what I meant by the advice the course being misguided. You don't transform input variables because their distribution is skew, you transform them so that the linear shape the model is trying to draw through your data is reasonable
For example, here is a scatterplot I found online of a country's GDP vs. its average life expectancy (attribution is in the image):
Clearly, drawing a line through the scatter plot is completely unreasonable, so the linear regression equation:
$$ textLife Expectancy = beta_0 + beta_1 textGDP $$
is a bad choice for the data. On the other hand, it looks like a logarithmic relationship is reasonable, so something like:
$$ textLife Expectancy = beta_0 + beta_1 log(textGDP) $$
looks like it would work a lot better. This is the type of situation where transforming the GDP measurements with a logarithm is a good idea. But it has nothing to do with the distribution of GDP. You can't tell it's a good idea by drawing a histogram of GDP, it's about the relationship between GDP and life expectancy.
the output variable is constant variance (that is, the variance of the output variable is independent of the input variables – why?
This is a deeper issue of a different nature than the others. For prediction models, it doesn't really matter, so if you're focusing on learning to build good predictive models don't worry about it for now.
As a summary, this assumption is intended to support the computation of the sampling distribution of parameter estimates. For example, if you want to say something like "the probability that I would collect data in which the relationship between log(GDP) and Life Expectancy is greater than what I actually observed, even when the there is truly no relationship, is very, very small" you need to be able to compute the sampling distribution of the parameter estimates. There are various assumptions that allow this to be done, and this constant variance assumption is one them.
That said, if you're only trying to make predictions, this isn't really relevant. And in no case is the distribution of the input data assumed to be normal, that's just a misconception.
$endgroup$
There's a lot here to break down. I hate to say it, but some of the advice in your course is quite misguided and wrong.
What is that transformation actually doing? I don't mean the nitty gritty math, but what is it doing conceptually?
The math here is pretty simple. You have a bunch of measurements of people's age that you would like to use as a feature in predicting some other measurement (looks like the probability of something happening). You're simply creating a new feature which is the logarithm of the original feature. I'll explain why you would want to do this below.
For linear and logistic regression, for example, you ideally want to make sure that: the relationship between input variables and output variables is approximately linear – why?
This is a structural assumption of the linear and logistic regression models. I'll focus on linear regression, because its a bit simpler, but the same thing holds for logistic regression.
The linear regression model makes predictions by building a formula based on the data you feed into the algorithm. All prediction models work this way, but linear regression is distinguished by building the simplest possible formula. If $y$ is the thing you are trying to predict, and $x_1, x_2, ldots$ are the features you are using to predict it, then the linear regression formula is:
$$y = beta_0 + beta_1 x_1 + beta_2 x_2 + cdots + beta_k x_k$$
Here, the $beta_i$'s are just numbers, and the job of the algorithm is to determine what numbers work best.
Notice that if you vary one of the $x$'s, and look at how the output $y$ changes as a result, you'll get a line. This is a direct consequence of the way the linear regression model works. If you want it to give you sensible results, then you need to make sure this drawing lines assumption is at least approximately true.
the input variables are approximately normal in distribution- why?
This is simply wrong. Linear regression works fine even if the distribution of the input variables is highly non-normal. What is important is the relationship between the inputs and outputs, not the distribution of the inputs themselves.
This is what I meant by the advice the course being misguided. You don't transform input variables because their distribution is skew, you transform them so that the linear shape the model is trying to draw through your data is reasonable
For example, here is a scatterplot I found online of a country's GDP vs. its average life expectancy (attribution is in the image):
Clearly, drawing a line through the scatter plot is completely unreasonable, so the linear regression equation:
$$ textLife Expectancy = beta_0 + beta_1 textGDP $$
is a bad choice for the data. On the other hand, it looks like a logarithmic relationship is reasonable, so something like:
$$ textLife Expectancy = beta_0 + beta_1 log(textGDP) $$
looks like it would work a lot better. This is the type of situation where transforming the GDP measurements with a logarithm is a good idea. But it has nothing to do with the distribution of GDP. You can't tell it's a good idea by drawing a histogram of GDP, it's about the relationship between GDP and life expectancy.
the output variable is constant variance (that is, the variance of the output variable is independent of the input variables – why?
This is a deeper issue of a different nature than the others. For prediction models, it doesn't really matter, so if you're focusing on learning to build good predictive models don't worry about it for now.
As a summary, this assumption is intended to support the computation of the sampling distribution of parameter estimates. For example, if you want to say something like "the probability that I would collect data in which the relationship between log(GDP) and Life Expectancy is greater than what I actually observed, even when the there is truly no relationship, is very, very small" you need to be able to compute the sampling distribution of the parameter estimates. There are various assumptions that allow this to be done, and this constant variance assumption is one them.
That said, if you're only trying to make predictions, this isn't really relevant. And in no case is the distribution of the input data assumed to be normal, that's just a misconception.
edited Mar 23 at 1:14
answered Mar 22 at 23:52


Matthew DruryMatthew Drury
27k267107
27k267107
add a comment |
add a comment |
aGYXNIzVvfUO,P MOEf,lM8MZ0f6HBzbkd,QMYiM j7F,Y6CleMs gvHJ qRKQ,K6EN wlwFZ5jzag3,pIc5t5ea fbKbA7,b,zcsFc2ND
$begingroup$
Perhaps this will help stats.stackexchange.com/questions/18844/… .
$endgroup$
– IrishStat
Mar 22 at 22:22
$begingroup$
You ask a lot of good questions. Most of them have been answered in more focused threads that duplicate parts of yours. But if any remain that are not answered there, please consider paring down your post to focus on those that still need replies. One keyword for a search is "Box-Cox."
$endgroup$
– whuber♦
Mar 23 at 14:06
$begingroup$
I hate it when questions such as yours are put on hold or marked as duplicate, as we cannot answer them properly. So we're reduced to having to just add comments instead of answers!
$endgroup$
– Isabella Ghement
Mar 23 at 18:02
$begingroup$
One intuitive way to think about what the log transformation is doing is to look at the distribution of Age - it's right skewed, so many of the ages are 'clumped together' at the lower end of the age scale, while fewer ages are spread out at the higher end of the age scale. When you log transform the distribution of Age, you 'stretch out' the clumped values to make it easier to see what is going on. In other words, the log transformed age values at the lower end of the scale are better separated from each other, instead of crowding each other.
$endgroup$
– Isabella Ghement
Mar 23 at 18:10
$begingroup$
Look at the high spike in the distribution of Age (a clear sign of clumping or crowdedness). That spike was attenuated in the distribution of the log-transformed Age.
$endgroup$
– Isabella Ghement
Mar 23 at 18:13