Asymptotics of the minimum of Binomial random variables The Next CEO of Stack OverflowAsymptotics of binomial coefficients and the entropy functionHow to maximize the expected number of corrected guesses?Asymptotics of a mixtureCDF of minimum of correlated and iid random variablesExtreme value distributions of uncountably infinite set of random variableslet $X_0, X_1, …$ be iid random variables with Bernoulli distribution. Suppose that $T_n = Sigma_i=0^n-1 mathbb 1_(X_i =1, X_i+1=1)$Distribution of the sum of binomial random variablesMaximum of exponential random variables is bigger than maximum of normal random variables almost surely as $n$ tends to infinity.Sum-Product of Random VariablesCentral Limit Theorem applied to binomial random variableShow that two random variables are independentExpected value of the maximum of binomial random variables
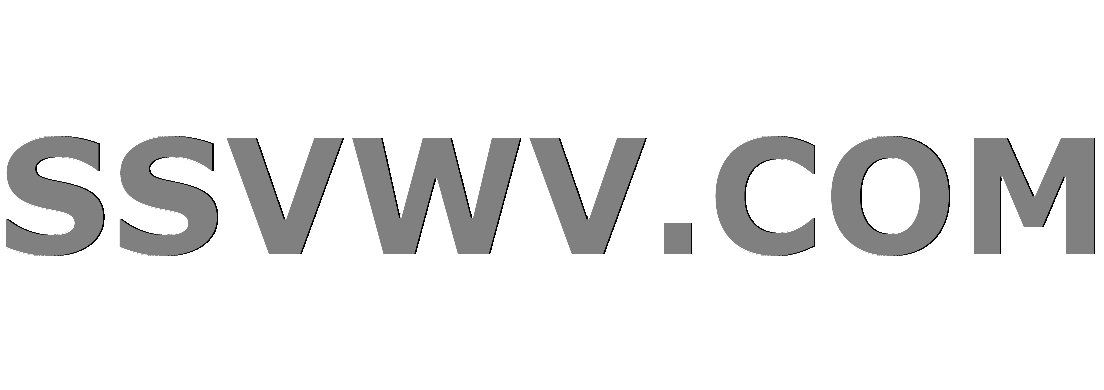
Multi tool use
Return the Closest Prime Number
What can we do to stop prior company from asking us questions?
Is the concept of a "numerable" fiber bundle really useful or an empty generalization?
How do we know the LHC results are robust?
How can I quit an app using Terminal?
Why did we only see the N-1 starfighters in one film?
Too much space between section and text in a twocolumn document
Why were Madagascar and New Zealand discovered so late?
Horror movie/show or scene where a horse creature opens its mouth really wide and devours a man in a stables
Anatomically Correct Strange Women In Ponds Distributing Swords
Can I equip Skullclamp on a creature I am sacrificing?
Why doesn't a table tennis ball float on the surface? How do we calculate buoyancy here?
What is the point of a new vote on May's deal when the indicative votes suggest she will not win?
Why didn't Theresa May consult with Parliament before negotiating a deal with the EU?
How to be diplomatic in refusing to write code that breaches the privacy of our users
Implement the Thanos sorting algorithm
What does "Its cash flow is deeply negative" mean?
I believe this to be a fraud - hired, then asked to cash check and send cash as Bitcoin
Why is there a PLL in CPU?
Can the Reverse Gravity spell affect the Meteor Swarm spell?
Example of a Mathematician/Physicist whose Other Publications during their PhD eclipsed their PhD Thesis
Can a caster that cast Polymorph on themselves stop concentrating at any point even if their Int is low?
Apart from "berlinern", do any other German dialects have a corresponding verb?
If the heap is initialized for security, then why is the stack uninitialized?
Asymptotics of the minimum of Binomial random variables
The Next CEO of Stack OverflowAsymptotics of binomial coefficients and the entropy functionHow to maximize the expected number of corrected guesses?Asymptotics of a mixtureCDF of minimum of correlated and iid random variablesExtreme value distributions of uncountably infinite set of random variableslet $X_0, X_1, …$ be iid random variables with Bernoulli distribution. Suppose that $T_n = Sigma_i=0^n-1 mathbb 1_(X_i =1, X_i+1=1)$Distribution of the sum of binomial random variablesMaximum of exponential random variables is bigger than maximum of normal random variables almost surely as $n$ tends to infinity.Sum-Product of Random VariablesCentral Limit Theorem applied to binomial random variableShow that two random variables are independentExpected value of the maximum of binomial random variables
$begingroup$
Let $Y=minX_1, X_2 cdots X_k$ be the minimum of $k$ iid Binomial $(n,1/2)$ random variables.
I'm interested in the asymptotics of $Y$ (distribution, or mean and variance) for large $n$ and $k = 2^ beta n $, with $beta < 1/2$.
Any suggestions or pointers would be appreciated.
probability asymptotics binomial-distribution extreme-value-analysis
$endgroup$
add a comment |
$begingroup$
Let $Y=minX_1, X_2 cdots X_k$ be the minimum of $k$ iid Binomial $(n,1/2)$ random variables.
I'm interested in the asymptotics of $Y$ (distribution, or mean and variance) for large $n$ and $k = 2^ beta n $, with $beta < 1/2$.
Any suggestions or pointers would be appreciated.
probability asymptotics binomial-distribution extreme-value-analysis
$endgroup$
$begingroup$
$Pr(Y=y)=left(2^-n binomni-I_frac12(n-i,i+1)+1right)^2^beta n-left(1-I_frac12(n-i,i+1)right)^2^beta n$ if $0leq y <n$ and $2^n left(-2^beta nright)$ if $y=n$ (according to Mathematica).
$endgroup$
– JimB
Mar 22 at 5:12
$begingroup$
And $I_z(a,b)$ is the regularized incomplete beta function.
$endgroup$
– JimB
Mar 22 at 5:20
$begingroup$
@JimB Perhaps you should add that as answer, perhaps commenting on its derivation.
$endgroup$
– leonbloy
Mar 22 at 15:10
add a comment |
$begingroup$
Let $Y=minX_1, X_2 cdots X_k$ be the minimum of $k$ iid Binomial $(n,1/2)$ random variables.
I'm interested in the asymptotics of $Y$ (distribution, or mean and variance) for large $n$ and $k = 2^ beta n $, with $beta < 1/2$.
Any suggestions or pointers would be appreciated.
probability asymptotics binomial-distribution extreme-value-analysis
$endgroup$
Let $Y=minX_1, X_2 cdots X_k$ be the minimum of $k$ iid Binomial $(n,1/2)$ random variables.
I'm interested in the asymptotics of $Y$ (distribution, or mean and variance) for large $n$ and $k = 2^ beta n $, with $beta < 1/2$.
Any suggestions or pointers would be appreciated.
probability asymptotics binomial-distribution extreme-value-analysis
probability asymptotics binomial-distribution extreme-value-analysis
edited Mar 21 at 23:28
leonbloy
asked Mar 17 at 21:14
leonbloyleonbloy
41.9k647108
41.9k647108
$begingroup$
$Pr(Y=y)=left(2^-n binomni-I_frac12(n-i,i+1)+1right)^2^beta n-left(1-I_frac12(n-i,i+1)right)^2^beta n$ if $0leq y <n$ and $2^n left(-2^beta nright)$ if $y=n$ (according to Mathematica).
$endgroup$
– JimB
Mar 22 at 5:12
$begingroup$
And $I_z(a,b)$ is the regularized incomplete beta function.
$endgroup$
– JimB
Mar 22 at 5:20
$begingroup$
@JimB Perhaps you should add that as answer, perhaps commenting on its derivation.
$endgroup$
– leonbloy
Mar 22 at 15:10
add a comment |
$begingroup$
$Pr(Y=y)=left(2^-n binomni-I_frac12(n-i,i+1)+1right)^2^beta n-left(1-I_frac12(n-i,i+1)right)^2^beta n$ if $0leq y <n$ and $2^n left(-2^beta nright)$ if $y=n$ (according to Mathematica).
$endgroup$
– JimB
Mar 22 at 5:12
$begingroup$
And $I_z(a,b)$ is the regularized incomplete beta function.
$endgroup$
– JimB
Mar 22 at 5:20
$begingroup$
@JimB Perhaps you should add that as answer, perhaps commenting on its derivation.
$endgroup$
– leonbloy
Mar 22 at 15:10
$begingroup$
$Pr(Y=y)=left(2^-n binomni-I_frac12(n-i,i+1)+1right)^2^beta n-left(1-I_frac12(n-i,i+1)right)^2^beta n$ if $0leq y <n$ and $2^n left(-2^beta nright)$ if $y=n$ (according to Mathematica).
$endgroup$
– JimB
Mar 22 at 5:12
$begingroup$
$Pr(Y=y)=left(2^-n binomni-I_frac12(n-i,i+1)+1right)^2^beta n-left(1-I_frac12(n-i,i+1)right)^2^beta n$ if $0leq y <n$ and $2^n left(-2^beta nright)$ if $y=n$ (according to Mathematica).
$endgroup$
– JimB
Mar 22 at 5:12
$begingroup$
And $I_z(a,b)$ is the regularized incomplete beta function.
$endgroup$
– JimB
Mar 22 at 5:20
$begingroup$
And $I_z(a,b)$ is the regularized incomplete beta function.
$endgroup$
– JimB
Mar 22 at 5:20
$begingroup$
@JimB Perhaps you should add that as answer, perhaps commenting on its derivation.
$endgroup$
– leonbloy
Mar 22 at 15:10
$begingroup$
@JimB Perhaps you should add that as answer, perhaps commenting on its derivation.
$endgroup$
– leonbloy
Mar 22 at 15:10
add a comment |
2 Answers
2
active
oldest
votes
$begingroup$
This is just a partial answer in that it gives the probability mass function for finite values of $n$ (i.e., no asymptotics).
Using Mathematica one can find the probability mass function for a specific $n$ and $beta$ with the following commands:
distY = OrderDistribution[BinomialDistribution[n, 1/2], 2^(beta n), 1];
pmf = PDF[distY, y]
with the following result:
$$Pr(Y=y)=left(2^-n binomny-I_frac12(n-y,y+1)+1right)^2^beta n-left(1-I_frac12(n-y,y+1)right)^2^beta n$$
when $0leq y<n$ and
$$Pr(Y=n)=left(2^-n binomnyright)^2^beta n$$
when $y=n$ where $I_z (a,b)$ is the regularized incomplete beta function.
If $beta=1/20$, then one can construct a table of the means and variances for values of $n$:
beta = 1/20;
distY = OrderDistribution[BinomialDistribution[n, 1/2], 2^(beta n), 1];
data = Table[n, 2^(beta n), Mean[distY] // N, Variance[distY] // N, n, 20, 240, 20]
TableForm[data, TableHeadings -> None, "n", "k", "Mean", "Variance"]
$$
beginarraycccc
20 & 2 & 8.74629 & 3.42822 \
40 & 4 & 16.7558 & 4.91162 \
60 & 8 & 24.5034 & 5.56277 \
80 & 16 & 32.1274 & 5.85087 \
100 & 32 & 39.6867 & 5.97801 \
120 & 64 & 47.2088 & 6.03288 \
140 & 128 & 54.7079 & 6.05502 \
160 & 256 & 62.1913 & 6.06233 \
180 & 512 & 69.6636 & 6.06297 \
200 & 1024 & 77.1272 & 6.06068 \
220 & 2048 & 84.5839 & 6.05716 \
240 & 4096 & 92.0349 & 6.05319 \
endarray
$$
With larger values of $n$, there will likely be numerical overflow issues unless some care is taken. However, so far the relationship with $n$ and the mean seems pretty linear:
$endgroup$
add a comment |
$begingroup$
This attempts to get the asymptotical behaviour of $P(Y)$, making some adaptations (and, I think, some simplifications and improvements) to this answer in other SE site, which corresponds to essentially this problem with $beta < 1/2$.
We seek to find some $d$ such that $P(X_ile d) approx 1/k$, so that $P(Y>d) approx (1-1/k)^k to e^-1$.
We expect (hope) that $d/n$ will be asymptotically constant, as $ntoinfty$.
Let's use the approximation $log_2 binomnd approx n , h(d/n)$, where $h()$ is the binary entropy function, so
$$P(X_i=d)approx 2^-n(1-h(d/n)) tag1$$
Noticing that for $delta ll x$ we have $h(x+delta) approx h(x) - delta log_2(fracx1-x)$, or
$$hleft(fracd-jnright)approx h(d/n) + log_2left(fracdn-dright) fracjn tag2$$
then
$$P(X_i=d-j)approx P(X_i=d) left(fracdn-dright)^j tag3$$
and
$$P(X_i le d) = sum_j=0^d P(X_i=d-j) approx P(X_i=d) left(fracn-dn-2dright) tag 4$$
Plugging $(1)$ into $(4)$ and equating it to $1/k= 2^-beta n$, calling $t=d/n$, we get
$$ log_2left(frac1- 2 t1-tright)=n left(h(t) - 1 +beta right) tag5$$
If we impose that $t$ is (asympotically) constant wrt $n$, then this implies that (asympotically) this constant (which we call $hatt$) must be a root of
$$ h(t)=1-beta tag6$$
In this case, it should be the lower ($t<1/2$) root. For example, for $beta=1/4$ we
get $hatt=0.2145017$.
So we take $d = n hatt $ , rounded to the nearest integer.
This implies (details here) that the distribution of $Y$ corresponds to a Gumbel distribution, with mean tending asymptotically to $d$ (hence growing linearly with $n$) and constant variance. Hence $Y$ is asymptotically concentrated around the root of $(6)$.
$endgroup$
add a comment |
Your Answer
StackExchange.ifUsing("editor", function ()
return StackExchange.using("mathjaxEditing", function ()
StackExchange.MarkdownEditor.creationCallbacks.add(function (editor, postfix)
StackExchange.mathjaxEditing.prepareWmdForMathJax(editor, postfix, [["$", "$"], ["\\(","\\)"]]);
);
);
, "mathjax-editing");
StackExchange.ready(function()
var channelOptions =
tags: "".split(" "),
id: "69"
;
initTagRenderer("".split(" "), "".split(" "), channelOptions);
StackExchange.using("externalEditor", function()
// Have to fire editor after snippets, if snippets enabled
if (StackExchange.settings.snippets.snippetsEnabled)
StackExchange.using("snippets", function()
createEditor();
);
else
createEditor();
);
function createEditor()
StackExchange.prepareEditor(
heartbeatType: 'answer',
autoActivateHeartbeat: false,
convertImagesToLinks: true,
noModals: true,
showLowRepImageUploadWarning: true,
reputationToPostImages: 10,
bindNavPrevention: true,
postfix: "",
imageUploader:
brandingHtml: "Powered by u003ca class="icon-imgur-white" href="https://imgur.com/"u003eu003c/au003e",
contentPolicyHtml: "User contributions licensed under u003ca href="https://creativecommons.org/licenses/by-sa/3.0/"u003ecc by-sa 3.0 with attribution requiredu003c/au003e u003ca href="https://stackoverflow.com/legal/content-policy"u003e(content policy)u003c/au003e",
allowUrls: true
,
noCode: true, onDemand: true,
discardSelector: ".discard-answer"
,immediatelyShowMarkdownHelp:true
);
);
Sign up or log in
StackExchange.ready(function ()
StackExchange.helpers.onClickDraftSave('#login-link');
);
Sign up using Google
Sign up using Facebook
Sign up using Email and Password
Post as a guest
Required, but never shown
StackExchange.ready(
function ()
StackExchange.openid.initPostLogin('.new-post-login', 'https%3a%2f%2fmath.stackexchange.com%2fquestions%2f3152094%2fasymptotics-of-the-minimum-of-binomial-random-variables%23new-answer', 'question_page');
);
Post as a guest
Required, but never shown
2 Answers
2
active
oldest
votes
2 Answers
2
active
oldest
votes
active
oldest
votes
active
oldest
votes
$begingroup$
This is just a partial answer in that it gives the probability mass function for finite values of $n$ (i.e., no asymptotics).
Using Mathematica one can find the probability mass function for a specific $n$ and $beta$ with the following commands:
distY = OrderDistribution[BinomialDistribution[n, 1/2], 2^(beta n), 1];
pmf = PDF[distY, y]
with the following result:
$$Pr(Y=y)=left(2^-n binomny-I_frac12(n-y,y+1)+1right)^2^beta n-left(1-I_frac12(n-y,y+1)right)^2^beta n$$
when $0leq y<n$ and
$$Pr(Y=n)=left(2^-n binomnyright)^2^beta n$$
when $y=n$ where $I_z (a,b)$ is the regularized incomplete beta function.
If $beta=1/20$, then one can construct a table of the means and variances for values of $n$:
beta = 1/20;
distY = OrderDistribution[BinomialDistribution[n, 1/2], 2^(beta n), 1];
data = Table[n, 2^(beta n), Mean[distY] // N, Variance[distY] // N, n, 20, 240, 20]
TableForm[data, TableHeadings -> None, "n", "k", "Mean", "Variance"]
$$
beginarraycccc
20 & 2 & 8.74629 & 3.42822 \
40 & 4 & 16.7558 & 4.91162 \
60 & 8 & 24.5034 & 5.56277 \
80 & 16 & 32.1274 & 5.85087 \
100 & 32 & 39.6867 & 5.97801 \
120 & 64 & 47.2088 & 6.03288 \
140 & 128 & 54.7079 & 6.05502 \
160 & 256 & 62.1913 & 6.06233 \
180 & 512 & 69.6636 & 6.06297 \
200 & 1024 & 77.1272 & 6.06068 \
220 & 2048 & 84.5839 & 6.05716 \
240 & 4096 & 92.0349 & 6.05319 \
endarray
$$
With larger values of $n$, there will likely be numerical overflow issues unless some care is taken. However, so far the relationship with $n$ and the mean seems pretty linear:
$endgroup$
add a comment |
$begingroup$
This is just a partial answer in that it gives the probability mass function for finite values of $n$ (i.e., no asymptotics).
Using Mathematica one can find the probability mass function for a specific $n$ and $beta$ with the following commands:
distY = OrderDistribution[BinomialDistribution[n, 1/2], 2^(beta n), 1];
pmf = PDF[distY, y]
with the following result:
$$Pr(Y=y)=left(2^-n binomny-I_frac12(n-y,y+1)+1right)^2^beta n-left(1-I_frac12(n-y,y+1)right)^2^beta n$$
when $0leq y<n$ and
$$Pr(Y=n)=left(2^-n binomnyright)^2^beta n$$
when $y=n$ where $I_z (a,b)$ is the regularized incomplete beta function.
If $beta=1/20$, then one can construct a table of the means and variances for values of $n$:
beta = 1/20;
distY = OrderDistribution[BinomialDistribution[n, 1/2], 2^(beta n), 1];
data = Table[n, 2^(beta n), Mean[distY] // N, Variance[distY] // N, n, 20, 240, 20]
TableForm[data, TableHeadings -> None, "n", "k", "Mean", "Variance"]
$$
beginarraycccc
20 & 2 & 8.74629 & 3.42822 \
40 & 4 & 16.7558 & 4.91162 \
60 & 8 & 24.5034 & 5.56277 \
80 & 16 & 32.1274 & 5.85087 \
100 & 32 & 39.6867 & 5.97801 \
120 & 64 & 47.2088 & 6.03288 \
140 & 128 & 54.7079 & 6.05502 \
160 & 256 & 62.1913 & 6.06233 \
180 & 512 & 69.6636 & 6.06297 \
200 & 1024 & 77.1272 & 6.06068 \
220 & 2048 & 84.5839 & 6.05716 \
240 & 4096 & 92.0349 & 6.05319 \
endarray
$$
With larger values of $n$, there will likely be numerical overflow issues unless some care is taken. However, so far the relationship with $n$ and the mean seems pretty linear:
$endgroup$
add a comment |
$begingroup$
This is just a partial answer in that it gives the probability mass function for finite values of $n$ (i.e., no asymptotics).
Using Mathematica one can find the probability mass function for a specific $n$ and $beta$ with the following commands:
distY = OrderDistribution[BinomialDistribution[n, 1/2], 2^(beta n), 1];
pmf = PDF[distY, y]
with the following result:
$$Pr(Y=y)=left(2^-n binomny-I_frac12(n-y,y+1)+1right)^2^beta n-left(1-I_frac12(n-y,y+1)right)^2^beta n$$
when $0leq y<n$ and
$$Pr(Y=n)=left(2^-n binomnyright)^2^beta n$$
when $y=n$ where $I_z (a,b)$ is the regularized incomplete beta function.
If $beta=1/20$, then one can construct a table of the means and variances for values of $n$:
beta = 1/20;
distY = OrderDistribution[BinomialDistribution[n, 1/2], 2^(beta n), 1];
data = Table[n, 2^(beta n), Mean[distY] // N, Variance[distY] // N, n, 20, 240, 20]
TableForm[data, TableHeadings -> None, "n", "k", "Mean", "Variance"]
$$
beginarraycccc
20 & 2 & 8.74629 & 3.42822 \
40 & 4 & 16.7558 & 4.91162 \
60 & 8 & 24.5034 & 5.56277 \
80 & 16 & 32.1274 & 5.85087 \
100 & 32 & 39.6867 & 5.97801 \
120 & 64 & 47.2088 & 6.03288 \
140 & 128 & 54.7079 & 6.05502 \
160 & 256 & 62.1913 & 6.06233 \
180 & 512 & 69.6636 & 6.06297 \
200 & 1024 & 77.1272 & 6.06068 \
220 & 2048 & 84.5839 & 6.05716 \
240 & 4096 & 92.0349 & 6.05319 \
endarray
$$
With larger values of $n$, there will likely be numerical overflow issues unless some care is taken. However, so far the relationship with $n$ and the mean seems pretty linear:
$endgroup$
This is just a partial answer in that it gives the probability mass function for finite values of $n$ (i.e., no asymptotics).
Using Mathematica one can find the probability mass function for a specific $n$ and $beta$ with the following commands:
distY = OrderDistribution[BinomialDistribution[n, 1/2], 2^(beta n), 1];
pmf = PDF[distY, y]
with the following result:
$$Pr(Y=y)=left(2^-n binomny-I_frac12(n-y,y+1)+1right)^2^beta n-left(1-I_frac12(n-y,y+1)right)^2^beta n$$
when $0leq y<n$ and
$$Pr(Y=n)=left(2^-n binomnyright)^2^beta n$$
when $y=n$ where $I_z (a,b)$ is the regularized incomplete beta function.
If $beta=1/20$, then one can construct a table of the means and variances for values of $n$:
beta = 1/20;
distY = OrderDistribution[BinomialDistribution[n, 1/2], 2^(beta n), 1];
data = Table[n, 2^(beta n), Mean[distY] // N, Variance[distY] // N, n, 20, 240, 20]
TableForm[data, TableHeadings -> None, "n", "k", "Mean", "Variance"]
$$
beginarraycccc
20 & 2 & 8.74629 & 3.42822 \
40 & 4 & 16.7558 & 4.91162 \
60 & 8 & 24.5034 & 5.56277 \
80 & 16 & 32.1274 & 5.85087 \
100 & 32 & 39.6867 & 5.97801 \
120 & 64 & 47.2088 & 6.03288 \
140 & 128 & 54.7079 & 6.05502 \
160 & 256 & 62.1913 & 6.06233 \
180 & 512 & 69.6636 & 6.06297 \
200 & 1024 & 77.1272 & 6.06068 \
220 & 2048 & 84.5839 & 6.05716 \
240 & 4096 & 92.0349 & 6.05319 \
endarray
$$
With larger values of $n$, there will likely be numerical overflow issues unless some care is taken. However, so far the relationship with $n$ and the mean seems pretty linear:
edited Mar 22 at 22:48
answered Mar 22 at 21:52
JimBJimB
61547
61547
add a comment |
add a comment |
$begingroup$
This attempts to get the asymptotical behaviour of $P(Y)$, making some adaptations (and, I think, some simplifications and improvements) to this answer in other SE site, which corresponds to essentially this problem with $beta < 1/2$.
We seek to find some $d$ such that $P(X_ile d) approx 1/k$, so that $P(Y>d) approx (1-1/k)^k to e^-1$.
We expect (hope) that $d/n$ will be asymptotically constant, as $ntoinfty$.
Let's use the approximation $log_2 binomnd approx n , h(d/n)$, where $h()$ is the binary entropy function, so
$$P(X_i=d)approx 2^-n(1-h(d/n)) tag1$$
Noticing that for $delta ll x$ we have $h(x+delta) approx h(x) - delta log_2(fracx1-x)$, or
$$hleft(fracd-jnright)approx h(d/n) + log_2left(fracdn-dright) fracjn tag2$$
then
$$P(X_i=d-j)approx P(X_i=d) left(fracdn-dright)^j tag3$$
and
$$P(X_i le d) = sum_j=0^d P(X_i=d-j) approx P(X_i=d) left(fracn-dn-2dright) tag 4$$
Plugging $(1)$ into $(4)$ and equating it to $1/k= 2^-beta n$, calling $t=d/n$, we get
$$ log_2left(frac1- 2 t1-tright)=n left(h(t) - 1 +beta right) tag5$$
If we impose that $t$ is (asympotically) constant wrt $n$, then this implies that (asympotically) this constant (which we call $hatt$) must be a root of
$$ h(t)=1-beta tag6$$
In this case, it should be the lower ($t<1/2$) root. For example, for $beta=1/4$ we
get $hatt=0.2145017$.
So we take $d = n hatt $ , rounded to the nearest integer.
This implies (details here) that the distribution of $Y$ corresponds to a Gumbel distribution, with mean tending asymptotically to $d$ (hence growing linearly with $n$) and constant variance. Hence $Y$ is asymptotically concentrated around the root of $(6)$.
$endgroup$
add a comment |
$begingroup$
This attempts to get the asymptotical behaviour of $P(Y)$, making some adaptations (and, I think, some simplifications and improvements) to this answer in other SE site, which corresponds to essentially this problem with $beta < 1/2$.
We seek to find some $d$ such that $P(X_ile d) approx 1/k$, so that $P(Y>d) approx (1-1/k)^k to e^-1$.
We expect (hope) that $d/n$ will be asymptotically constant, as $ntoinfty$.
Let's use the approximation $log_2 binomnd approx n , h(d/n)$, where $h()$ is the binary entropy function, so
$$P(X_i=d)approx 2^-n(1-h(d/n)) tag1$$
Noticing that for $delta ll x$ we have $h(x+delta) approx h(x) - delta log_2(fracx1-x)$, or
$$hleft(fracd-jnright)approx h(d/n) + log_2left(fracdn-dright) fracjn tag2$$
then
$$P(X_i=d-j)approx P(X_i=d) left(fracdn-dright)^j tag3$$
and
$$P(X_i le d) = sum_j=0^d P(X_i=d-j) approx P(X_i=d) left(fracn-dn-2dright) tag 4$$
Plugging $(1)$ into $(4)$ and equating it to $1/k= 2^-beta n$, calling $t=d/n$, we get
$$ log_2left(frac1- 2 t1-tright)=n left(h(t) - 1 +beta right) tag5$$
If we impose that $t$ is (asympotically) constant wrt $n$, then this implies that (asympotically) this constant (which we call $hatt$) must be a root of
$$ h(t)=1-beta tag6$$
In this case, it should be the lower ($t<1/2$) root. For example, for $beta=1/4$ we
get $hatt=0.2145017$.
So we take $d = n hatt $ , rounded to the nearest integer.
This implies (details here) that the distribution of $Y$ corresponds to a Gumbel distribution, with mean tending asymptotically to $d$ (hence growing linearly with $n$) and constant variance. Hence $Y$ is asymptotically concentrated around the root of $(6)$.
$endgroup$
add a comment |
$begingroup$
This attempts to get the asymptotical behaviour of $P(Y)$, making some adaptations (and, I think, some simplifications and improvements) to this answer in other SE site, which corresponds to essentially this problem with $beta < 1/2$.
We seek to find some $d$ such that $P(X_ile d) approx 1/k$, so that $P(Y>d) approx (1-1/k)^k to e^-1$.
We expect (hope) that $d/n$ will be asymptotically constant, as $ntoinfty$.
Let's use the approximation $log_2 binomnd approx n , h(d/n)$, where $h()$ is the binary entropy function, so
$$P(X_i=d)approx 2^-n(1-h(d/n)) tag1$$
Noticing that for $delta ll x$ we have $h(x+delta) approx h(x) - delta log_2(fracx1-x)$, or
$$hleft(fracd-jnright)approx h(d/n) + log_2left(fracdn-dright) fracjn tag2$$
then
$$P(X_i=d-j)approx P(X_i=d) left(fracdn-dright)^j tag3$$
and
$$P(X_i le d) = sum_j=0^d P(X_i=d-j) approx P(X_i=d) left(fracn-dn-2dright) tag 4$$
Plugging $(1)$ into $(4)$ and equating it to $1/k= 2^-beta n$, calling $t=d/n$, we get
$$ log_2left(frac1- 2 t1-tright)=n left(h(t) - 1 +beta right) tag5$$
If we impose that $t$ is (asympotically) constant wrt $n$, then this implies that (asympotically) this constant (which we call $hatt$) must be a root of
$$ h(t)=1-beta tag6$$
In this case, it should be the lower ($t<1/2$) root. For example, for $beta=1/4$ we
get $hatt=0.2145017$.
So we take $d = n hatt $ , rounded to the nearest integer.
This implies (details here) that the distribution of $Y$ corresponds to a Gumbel distribution, with mean tending asymptotically to $d$ (hence growing linearly with $n$) and constant variance. Hence $Y$ is asymptotically concentrated around the root of $(6)$.
$endgroup$
This attempts to get the asymptotical behaviour of $P(Y)$, making some adaptations (and, I think, some simplifications and improvements) to this answer in other SE site, which corresponds to essentially this problem with $beta < 1/2$.
We seek to find some $d$ such that $P(X_ile d) approx 1/k$, so that $P(Y>d) approx (1-1/k)^k to e^-1$.
We expect (hope) that $d/n$ will be asymptotically constant, as $ntoinfty$.
Let's use the approximation $log_2 binomnd approx n , h(d/n)$, where $h()$ is the binary entropy function, so
$$P(X_i=d)approx 2^-n(1-h(d/n)) tag1$$
Noticing that for $delta ll x$ we have $h(x+delta) approx h(x) - delta log_2(fracx1-x)$, or
$$hleft(fracd-jnright)approx h(d/n) + log_2left(fracdn-dright) fracjn tag2$$
then
$$P(X_i=d-j)approx P(X_i=d) left(fracdn-dright)^j tag3$$
and
$$P(X_i le d) = sum_j=0^d P(X_i=d-j) approx P(X_i=d) left(fracn-dn-2dright) tag 4$$
Plugging $(1)$ into $(4)$ and equating it to $1/k= 2^-beta n$, calling $t=d/n$, we get
$$ log_2left(frac1- 2 t1-tright)=n left(h(t) - 1 +beta right) tag5$$
If we impose that $t$ is (asympotically) constant wrt $n$, then this implies that (asympotically) this constant (which we call $hatt$) must be a root of
$$ h(t)=1-beta tag6$$
In this case, it should be the lower ($t<1/2$) root. For example, for $beta=1/4$ we
get $hatt=0.2145017$.
So we take $d = n hatt $ , rounded to the nearest integer.
This implies (details here) that the distribution of $Y$ corresponds to a Gumbel distribution, with mean tending asymptotically to $d$ (hence growing linearly with $n$) and constant variance. Hence $Y$ is asymptotically concentrated around the root of $(6)$.
edited Mar 23 at 14:58
answered Mar 23 at 2:09
leonbloyleonbloy
41.9k647108
41.9k647108
add a comment |
add a comment |
Thanks for contributing an answer to Mathematics Stack Exchange!
- Please be sure to answer the question. Provide details and share your research!
But avoid …
- Asking for help, clarification, or responding to other answers.
- Making statements based on opinion; back them up with references or personal experience.
Use MathJax to format equations. MathJax reference.
To learn more, see our tips on writing great answers.
Sign up or log in
StackExchange.ready(function ()
StackExchange.helpers.onClickDraftSave('#login-link');
);
Sign up using Google
Sign up using Facebook
Sign up using Email and Password
Post as a guest
Required, but never shown
StackExchange.ready(
function ()
StackExchange.openid.initPostLogin('.new-post-login', 'https%3a%2f%2fmath.stackexchange.com%2fquestions%2f3152094%2fasymptotics-of-the-minimum-of-binomial-random-variables%23new-answer', 'question_page');
);
Post as a guest
Required, but never shown
Sign up or log in
StackExchange.ready(function ()
StackExchange.helpers.onClickDraftSave('#login-link');
);
Sign up using Google
Sign up using Facebook
Sign up using Email and Password
Post as a guest
Required, but never shown
Sign up or log in
StackExchange.ready(function ()
StackExchange.helpers.onClickDraftSave('#login-link');
);
Sign up using Google
Sign up using Facebook
Sign up using Email and Password
Post as a guest
Required, but never shown
Sign up or log in
StackExchange.ready(function ()
StackExchange.helpers.onClickDraftSave('#login-link');
);
Sign up using Google
Sign up using Facebook
Sign up using Email and Password
Sign up using Google
Sign up using Facebook
Sign up using Email and Password
Post as a guest
Required, but never shown
Required, but never shown
Required, but never shown
Required, but never shown
Required, but never shown
Required, but never shown
Required, but never shown
Required, but never shown
Required, but never shown
aH l wXlR30qe,L8ObiPLYhMw0VWI y7NE8,M3SIUfP,TAm1kW6K7yUfTQ5wx 2bHaol
$begingroup$
$Pr(Y=y)=left(2^-n binomni-I_frac12(n-i,i+1)+1right)^2^beta n-left(1-I_frac12(n-i,i+1)right)^2^beta n$ if $0leq y <n$ and $2^n left(-2^beta nright)$ if $y=n$ (according to Mathematica).
$endgroup$
– JimB
Mar 22 at 5:12
$begingroup$
And $I_z(a,b)$ is the regularized incomplete beta function.
$endgroup$
– JimB
Mar 22 at 5:20
$begingroup$
@JimB Perhaps you should add that as answer, perhaps commenting on its derivation.
$endgroup$
– leonbloy
Mar 22 at 15:10